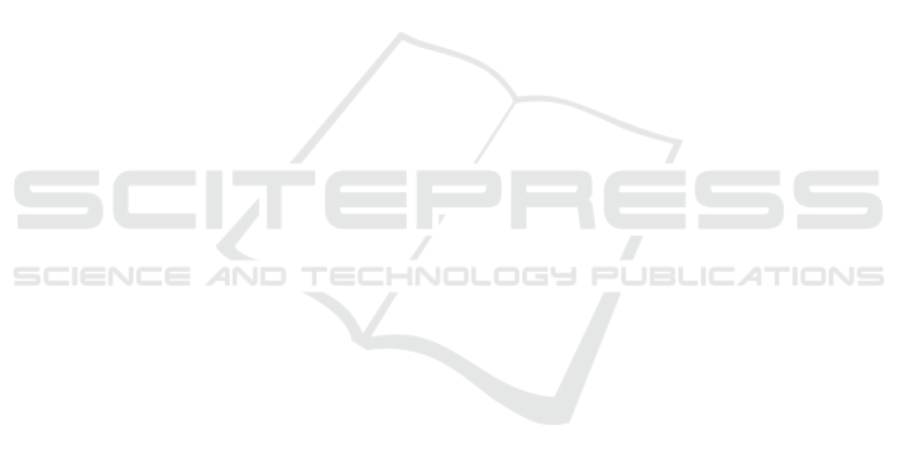
Test. However, it faced challenges with certain
blurred images and patients wearing corrective lenses.
These issues are expected, as the CNN identifies the
most likely locations for the CR point, and the fil-
tering process may not always correct inaccurate de-
tections. Additionally, there were difficulties related
to horizontally or vertically occluded limbus, which
are common error cases. The CNN struggles to ac-
curately estimate the location and size of the limbus
bounding box when it lacks relevant information in
the image. Nevertheless, the method is expected to
yield lower error rates for these situations. Such limi-
tations could be addressed by utilizing a larger dataset
and training for more epochs.
A possible use case for this method involves cap-
turing patients’ photos with a camera’s flash from dif-
ferent positions and then analyzing the images with
the model for preliminary strabismus measurement.
If the detection exceeds a threshold, the model could
prompt the patient to see an expert for in-person mea-
surements. This technique could help prevent many
cases of early strabismus, amblyopia, and other vi-
sion problems related to strabismus by providing oph-
thalmologists with a preliminary screening tool that
doesn’t require specialized equipment.
In future work, the bounding box filtering could
be augmented since it is a heuristic that does not al-
ways produce precise results. This is evident from
some detections of CR that are very distant (Euclidean
distance > 200px) in the image. A possible aug-
mentation for the bounding box filtering would be to
somehow segment the patient’s face and only consider
bounding boxes within that area.
Additionally, the training process could be opti-
mized by training for more epochs, fine-tuning hy-
perparameters (Tuba et al., 2021), or using a larger
dataset, which could enhance the CNN’s generaliza-
tion and object detection performance. Moreover,
case studies similar to (Cheng et al., 2021) could
be undertaken to evaluate the method’s effectiveness
and performance in clinics, particularly in challeng-
ing cases with real personnel.
ACKNOWLEDGEMENTS
The authors acknowledge the Coordenac¸
˜
ao de
Aperfeic¸oamento de Pessoal de N
´
ıvel Superior
(CAPES), Brazil - Finance Code 001, Conselho
Nacional de Desenvolvimento Cient
´
ıfico e Tec-
nol
´
ogico (CNPq), Brazil, and Fundac¸
˜
ao de Am-
paro
`
a Pesquisa Desenvolvimento Cient
´
ıfico e Tec-
nol
´
ogico do Maranh
˜
ao (FAPEMA) (Brazil), Empresa
Brasileira de Servic¸os Hospitalares (Ebserh) Brazil
(Grant number 409593/2021-4) for the financial sup-
port.
REFERENCES
Almeida, J.D. S., S. A. T. J. (2015). Computer-aided
methodology for syndromic strabismus diagnosis.
Journal of Digital Imaging, 28:462—-473.
Bazarevsky, V., Kartynnik, Y., Vakunov, A., Raveendran,
K., and Grundmann, M. (2019). Blazeface: Sub-
millisecond neural face detection on mobile gpus.
Buffenn, A. N. (2021). The impact of strabismus on psy-
chosocial heath and quality of life: a systematic re-
view. Survey of Ophthalmology, 66(6):1051–1064.
Canny, J. (1986). A computational approach to edge de-
tection. IEEE Transactions on pattern analysis and
machine intelligence, (6):679–698.
Cheng, W., Lynn, M. H., Pundlik, S., Almeida, C., Luo, G.,
and Houston, K. (2021). A smartphone ocular align-
ment measurement app in school screening for stra-
bismus. BMC ophthalmology, 21:1–10.
Choi, R. Y. and Kushner, B. J. (1998). The accuracy of
experienced strabismologists using the hirschberg and
krimsky tests. Ophthalmology, 105 7:1301–6.
de Almeida, J. D. S., Silva, A. C., de Paiva, A. C., and
Teixeira, J. A. M. (2012). Computational methodol-
ogy for automatic detection of strabismus in digital
images through hirschberg test. Computers in biology
and medicine, 42(1):135–146.
Dericio
˘
glu, V. and C¸ erman, E. (2019). Quantitative mea-
surement of horizontal strabismus with digital photog-
raphy. Journal of American Association for Pediatric
Ophthalmology and Strabismus, 23(1):18.e1–18.e6.
Durajczyk, M., Grudzi
´
nska, E., and Modrzejewska, M.
(2023). Present knowledge of modern technology and
virtual computer reality to assess the angle of strabis-
mus. Klinika Oczna / Acta Ophthalmologica Polonica,
125(1):13–16.
Google (2023). Google colaboratory. https://colab.research.
google.com/. Accessed: 2024-12-30.
Hashemi, H., Pakzad, R., Heydarian, S., Yekta, A.,
Aghamirsalim, M., Shokrollahzadeh, F., Khoshhal, F.,
Pakbin, M., Ramin, S., and Khabazkhoob, M. (2019).
Global and regional prevalence of strabismus: a com-
prehensive systematic review and meta-analysis. Stra-
bismus, 27:54 – 65.
Jocher, G., Chaurasia, A., and Qiu, J. (2023). Ultralytics
yolov8. https://github.com/ultralytics/ultralytics.
Khng, C. and Osher, R. H. (2008). Evaluation of the rela-
tionship between corneal diameter and lens diameter.
Journal of cataract and refractive surgery, 34.
Kirillov, A., Mintun, E., Ravi, N., Mao, H., Rolland, C.,
Gustafson, L., Xiao, T., Whitehead, S., Berg, A. C.,
Lo, W.-Y., Doll
´
ar, P., and Girshick, R. (2023). Seg-
ment anything.
Li, Z., Liu, F., Yang, W., Peng, S., and Zhou, J. (2022).
A survey of convolutional neural networks: Anal-
ysis, applications, and prospects. IEEE Transac-
Cost-Effective Strabismus Measurement with Deep Learning
603