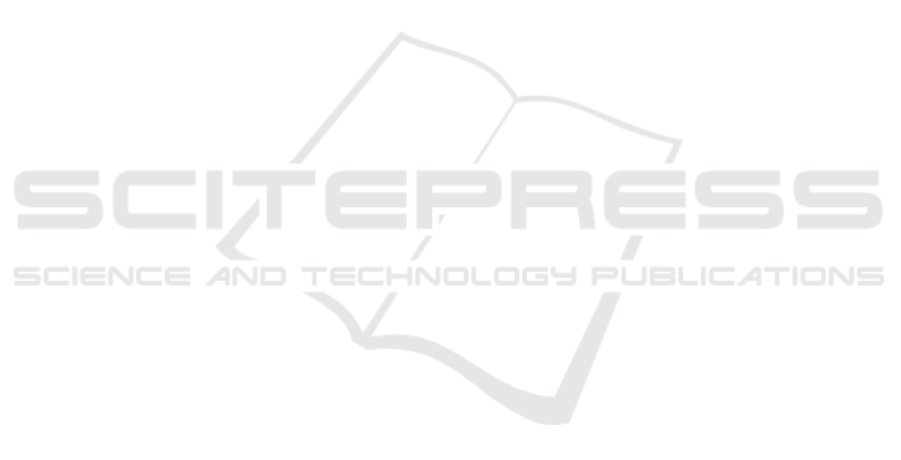
can explain the advantages of each and recommend
which model might be most suitable for the task at
hand.
The AutoML system autonomously selects and
evaluates a range of ML models based on the config-
uration. The LLM helps users understand the selec-
tion process, explaining how AutoML identifies the
best models for the problem and adjusts hyperparame-
ters to maximize performance. Users are not required
to understand the underlying technicalities; the LLM
simplifies these concepts into user-friendly language.
The AutoML Module (see Figure 1) is the core
component of the framework, responsible for au-
tomating the ML workflow. This layer handles the
bulk of the computational processes, allowing users
to focus on higher-level decisions. Based on the con-
figuration, the AutoML module trains multiple ML
models using the dataset. This could include mod-
els like Random Forests, Support Vector Machines, or
neural networks. The system automatically splits the
data into training and validation sets, ensuring proper
model evaluation.
The AutoML system performs hyperparameter
optimization (see Figure 1) to fine-tune model pa-
rameters and improve the accuracy of the trained
models. Methods like grid search, random search,
or Bayesian optimization are applied to find the best
possible configuration for each model. After training,
the models are evaluated using appropriate metrics.
For classification tasks, common evaluation metrics
include accuracy, precision, recall, and F1-score. For
regression, metrics such as mean squared error (MSE)
or R-squared are used. The system generates an eval-
uation report that allows the user to assess model per-
formance.
Following the initial model evaluation, the frame-
work enters a feedback loop that integrates the LLM
to refine and optimize the process. The LLM reviews
the evaluation results and provides the user with an
easy-to-understand analysis of model performance.
For example, it can explain why a model performed
well or poorly based on certain features or hyperpa-
rameter settings. The LLM also offers visualizations
to help the user understand the model’s strengths and
weaknesses.
Based on the evaluation metrics, the LLM sug-
gests adjustments to improve model performance. For
instance, if the model performs poorly on certain sub-
sets of data, the LLM might recommend using addi-
tional features, applying different preprocessing tech-
niques, or altering the hyperparameter settings. The
user can ask the LLM specific questions like, “What
can we do to improve the model?” or “Why did the
model underperform?” The LLM interprets the user’s
queries and helps refine the AutoML pipeline accord-
ingly, guiding the user through the process of improv-
ing the model.
Once the LLM provides feedback and sugges-
tions, the framework enters an iterative process of
model reconfiguration. The AutoML system automat-
ically applies any suggested changes, such as retrain-
ing the model with different hyperparameters or addi-
tional data features. The LLM ensures that the user is
aware of all adjustments and their implications. The
training, evaluation, and refinement cycle continues
until the best model is identified, ensuring that the
user receives a model with optimal performance tai-
lored to their problem.
After the model has been trained and optimized,
the framework moves to the Deployment Layer (see
Figure 1), where the model is put into production. The
selected model is deployed into a production environ-
ment. The deployment process is automated, ensuring
that the user does not need to manage the intricacies
of model deployment. The LLM can help explain the
deployment process and provide guidance on how to
integrate the model into existing systems.
The system continuously monitors the model’s
performance in the real world. The LLM alerts the
user to potential issues, such as data drift or declining
accuracy, and suggests corrective actions. The LLM
can also assist in retraining the model if performance
degradation occurs over time.
Interpretability and explainability are critical for
understanding how models make decisions, especially
for non-experts. Thus, the Interpretability Layer
(see Figure 1) ensures that users can trust and un-
derstand their models. The LLM explains the rea-
soning behind the model’s predictions in a way that
is accessible to the user. For instance, it might say,
“The model predicted this outcome because there was
a high correlation between the values of feature X and
thos of the target variable”. This enhances the user’s
confidence in the model’s decisions. The LLM also
provides insights into the dataset and model predic-
tions, highlighting patterns in the data that the model
has identified. This helps the user gain a deeper un-
derstanding of the problem domain and the model’s
behavior.
The Resource Optimization Layer (see Figure
1) focuses on ensuring the framework operates effi-
ciently, even with large datasets or complex models.
The system manages computational resources, such
as GPUs and CPUs, to ensure cost-effective training
and inference. The LLM can suggest resource opti-
mization strategies to the user, helping them balance
performance with computational cost. The system is
designed to scale with the complexity of the task, en-
ICEIS 2025 - 27th International Conference on Enterprise Information Systems
962