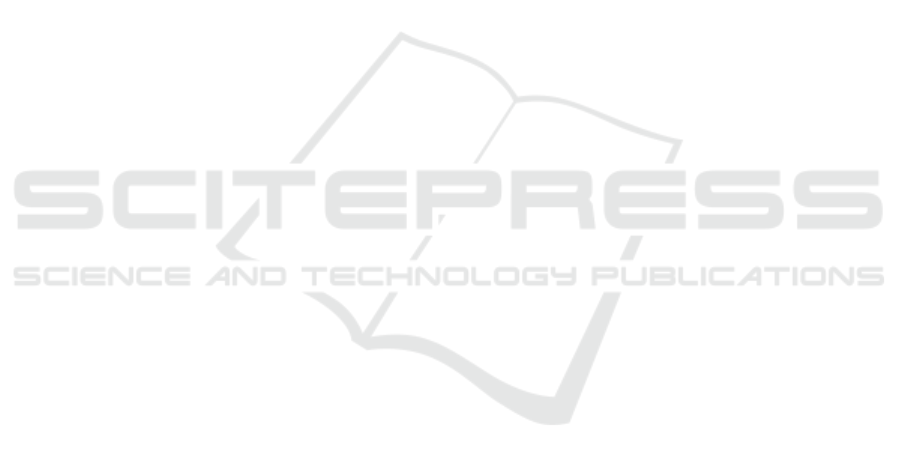
2014) introduced the SenTube corpus, a dataset de-
signed for sentiment analysis in multiple languages.
Together, these studies show how sentiment analysis
on YouTube has grown from basic dataset creation to
more advanced models that can handle a wider range
of content and languages.
Sentiment analysis has been widely used by re-
searchers on YouTube, but there were also other ap-
proaches regarding user participation in the com-
ments. It is important to note that the following re-
searches were done in pre-recorded posted videos on
YouTube, not live streams. Recent studies have ex-
plored various aspects of YouTube comments, from
informal learning to public discourse and content
moderation. Dubovi and Tabak (Dubovi and Tabak,
2020) focused on how YouTube comments foster
knowledge co-construction, particularly in science
videos. Their research demonstrated that user com-
ments often go beyond simple information sharing,
engaging in discussions and debates that lead to
deeper learning.
Other researchers have examined the broader dy-
namics of YouTube comments, particularly their im-
pact on engagement and content quality. Siersdorfer
et al. (Siersdorfer et al., 2010) analyzed over six mil-
lion comments to understand the factors that influ-
ence comment ratings and usefulness. They found
a clear correlation between positive sentiment and
higher community ratings, while offensive language
led to negative ratings. Their work also introduced
machine learning classifiers to predict which com-
ments would be rated positively or negatively by the
community, providing insights into how sentiment
and language shape user interactions on the platform.
Comment analysis on YouTube has also been ap-
plied to specific issues such as hate speech and spam.
Latorre and Amores (Latorre and Amores, 2021)
used topic modeling to identify racist and xenopho-
bic comments targeting migrants and refugees, show-
ing that 19.35 percent of the analyzed comments con-
tained hate speech. This highlights the darker side of
YouTube comments, where far-right rhetoric and na-
tionalist views are prevalent in certain channels. Ab-
dullah et al.(Abdullah et al., 2018) took a different ap-
proach, focusing on spam detection in YouTube com-
ments. They compared various spam filtering tech-
niques, finding that low-complexity algorithms can
achieve high accuracy in identifying spam content,
suggesting that YouTube’s built-in tools could be en-
hanced to combat the spread of malicious content.
These studies show that while YouTube comments of-
fer valuable insights into user sentiment and interac-
tion, they also present challenges related to content
moderation and the spread of harmful speech.
More specifically about YouTube comments anal-
ysis in live streams, we have some relevant findings.
Recent research focuses on understanding user behav-
ior, emotional intensity, and content moderation dur-
ing live events. Sentiment analysis was one of the
tools used to analyze live stream comments. Tirpude
et al. (Tirpude et al., 2024) developed a system to
analyze sentiments in live chat through Natural Lan-
guage Processing (NLP) techniques. By using Fast-
Text for sentiment scoring and emoji analysis, they
provided real-time insights into audience reactions,
enabling content creators to adjust their approach dy-
namically during live streams. Similarly, Liebeskind
et al. (Liebeskind et al., 2021) investigated engage-
ment patterns in YouTube live chats, specifically dur-
ing political events such as Donald Trump’s 2020
campaign. Their study revealed that live comments
were highly emotional, with a significant portion be-
ing abusive, but frequent commenters tended to use
less offensive language, emphasizing how emotional
involvement plays a role in live chat dynamics.
Emotional intensity in live streams has been an-
other focus, as evidenced by the works of Luo et
al. (Luo et al., 2020) and Guo and Fussell (Guo and
Fussell, 2020). Luo et al. explored how emotions are
amplified in live chat compared to comments posted
after events, finding that shared real-time experiences
intensify both positive and negative sentiments. This
emotional amplification has implications for content
moderation, as heightened emotions can lead to an in-
crease in abusive or toxic comments. Guo and Fussell
took this further by examining emotional contagion in
live-streaming environments, showing that the senti-
ments in chat messages have a stronger influence on
subsequent messages than the content of the video it-
self. Their findings suggest that audience interactions
can significantly shape the overall sentiment of live
chat, often more than the live content itself.
In addition to understanding emotional dynam-
ics, other researchers have focused on combat-
ing challenges such as spam and toxicity in live
chats. Yousukkee and Wisitpongphan (Yousukkee
and Wisitpongphan, 2021) analyzed spammers’ be-
havior in live streams, using machine learning models
to differentiate between spam and legitimate user en-
gagement. Their decision tree classifier achieved high
accuracy in detecting repetitive, irrelevant content.
Complementing this, Tarafder (Tarafder et al., 2023)
developed an automated tool to identify and flag
toxic comments in real-time, addressing the increas-
ing need for content moderation during live streams,
especially as platforms like YouTube have seen a
surge in usage during the pandemic.
An interesting recent tool that is worth mentioning
StreamVis: An Analysis Platform for YouTube Live Chat Audience Interaction, Trends and Controversial Topics
633