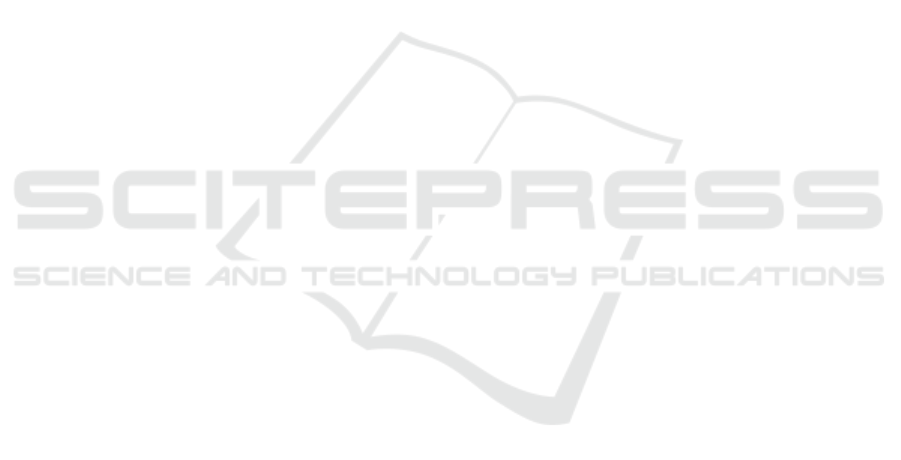
overhead, compared to more complex machine learn-
ing models, makes it an attractive option for a wide
range of applications. Importantly, the effectiveness
of the AnEWMA in our tests suggests its potential as
a standalone tool in scenarios where the prompt and
accurate detection of anomalies is essential, negating
the necessity for extensive historical data and reduc-
ing the time to implementation.
The contributions of this work are summarized as
follows:
1. Effective Anomaly Detection: AnEWMA demon-
strates adequate sensitivity and specificity in de-
tecting deviations from established patterns.
2. Simplicity and Efficiency: Its simplicity and low
computational overhead makes it a practical alter-
native to more complex machine learning models.
3. Standalone Tool Potential: The algorithm’s effec-
tiveness suggests it can be used as a standalone
tool, especially in scenarios requiring prompt and
accurate anomaly detection, without the need for
extensive historical data, thus reducing implemen-
tation time.
This paper is organized as follows: Section 2
briefly covers major research work in anomaly detec-
tion in time series. Section 3 reviews the Exponen-
tially Weighted Moving Average method. Sections 4
and 5 describe in detail the methodology and Section
6 shows the detection algorithm, AnEWMA. Section
7 presents the simulation implementation, assump-
tions, and performance results. Section 8 presents the
conclusion and future work.
2 BACKGROUND
Adams et al. (Adams and MacKay, 2007) presents
a Bayesian online changepoint detection is a method
used for identifying changes in the statistical proper-
ties of a time series in real-time. A changepoint is
defined as a time when the statistical properties of the
data abruptly shift. The algorithm detects the most
recent changepoint in the current input values by ana-
lyzing the probability distributions of time series par-
titions, which are derived from changepoints identi-
fied in past values. However, the algorithm requires
a well-specified probabilistic model for the data. If
the model is poorly chosen or does not fit the data ac-
curately, the changepoint detection performance will
degrade significantly.
The authors in (Maciag et al., 2019) introduce a
novel approach called Online Evolving Spiking Neu-
ral Networks for Unsupervised Anomaly Detection
(OeSNN-UAD). The article shows that the spiking
neural networks evolve over time, adapting to new
data without the need for retraining and incorporates
dynamic normalization to handle variations in the
data stream. But the evolving nature of the network
and the use of spiking neurons can make the imple-
mentation complex and computationally demanding.
D
¨
aubener et al. (D
¨
aubener et al., 2019) conducted
an empirical comparison of common machine learn-
ing and statistical methods for anomaly detection.
Their findings indicate that Gaussian processes and
support vector machines perform slightly better than
other algorithms.
Garcia et al. (Bl
´
azquez-Garc
´
ıa et al., 2021) pre-
sented a structured and comprehensive state-of-the-
art on outlier detection techniques in time series data.
The authors propose a taxonomy based on three main
aspects: the type of input data, the type of out-
lier, and the nature of the detection method. The
paper discusses various types of outliers, including
point anomalies, contextual anomalies, and collective
anomalies It also covers a wide range of detection
methods, such as statistical methods, machine learn-
ing approaches, and hybrid techniques.
The article (Laptev et al., 2015) introduces a
framework developed by Yahoo Labs for detecting
anomalies in large-scale time-series data. The frame-
work aims to provide early detection of anomalies
to maintain data consistency and protect against ma-
licious attacks. The framework uses a combination
of anomaly detection and forecasting models with an
anomaly filtering layer to improve accuracy and scal-
ability. The framework shows a 50-60% improvement
in precision and recall across various use cases.
Thill et al. (Thill et al., 2017) proposed an on-
line regression anomaly detector (SORAD) for de-
tecting anomalies in streaming data using the Yahoo
S5 dataset (Webscope, 2015). The authors show that
SORAD outperformed other detection algorithms on
these datasets. However, the algorithm doesn’t work
well on the Numenta Anomaly Benchmark (NAB)
datasets.
Schneider et al. (Schneider et al., 2016) intro-
duces an algorithm to detect anomalies by estimating
the similarity between new data points and the dis-
tribution of regular data. EXPoSE is a kernel-based
method that uses an inner product with a reproduc-
ing kernel Hilbert space embedding, making no as-
sumptions about the data distribution. It offers linear
time complexity for batch learning and constant time
for online learning and predictions. However, the per-
formance of EXPoSE heavily depends on the choice
of the kernel function, which may require domain-
specific knowledge and experimentation.
Etsy Skyline (Stanway, 2013) is an open-source
Anomaly Detection on Univariate Time Series Data Using Exponentially Weighted Moving Average (AnEWMA)
403