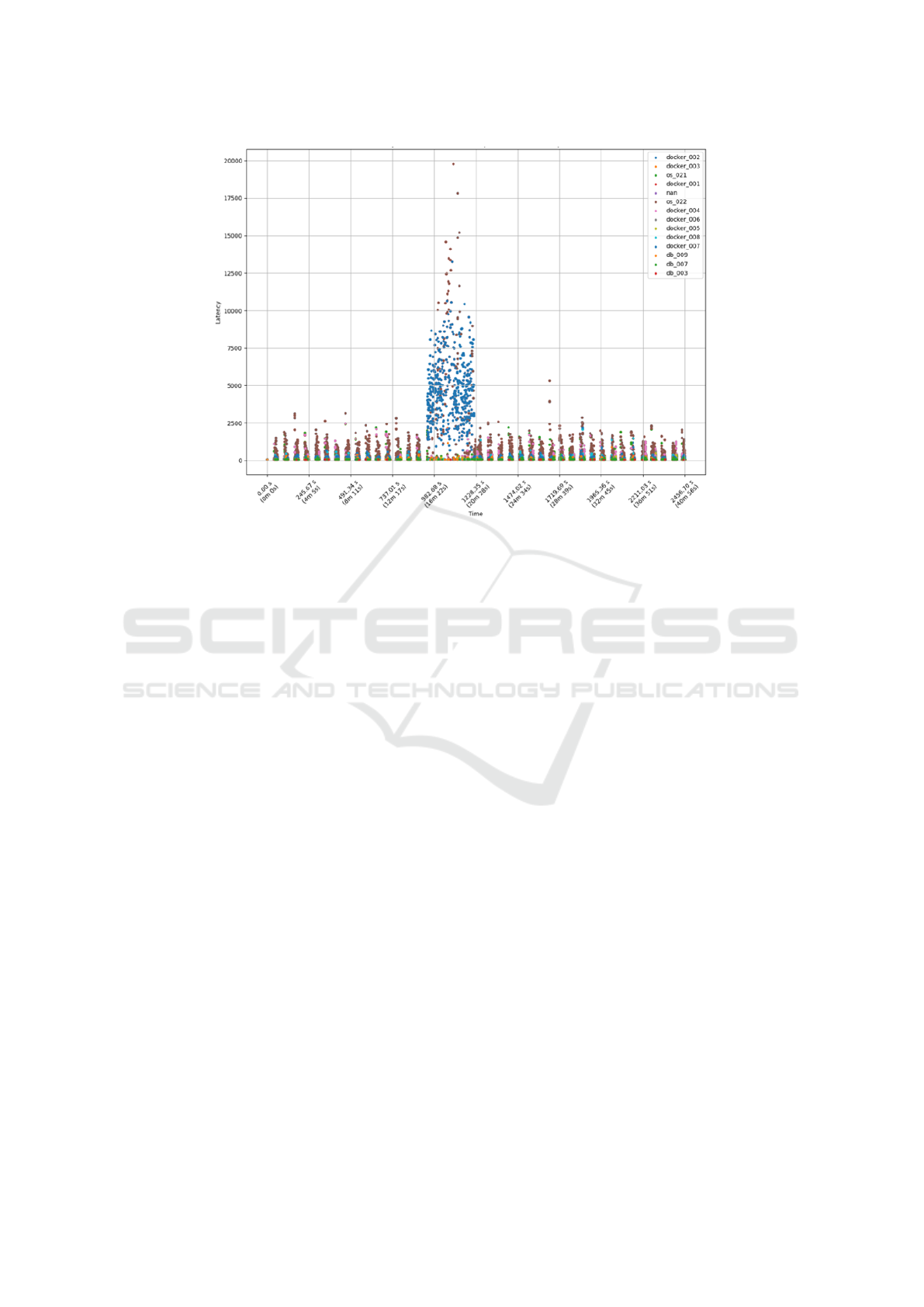
Figure 9: Latency Distribution over Time.
