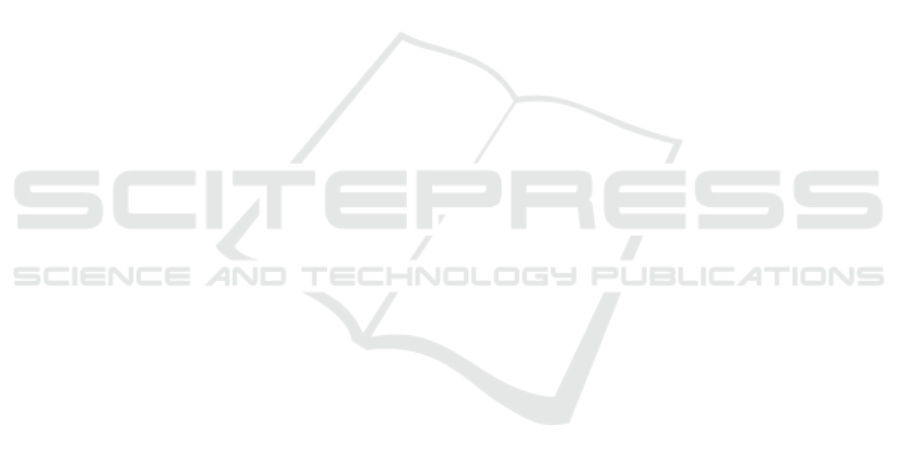
ACKNOWLEDGMENTS
The authors acknowledge the Coordenac¸
˜
ao de
Aperfeic¸oamento de Pessoal de N
´
ıvel Superior
(CAPES), Brazil - Finance Code 001, Conselho
Nacional de Desenvolvimento Cient
´
ıfico e Tec-
nol
´
ogico (CNPq), Brazil, and Fundac¸
˜
ao de Amparo
`
a
Pesquisa Desenvolvimento Cient
´
ıfico e Tecnol
´
ogico
do Maranh
˜
ao (FAPEMA) (Brazil) and Tribunal de
Contas do Estado do Maranh
˜
ao (TCE-MA) for the fi-
nancial support.
During the preparation of this work the authors
used ChatGPT in order to enhance the flow of the text
and DeepL as a translation assistant to improve flu-
ency. After using these tools, the authors reviewed
and edited the content as needed and take full respon-
sibility for the content of the publication.
REFERENCES
Adhikari, A., Ram, A., Tang, R., and Lin, J. (2019a).
Docbert: Bert for document classification.
Adhikari, A., Ram, A., Tang, R., and Lin, J. (2019b).
Rethinking complex neural network architectures for
document classification. In Proceedings of the 2019
Conference of the North American Chapter of the As-
sociation for Computational Linguistics: Human Lan-
guage Technologies, Volume 1 (Long and Short Pa-
pers), pages 4046–4051, Minneapolis, Minnesota. As-
sociation for Computational Linguistics.
Akiba, T., Sano, S., Yanase, T., Ohta, T., and Koyama, M.
(2019). Optuna: A next-generation hyperparameter
optimization framework.
Conneau, A., Khandelwal, K., Goyal, N., Chaudhary, V.,
Wenzek, G., Guzm
´
an, F., Grave, E., Ott, M., Zettle-
moyer, L., and Stoyanov, V. (2020). Unsupervised
cross-lingual representation learning at scale. In Juraf-
sky, D., Chai, J., Schluter, N., and Tetreault, J., editors,
Proceedings of the 58th Annual Meeting of the As-
sociation for Computational Linguistics, pages 8440–
8451, Online. Association for Computational Linguis-
tics.
Devlin, J., Chang, M.-W., Lee, K., and Toutanova, K.
(2019). BERT: Pre-training of deep bidirectional
transformers for language understanding. In Burstein,
J., Doran, C., and Solorio, T., editors, Proceedings
of the 2019 Conference of the North American Chap-
ter of the Association for Computational Linguistics:
Human Language Technologies, Volume 1 (Long and
Short Papers), pages 4171–4186, Minneapolis, Min-
nesota. Association for Computational Linguistics.
Khurana, D., Koli, A., Khatter, K., and Singh, S. (2023).
Natural language processing: state of the art, current
trends and challenges. Multimedia Tools and Applica-
tions, 82(3):3713–3744.
LENZA, P. (2020). Direito constitucional esquematizado.
Saraiva, S
˜
ao Paulo, 15. ed. rev. atual. ampl edition.
Loshchilov, I. and Hutter, F. (2019). Decoupled weight
decay regularization. In International Conference on
Learning Representations.
Naveed, H., Khan, A. U., Qiu, S., Saqib, M., Anwar, S., Us-
man, M., Akhtar, N., Barnes, N., and Mian, A. (2023).
A comprehensive overview of large language models.
Opitz, J. (2022). From bias and prevalence to macro f1,
kappa, and mcc: A structured overview of metrics for
multi-class evaluation.
Pe
˜
na, A., Morales, A., Fierrez, J., Serna, I., Ortega-Garcia,
J., Puente, I., C
´
ordova, J., and C
´
ordova, G. (2023).
Leveraging large language models for topic classifica-
tion in the domain of public affairs.
Song, D., Vold, A., Madan, K., and Schilder, F. (2022).
Multi-label legal document classification: A deep
learning-based approach with label-attention and
domain-specific pre-training. Inf. Syst., 106(C).
Stites, M. C., Howell, B. C., and Baxley, P. A. (2023).
Assessing the impact of automated document classi-
fication decisions on human decision-making. Tech-
nical report, Sandia National Lab.(SNL-NM), Albu-
querque, NM (United States).
TCE/MA (2023a). e-pca - sistema de prestac¸
˜
ao de contas
anual eletr
ˆ
onica.
TCE/MA (2023b). InstruC¸
˜
Ao normativa tce/ma nº 52, de
25 de outubro de 2017.
TCE/MA (2023c). Sistema de prestac¸
˜
ao de contas anual
eletr
ˆ
onica (epca) j
´
a est
´
a dispon
´
ıvel aos usu
´
arios.
Wan, L., Papageorgiou, G., Seddon, M., and Bernardoni, M.
(2019). Long-length legal document classification.
Xue, L., Constant, N., Roberts, A., Kale, M., Al-Rfou,
R., Siddhant, A., Barua, A., and Raffel, C. (2021).
mT5: A massively multilingual pre-trained text-to-
text transformer. In Toutanova, K., Rumshisky,
A., Zettlemoyer, L., Hakkani-Tur, D., Beltagy, I.,
Bethard, S., Cotterell, R., Chakraborty, T., and Zhou,
Y., editors, Proceedings of the 2021 Conference of the
North American Chapter of the Association for Com-
putational Linguistics: Human Language Technolo-
gies, pages 483–498, Online. Association for Compu-
tational Linguistics.
Comparative Study of Large Language Models Applied to the Classification of Accountability Documents
951