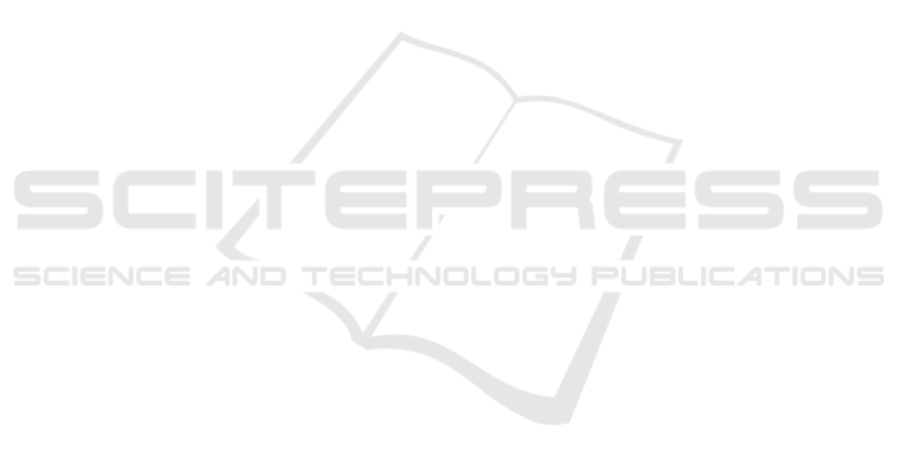
integrating data from other clients, FL effectively mit-
igated these limitations, enabling the global model to
address class imbalances and improve prediction ac-
curacy. Compared to traditional centralized learning
approaches, this combination not only preserves data
privacy but also enhances model robustness by lever-
aging distributed knowledge, making it particularly
effective in scenarios with class imbalances or miss-
ing labels.
The findings suggest that synthetic data and FL
are not only complementary but also mutually rein-
forcing. Synthetic data provides the foundation for
privacy-preserving ML, while FL extends this foun-
dation to handle more complex challenges inherent
in decentralized environments. Together, these ap-
proaches form a robust framework for developing
high-performing and privacy-conscious ML models
suitable for real-world applications.
For future work, there are several potential direc-
tions to build upon our current findings. First, the im-
pact of alternative synthetic data generation methods
could be examined, focusing on how different tech-
niques influence model performance in both central-
ized and FL frameworks. Furthermore, expanding the
scope of the study to include diverse datasets from
various domains would help validate the robustness
and applicability of the proposed approach. Another
promising avenue involves testing more advanced
classification algorithms to explore their potential for
improving both predictive accuracy and generaliza-
tion across heterogeneous environments. These direc-
tions would collectively contribute to a deeper under-
standing of the interplay between synthetic data and
FL in addressing real-world ML challenges.
REFERENCES
Ahmed, S., Alshater, M. M., El Ammari, A., and Ham-
mami, H. (2022). Artificial intelligence and machine
learning in finance: A bibliometric review. Research
in International Business and Finance, 61:101646.
Aktas¸, M., Akkus¸ Halepmollası, R., and T
¨
oreyin, B. U.
(2024). Enhancing credit risk assessment with fed-
erated learning through a comparative study. In 8th
EAI International Conference on Robotic Sensor Net-
works.
Assefa, S. A., Dervovic, D., Mahfouz, M., Tillman, R. E.,
Reddy, P., and Veloso, M. (2020). Generating syn-
thetic data in finance: opportunities, challenges and
pitfalls. In Proceedings of the First ACM International
Conference on AI in Finance, pages 1–8.
Awosika, T. et al. (2023). Transparency and privacy: The
role of explainable ai and federated learning in finan-
cial fraud detection. Journal of Financial Technology
and Ethics, 8(1):15–30.
Bottou, L. (2010). Large-scale machine learning with
stochastic gradient descent. In Proceedings of COMP-
STAT’2010, pages 177–186. Springer.
Cao, L. (2022). Ai in finance: challenges, techniques,
and opportunities. ACM Computing Surveys (CSUR),
55(3):1–38.
Cohen, I. G. and Mello, M. M. (2018). Hipaa and pro-
tecting health information in the 21st century. Jama,
320(3):231–232.
Cortes, C. and Vapnik, V. (1995). Support-vector networks.
Machine Learning, 20(3):273–297.
Goodfellow, I., Bengio, Y., and Courville, A. (2016). Deep
Learning. MIT Press.
Ho, J., Jain, A., and Abbeel, P. (2020). Denoising diffusion
probabilistic models. Advances in neural information
processing systems, 33:6840–6851.
Hoofnagle, C. J., Van Der Sloot, B., and Borgesius, F. Z.
(2019). The european union general data protection
regulation: what it is and what it means. Information
& Communications Technology Law, 28(1):65–98.
Hosmer, D. W., Lemeshow, S., and Sturdivant, R. X. (2013).
Applied Logistic Regression. Wiley.
Jolicoeur-Martineau, A. et al. (2023). Generating and im-
puting tabular data via diffusion and flow based gra-
dient boosted trees. Advances in Neural Information
Processing Systems (NeurIPS).
Khaled, A. et al. (2024). Synthetic data generation and
impact analysis of machine learning models for en-
hanced credit card fraud detection. Journal of Artifi-
cial Intelligence and Applications, 12(3):45–60.
Lu, Y., Shen, M., Wang, H., Wang, X., van Rechem, C.,
Fu, T., and Wei, W. (2023). Machine learning for
synthetic data generation: a review. arXiv preprint
arXiv:2302.04062.
McMahan, B., Moore, E., Ramage, D., Hampson, S., and
y Arcas, B. A. (2017). Communication-efficient learn-
ing of deep networks from decentralized data. In Ar-
tificial intelligence and statistics, pages 1273–1282.
PMLR.
Mothukuri, V., Parizi, R. M., Pouriyeh, S., Huang, Y., De-
hghantanha, A., and Srivastava, G. (2021). A survey
on security and privacy of federated learning. Future
Generation Computer Systems, 115:619–640.
Sattarov, E. et al. (2023). Findiff: Diffusion models for
financial tabular data generation. Financial Data Sci-
ence Journal, 9(2):75–90.
Sohl-Dickstein, J., Weiss, E., Maheswaranathan, N., and
Ganguli, S. (2015). Deep unsupervised learning us-
ing nonequilibrium thermodynamics. In International
conference on machine learning, pages 2256–2265.
PMLR.
Truong, N., Sun, K., Wang, S., Guitton, F., and Guo, Y.
(2021). Privacy preservation in federated learning: An
insightful survey from the gdpr perspective. Comput-
ers & Security, 110:102402.
¨
Ulver, B., Yurto
˘
glu, R. A., Dervis¸o
˘
glu, H., Halepmollası,
R., and Haklıdır, M. (2023). Federated learning in pre-
dicting heart disease. In 2023 31st Signal Processing
and Communications Applications Conference (SIU),
pages 1–4. IEEE.
FEMIB 2025 - 7th International Conference on Finance, Economics, Management and IT Business
88