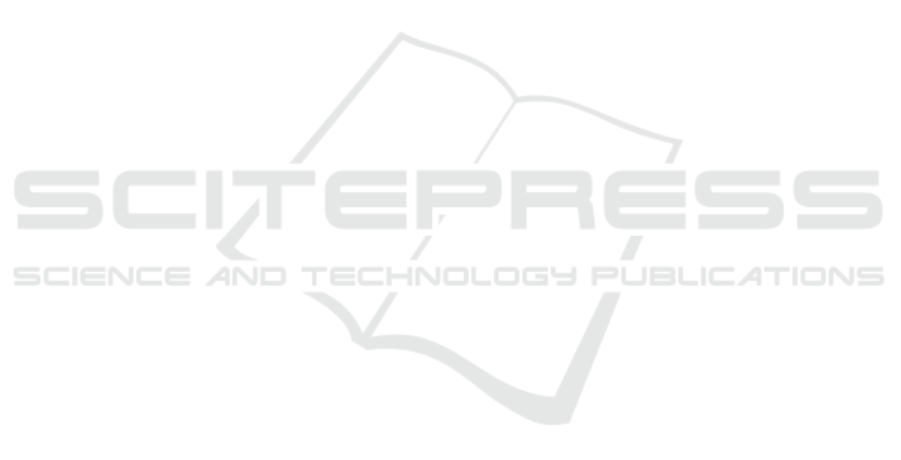
light areas for further research and improvement.
6.1 Future Work
While our MMS has provided a comprehensive
overview of the current landscape of artifact traceabil-
ity, there are several avenues for future research and
exploration in this area. First, future studies could
delve deeper into specific industries or domains to
understand how traceability challenges vary across
different contexts. Additionally, further longitudinal
studies could investigate the evolution of traceabil-
ity practices over time and assess the effectiveness of
interventions and tools in addressing identified chal-
lenges. It is clear that there is an imminent need
for continued research and development of innovative
tools and techniques to support artifact traceability in
practice. This includes the exploration of automated
tracing algorithms, integration of traceability mecha-
nisms into existing development workflows, and the
development of frameworks for assessing the quality
and completeness of traceability information.
REFERENCES
Abbas, M., Ferrari, A., Shatnawi, A., Enoiu, E., Saadat-
mand, M., and Sundmark, D. (2022a). Correction to:
On the relationship between similar requirements and
similar software: A case study in the railway domain.
Requirements Engineering, 27(3):399–399.
Abbas, M., Ferrari, A., Shatnawi, A., Enoiu, E., Saadat-
mand, M., and Sundmark, D. (2022b). On the re-
lationship between similar requirements and similar
software: A case study in the railway domain. Re-
quirements Engineering.
Aksnes, D. W., Langfeldt, L., and Wouters, P. (2019). Ci-
tations, citation indicators, and research quality: An
overview of basic concepts and theories. SAGE Open,
9(1):2158244019829575.
Aung, T. W. W., Huo, H., and Sui, Y. (2020). A literature re-
view of automatic traceability links recovery for soft-
ware change impact analysis. In Proceedings of the
28th International Conference on Program Compre-
hension, ICPC ’20, page 14–24, New York, NY, USA.
Association for Computing Machinery.
Garousi, V., Felderer, M., and M
¨
antyl
¨
a, M. V. (2016). The
need for multivocal literature reviews in software en-
gineering: complementing systematic literature re-
views with grey literature. In Proceedings of the 20th
International Conference on Evaluation and Assess-
ment in Software Engineering, EASE ’16, New York,
NY, USA. Association for Computing Machinery.
Garousi, V., Felderer, M., and M
¨
antyl
¨
a, M. V. (2019).
Guidelines for including grey literature and conduct-
ing multivocal literature reviews in software engineer-
ing. Information and Software Technology, 106:101–
121.
Gotel, O., Cleland-Huang, J., Hayes, J. H., Zisman, A.,
Egyed, A., Gr
¨
unbacher, P., Dekhtyar, A., Antoniol,
G., and Maletic, J. (2012). The Grand Challenge of
Traceability (v1.0), pages 343–409. Springer London,
London.
Guo, J., Cheng, J., and Cleland-Huang, J. (2017). Semanti-
cally enhanced software traceability using deep learn-
ing techniques. In 2017 IEEE/ACM 39th International
Conference on Software Engineering (ICSE), pages 3–
14.
Harzing, A. W. K. and van der Wal, R. (2008). Google
scholar as a new source for citation analysis. Ethics
Sci. Environ. Polit., 8:61–73.
Kitchenham, B. A. and Charters, S. (2007). Guidelines for
performing systematic literature reviews in software
engineering. Technical Report EBSE 2007-001, Keele
University and Durham University Joint Report.
Lefebvre, C., Manheimer, E., and Glanville, J. (2008).
Searching for studies. Cochrane handbook for system-
atic reviews of interventions: Cochrane book series,
pages 95–150.
Lyu, Y., Cho, H., Jung, P., and Lee, S. (2023). A systematic
literature review of issue-based requirement traceabil-
ity. Ieee Access, 11:13334–13348.
Mahmoud, A. and Niu, N. (2014). On the role of seman-
tics in automated requirements tracing. Requirements
Engineering, 20(3):281–300.
Neto, G. T. G., Santos, W. B., Endo, P. T., and Fagundes,
R. A. (2019). Multivocal literature reviews in soft-
ware engineering: Preliminary findings from a tertiary
study. In 2019 ACM/IEEE International Symposium
on Empirical Software Engineering and Measurement
(ESEM), pages 1–6.
Pauzi, Z. and Capiluppi, A. (2023). Applications of natural
language processing in software traceability: A sys-
tematic mapping study. Journal of Systems and Soft-
ware, 198:111616.
Saleem, M. and Minhas, N. M. (2018). Information retrieval
based requirement traceability recovery approaches-a
systematic literature review. University of Sindh Jour-
nal of Information and Communication Technology,
2(4):180–188.
Tufail, H., Masood, M. F., Zeb, B., Azam, F., and Anwar,
M. W. (2017). A systematic review of requirement
traceability techniques and tools. In 2017 2nd inter-
national conference on system reliability and safety
(ICSRS), pages 450–454. IEEE.
Wang, B., Peng, R., Li, Y., Lai, H., and Wang, Z. (2018).
Requirements traceability technologies and technol-
ogy transfer decision support: A systematic review.
Journal of Systems and Software, 146:59–79.
Zhou, X., Jin, Y., Zhang, H., Li, S., and Huang, X. (2016).
A map of threats to validity of systematic literature
reviews in software engineering. In 2016 23rd Asia-
Pacific Software Engineering Conference (APSEC),
pages 153–160, Hamilton, New Zealand. IEEE.
A Multivocal Mapping Study on Artifact Traceability Complexities in Practice
761