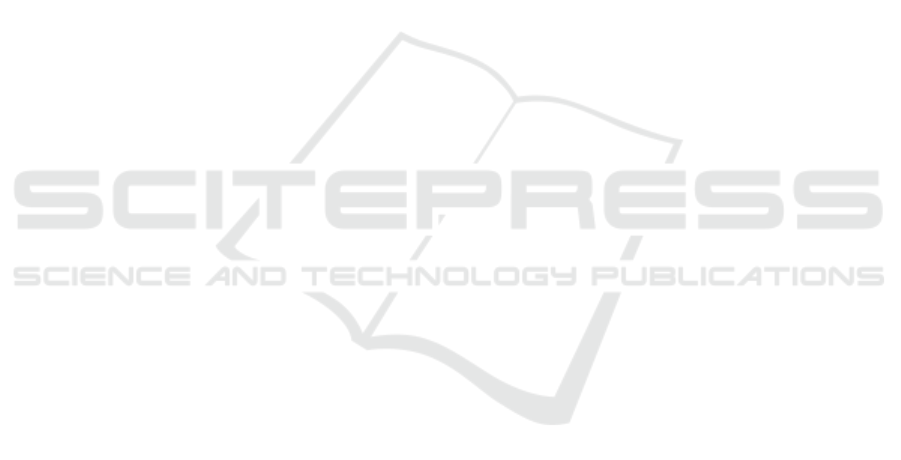
the skills to critically engage with these tools rather
than passively rely on them.
Finally, we believe that the proposed course may
serve as a laboratory for experimentation, poten-
tially generating insights and approaches that could be
adapted for use in other courses, including prerequi-
site ones. In fact, the very process of refining effective
activities and exercises for integrating LLMs without
compromising foundational programming skills may
contribute to the eventual obsolescence of the course
itself, as these best practices become embedded in
earlier stages of the curriculum.
6 CONCLUSIONS
We believe that it is of the upmost importance to in-
tegrate LLM-related training in the curricula of com-
puter science and engineering degrees. These tools
are already being used in the industry (DeBellis et al.,
2024), which means that companies will expect grad-
uates to have authentic experiences with the use of
these tools to produce working software. Although
some courses worldwide are already doing integra-
tions of these tools (as seen, for example, in (Kor-
pimies et al., 2024)), we believe that the topic’s im-
portance and depth justifies a new curricular unit.
Although further research is still needed to de-
velop the optimal pedagogical approaches and tech-
niques for integrating LLMs in computer science ed-
ucation, the sooner the CSE community starts to inte-
grate these tools, the sooner we will be able to develop
those approaches and techniques.
With this work we hope to contribute to the gen-
eral discussion of why, how, and when students should
be exposed to LLMs as software development support
tools. We have plans to begin implementing a pilot
version of the described course in the second semester
of the 2024/2025 school year. We will share any rele-
vant findings with the CSE community.
ACKNOWLEDGEMENTS
This research has received funding from the European
Union’s DIGITAL-2021-SKILLS-01 Programme un-
der grant agreement no. 101083594.
REFERENCES
Alves, P. and Cipriano, B. P. (2024). ”Give me the code”–
Log Analysis of First-Year CS Students’ Interactions
With GPT. arXiv preprint arXiv:2411.17855.
Asare, O., Nagappan, M., and Asokan, N. (2023). Is
GitHub’s Copilot as bad as humans at introducing vul-
nerabilities in code? Empirical Software Engineering,
28(6):129.
Babe, H. M., Nguyen, S., Zi, Y., Guha, A., Feldman, M. Q.,
and Anderson, C. J. (2023). Studenteval: A bench-
mark of student-written prompts for large language
models of code. arXiv preprint arXiv:2306.04556.
Barke, S., James, M. B., and Polikarpova, N. (2022).
Grounded copilot: How programmers interact with
code-generating models.(2022). CoRR arXiv, 2206.
Bloom, B. S., Engelhart, M. D., Furst, E. J., Hill, W. H., and
Krathwohl, D. R. (1964). Taxonomy of educational
objectives, volume 2. Longmans, Green New York.
Chen, L., Zaharia, M., and Zou, J. (2023). How is Chat-
GPT’s behavior changing over time? arXiv preprint
arXiv:2307.09009.
Cipriano, B. P. and Alves, P. (2024a). ”ChatGPT Is Here
to Help, Not to Replace Anybody” - An Evaluation
of Students’ Opinions On Integrating ChatGPT In CS
Courses. arXiv preprint arXiv:2404.17443.
Cipriano, B. P. and Alves, P. (2024b). LLMs Still
Can’t Avoid Instanceof: An investigation Into GPT-
3.5, GPT-4 and Bard’s Capacity to Handle Object-
Oriented Programming Assignments. In Proceedings
of the IEEE/ACM 46th International Conference on
Software Engineering: Software Engineering Educa-
tion and Training (ICSE-SEET).
Cipriano, B. P., Alves, P., and Denny, P. (2024). A Picture
Is Worth a Thousand Words: Exploring Diagram and
Video-Based OOP Exercises to Counter LLM Over-
Reliance. In European Conference on Technology En-
hanced Learning, pages 75–89. Springer.
Daun, M. and Brings, J. (2023). How ChatGPT Will
Change Software Engineering Education. In Proceed-
ings of the 2023 Conference on Innovation and Tech-
nology in Computer Science Education V. 1, ITiCSE
2023, page 110–116, New York, NY, USA. Associa-
tion for Computing Machinery.
DeBellis, D., Storer, K. M., Lewis, A., Good, B., Villalba,
D., Maxwell, E., Castillo, Kim, I., Michelle, and Har-
vey, N. (2024). 2024 Accelerate State of DevOps Re-
port. DORA and Google Cloud, Tech. Rep.
Denny, P., Leinonen, J., Prather, J., Luxton-Reilly, A.,
Amarouche, T., Becker, B. A., and Reeves, B. N.
(2023a). Promptly: Using Prompt Problems to Teach
Learners How to Effectively Utilize AI Code Genera-
tors. arXiv preprint arXiv:2307.16364.
Denny, P., Leinonen, J., Prather, J., Luxton-Reilly, A.,
Amarouche, T., Becker, B. A., and Reeves, B. N.
(2023b). Promptly: Using Prompt Problems to Teach
Learners How to Effectively Utilize AI Code Genera-
tors. arXiv:2307.16364 [cs].
Denny, P., Leinonen, J., Prather, J., Luxton-Reilly, A.,
Amarouche, T., Becker, B. A., and Reeves, B. N.
(2024). Prompt Problems: A new programming ex-
ercise for the generative AI era. In Proceedings of the
55th ACM Technical Symposium on Computer Science
Education V. 1, pages 296–302.
Programmers Aren’t Obsolete yet: A Syllabus for Teaching CS Students to Responsibly Use Large Language Models for Code Generation
419