Fonseca, R., Porter, G., Katz, R. H., Shenker, S., and Sto-
ica, I. (2007). X-trace: A pervasive network tracing
framework. In USENIX Symposium.
Forsberg, V. (2019). Automatic anomaly detection and root
cause analysis for microservice clusters. Master’s the-
sis, Ume
˚
a University.
Hadi, R. and Girsang, A. S. (2023). Root cause analysis for
it incident using artificial neural network (ann). Jour-
nal of System and Management Sciences, 13.
Ikram, A., Chakraborty, S., Mitra, S., Saini, S. K., Bagchi,
S., and Kocaoglu, M. (2022). Root cause analysis of
failures in microservices through causal discovery. In
International Conference on Software Engineering.
Landim, L. P. T. (2023). Monitoring and detection of
anomaly in microservices environments. Master’s the-
sis, Polytechnic Institute of Castelo Branco.
Li, B., Yang, T., Chen, Z., Su, Y., Yang, Y., and Lyu, M.
(2024). Heterogeneous anomaly detection for soft-
ware systems via attentive multi-modal learning. In
ICSE.
Li, Z., Chen, J., Jiao, R., Zhao, N., Wang, Z., Zhang, S.,
Wu, Y., Jiang, L., Yan, L., Wang, Z., Chen, Z., Zhang,
W., Nie, X., Su, K., and Pei, D. (2021). Practical root
cause localization for microservice systems via trace
analysis. In Intl Symp on Quality of Service.
Merkel, D. (2014). Docker: lightweight linux containers for
consistent development and deployment. Linux Jrnl.
Mohamed, H. and El-Gayar, O. (2021). End-to-end latency
prediction of microservices workflow on kubernetes:
A comparative evaluation of machine learning models
and resource metrics. In HICSS.
Pahl, C. (2023). Research challenges for machine learning-
constructed software. Service Oriented Computing
and Applications, 17(1):1–4.
Samir, A. and Pahl, C. (2020). Detecting and localizing
anomalies in container clusters using markov models.
Electronics.
Samir, A. and Pahl, C. (2021). Autoscaling recovery ac-
tions for container-based clusters. Concurrency and
Computation: Practice and Experience, 33(23).
Scolati, R., Fronza, I., El Ioini, N., Samir, A., Barzegar,
H. R., and Pahl, C. (2020). A containerized edge cloud
architecture for data stream processing. In Lecture
Notes in Computer Science. Springer.
Sigelman, B. H., Barroso, L. A., Burrows, M., Stephenson,
P., Plakal, M., Beaver, D., Jaspan, S., and Shanbhag,
C. (2010). Dapper, a large-scale distributed systems
tracing infrastructure. Google research.
Sundberg S., Brunstrom A., F.-R. S. and S., C. (2024). Mea-
suring network latency from a wireless isp: Variations
within and across subnets. Preprint.
von Leon, D., Miori, L., Sanin, J., El Ioini, N., Helmer, S.,
and Pahl, C. A lightweight container middleware for
edge cloud architectures. Fog and Edge Computing:
Principles and Paradigms.
Wang, R., Qiu, H., Cheng, X., and Liu, X. (2023). Anomaly
detection with a container-based stream processing
framework for industrial internet of things. Journal
of Industrial Information Integration, 35.
Wang, Y., Zhu, Z., Fu, Q., Ma, Y., and He, P. (2024).
MRCA: Metric-level root cause analysis for microser-
vices via multi-modal data. In ASE.
Yu, G., Chen, P., Chen, H., Guan, Z., Huang, Z., Jing, L.,
Weng, T., Sun, X., and Li, X. (2024). Microrank: End-
to-end latency issue localization with extended spec-
trum analysis in microservice environments. In Con-
ference on Computer Communications.
Hybrid Root Cause Analysis for Partially Observable Microservices Based on Architecture Profiling
263