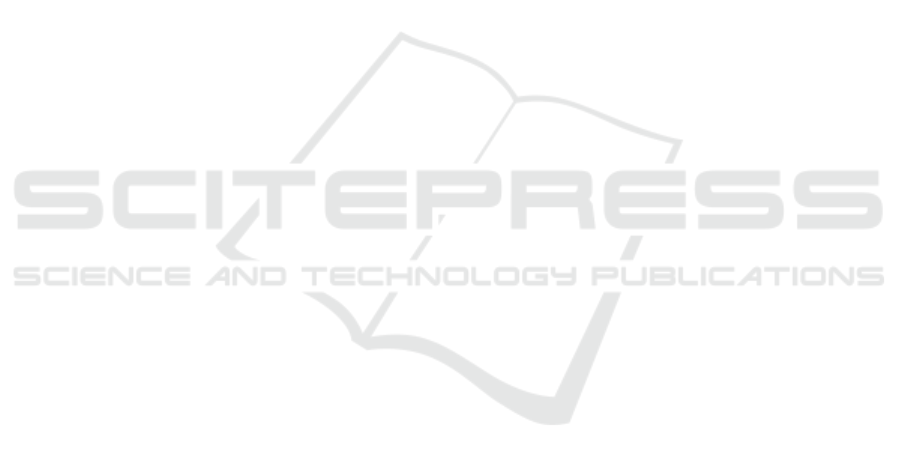
formance and areas for further refinement.
Moving forward, we plan to further enhance the
system by refining its contextual modeling to bet-
ter reflect real-world sanitary management processes.
This includes improving the ontology to more accu-
rately represent the dynamics of disease control and
agricultural management. Additionally, we will de-
velop and implement new adaptive rules within the
system, allowing it to respond more effectively to di-
verse outbreak scenarios. These improvements aim to
streamline the user experience while ensuring the sys-
tem remains practical, responsive, and aligned with
the needs of its users in managing sanitary events.
ACKNOWLEDGMENTS
This research is supported by FUNDESA, project
“Combining Process Mapping and Improvement with
BPM and the Application of Data Analytics in
the Context of Animal Health Defense and Inspec-
tion of Animal-Origin Products in the State of RS”
(UFSM/060496) and FAPERGS, grant n. 24/2551-
0001401-2. The research by Vin
´
ıcius Maran is par-
tially supported by CNPq grant 306356/2020-1 DT-
2, CNPq PIBIC and PIBIT program and FAPERGS
PROBIC program.
REFERENCES
Abowd, G. D. and Mynatt, E. D. (2000). Charting past,
present, and future research in ubiquitous computing.
ACM Transactions on Computer-Human Interaction
(TOCHI), 7(1):29–58.
Ala’a, B. et al. (2024). Newcastle disease outbreak in the
state of rio grande do sul, brazil 2024: Is there any im-
pact on jordan as one of the major importers of brazil-
ian poultry?
Alexander, D. J. (2012). Newcastle disease, volume 8.
Springer Science & Business Media.
BBC (2025). Uk bans cattle, pigs, and sheep imports from
germany after foot-and-mouth case. Accessed: 2025-
01-16.
Bizer, C., Heath, T., and Berners-Lee, T. (2023). Linked
data-the story so far. In Linking the World’s Infor-
mation: Essays on Tim Berners-Lee’s Invention of the
World Wide Web, pages 115–143.
Cardenas, N. C., Lopes, F. P., Machado, A., Maran, V.,
Trois, C., Machado, F. A., and Machado, G. (2024).
Modeling foot-and-mouth disease dissemination in rio
grande do sul, brazil and evaluating the effectiveness
of control measures. Frontiers in Veterinary Science,
11:1468864.
Chen, H., Finin, T., and Joshi, A. (2003). An ontology
for context-aware pervasive computing environments.
The knowledge engineering review, 18(3):197–207.
Chihani, B., Bertin, E., Jeanne, F., and Crespi, N. (2011).
Context-aware systems: a case study. In DICTAP
2011, Dijon, France, June 21-23, 2011, Proceedings,
Part II, pages 718–732. Springer.
Cook, D. and Das, S. K. (2004). Smart environments: tech-
nology, protocols, and applications, volume 43. John
Wiley & Sons.
Descovi, G., Maran, V., Ebling, D., and Machado, A.
(2021). Towards a blockchain architecture for animal
sanitary control. In ICEIS (1), pages 305–312.
Dey, A. K., Kortuem, G., Morse, D. R., and Schmidt, A.
(2001). Situated interaction and context-aware com-
puting. Personal and Ubiquitous Computing, 5:1–3.
D
´
orea, F. C. and Vial, F. (2016). Animal health syn-
dromic surveillance: a systematic literature review of
the progress in the last 5 years (2011–2016). Veteri-
nary Medicine: Research and Reports, pages 157–
170.
D
´
orea, F. C. e. a. (2019). Drivers for the development of an
animal health surveillance ontology (ahso). Preven-
tive veterinary medicine, 166:39–48.
Gruber, T. R. (1993). A translation approach to portable
ontology specifications. Knowledge acquisition,
5(2):199–220.
Grubman, M. J. and Baxt, B. (2004). Foot-and-mouth dis-
ease. Clinical microbiology reviews, 17(2):465–493.
Gu, T., Wang, X. H., Pung, H. K., and Zhang, D. Q. (2020).
An ontology-based context model in intelligent envi-
ronments. arXiv preprint arXiv:2003.05055.
Guarino, N., Oberle, D., and Staab, S. (2009). What is an
ontology? Handbook on ontologies, pages 1–17.
Moreno, D., Ochoa, S. F., and Meseguer, R. (2015). A
context-aware model to provide positioning in disaster
relief scenarios. Sensors, 15(10):25176–25207.
Noy, N. F., McGuinness, D. L., et al. (2001). Ontology
development 101: A guide to creating your first ontol-
ogy.
Perera, C., Zaslavsky, A., Christen, P., and Georgakopoulos,
D. (2014). Context aware computing for the internet
of things. ieee communication survey and tutorials. 16
(1): 414–454.
Rauber, R. H. (2023). Avian influenza and the brazilian
poultry production: Current situation and prevention
strategies. Ger J Vet Res [Internet], 3(4):13–9.
Rural, G. (2024). Governo vai informar fim do foco de
doenc¸a de newcastle a
´
org
˜
ao internacional de sa
´
ude
animal. Accessed: 2025-01-16.
Rushton, J. (2009). The economics of animal health and
production. Cabi.
Secretaria da Agricultura, Pecu
´
aria e Desenvolvimento Ru-
ral (2024). Agricultura conclui vigil
ˆ
ancia a pro-
priedades num raio de 5 km do foco de gripe avi
´
aria
em rio pardo. Accessed: 2024-12-17.
Swayne, D. E. (2009). Avian influenza. John Wiley & Sons.
Terence, S., Immaculate, J., Raj, A., and Nadarajan, J.
(2024). Systematic review on internet of things in
smart livestock management systems. Sustainability,
16(10):4073.
Towards an Ontology-Based Approach for Enhancing Animal Sanitary Event Management
641