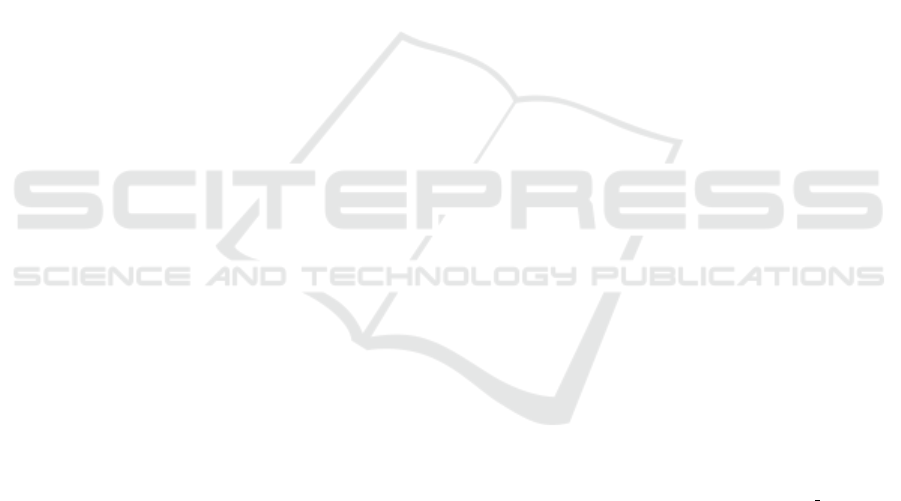
5 CONCLUSIONS
In conclusion, this study contributes a meaningful
tool to the fitness industry, offering an accessible and
effective means of posture correction for gym enthu-
siasts. Iterative improvements in model accuracy and
stability reinforce the model’s practical applicability,
while the final results demonstrate a reliable solution
for exercise optimization. The potential impact on re-
ducing injuries and enhancing exercise efficacy posi-
tions this application as a valuable asset for individu-
als and fitness institutions aiming to foster safer and
more effective workout environments.
The application of LSTM networks for sequential
data processing has proven effective in handling the
complex and dynamic nature of gym exercises. Ini-
tial training iterations revealed challenges related to
model accuracy and stability, including fluctuations
and underfitting. However, by refining the model ar-
chitecture—using techniques such as L2 regulariza-
tion, dropout adjustments, and lowering the learning
rate—subsequent iterations showed marked improve-
ments. The final model achieved a test accuracy of
87% and an F1 score of 0.87, reflecting robust learn-
ing and effective generalization.
While the model performed well in posture anal-
ysis, the reliance on 2D pose estimation limits its
ability to fully capture depth-related details in com-
plex movements. This limitation may affect feed-
back accuracy in exercises that involve multiple
joint movements. (Lozano-Mej
´
ıa et al., 2020) The
current model’s performance could benefit from a
more diverse dataset that includes a wider range of
body types, exercise intensities, and environments.
(Cornejo et al., 2021) Expanding the dataset would
enhance the model’s generalization across various
user demographics and workout conditions, contribut-
ing to more consistent feedback accuracy. (Ysique-
Neciosup et al., 2022)
Future research could focus on integrating 3D
pose estimation and conducting longitudinal stud-
ies to evaluate the application’s long-term impact on
users’ exercise habits, injury rates, and performance
improvements. Additionally, implementing personal-
ized feedback based on user-specific goals could fur-
ther tailor the fitness experience, making it more en-
gaging and effective.
REFERENCES
Ad
˜
ao, T., Gonzalez, D., Castilla, Y. C., P
´
erez, J.,
Shahrabadi, S., Sousa, N., Guevara, M., and Mag-
alh
˜
aes, L. G. (2022). Using deep learning to detect the
presence/absence of defects on leather: on the way to
build an industry-driven approach. Journal of Physics:
Conference Series, 2224(1):012009.
Bairaktaris, D. and Levy, J. (1993). Using old memories
to store new ones. In IJCNN, volume 2, pages 1163–
1166 vol.2.
Chen, K., Shin, J., Hasan, M. A. M., Liaw, J., Okuyama,
Y., and Tomioka, Y. (2022). Fitness movement types
and completeness detection using a transfer-learning-
based deep neural network. Sensors, 22(15):5700.
Cornejo, L., Urbano, R., and Ugarte, W. (2021). Mobile
application for controlling a healthy diet in peru using
image recognition. In FRUCT, pages 32–41. IEEE.
Ghojogh, B. and Ghodsi, A. (2023). Recurrent neural net-
works and long short-term memory networks: Tuto-
rial and survey. CoRR, abs/2304.11461.
Gionfrida, L., Wang, C., Gan, L., Chli, M., and Carlone, L.
(2024). Computer and robot vision: Past, present, and
future [TC spotlight]. IEEE Robotics Autom. Mag.,
31(3):211–215.
Kaewrat, C., Khundam, C., and Thu, M. (2024). Enhanc-
ing exercise monitoring and guidance through mobile
augmented reality: A comparative study of RGB and
lidar. IEEE Access, 12:95447–95460.
Kemler, E., Noteboom, L., and van Beijsterveldt, A.-M.
(2022). Characteristics of fitness-related injuries in
the netherlands: A descriptive epidemiological study.
Sports, 10(12).
Lozano-Mej
´
ıa, D. J., Vega-Uribe, E. P., and Ugarte, W.
(2020). Content-based image classification for sheet
music books recognition. In EIRCON, pages 1–4.
IEEE.
Lugaresi, C., Tang, J., Nash, H., McClanahan, C., Uboweja,
E., Hays, M., Zhang, F., Chang, C., Yong, M. G., Lee,
J., Chang, W., Hua, W., Georg, M., and Grundmann,
M. (2019). Mediapipe: A framework for building per-
ception pipelines. CoRR, abs/1906.08172.
Mallick, T., Das, P. P., and Majumdar, A. K. (2022). Posture
and sequence recognition for Bharatanatyam dance
performances using machine learning approaches. J.
Vis. Commun. Image Represent., 87:103548.
M
¨
uller, P. N., M
¨
uller, A. J., Achenbach, P., and G
¨
obel, S.
(2024). Imu-based fitness activity recognition using
cnns for time series classification. Sensors, 24(3):742.
Palanimeera, J. and Ponmozhi, K. (2023). Yap lstm: yoga
asana prediction using pose estimation and long short-
term memory. Soft Computing.
Vali, D., Venkata Chalapathi, M., Yellapragada, V.,
Purna Prakash, K., Challa, P., Gangishetty, D.,
Solanki, M., and Singhu, R. (2024). Physiotherapy
assistance for patients using human pose estimation
with raspberry pi. ASEAN Journal of Scientific and
Technological Reports, 27:e251096.
Ysique-Neciosup, J., Chavez, N. M., and Ugarte, W. (2022).
Deephistory: A convolutional neural network for au-
tomatic animation of museum paintings. Comput. An-
imat. Virtual Worlds, 33(5).
Mobile Application for Optimizing Exercise Posture Through Machine Learning and Computer Vision in Gyms
367