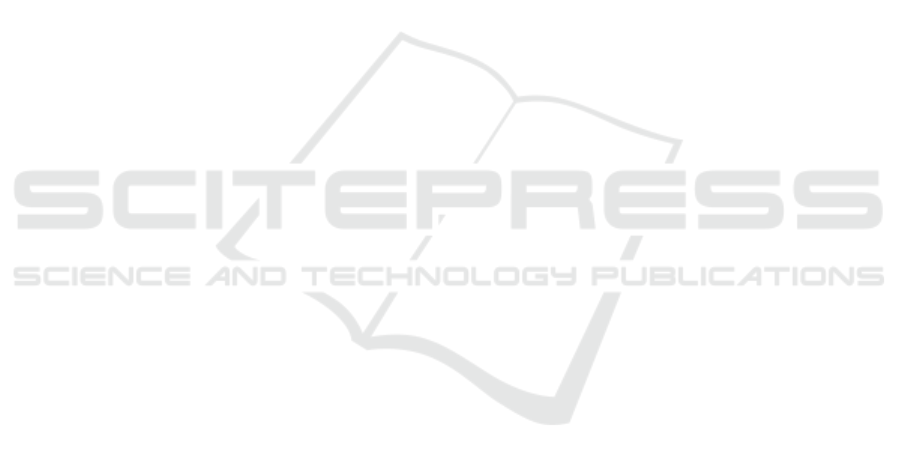
ACKNOWLEDGEMENTS
The authors express their sincere gratitude to Hildo
Bijl, University of Eindhoven, for proofreading,
and critical comments. From the Westf
¨
alische
Hochschule University of Applied Sciences, the au-
thors give thanks to Nadine Schaefer for her didac-
tic expertise and to all tutors for their practical sup-
port during the prep-courses, without which this study
would not have been possible.
REFERENCES
Akutsu, T., Mori, T., Nakamura, N., Kozawa, S., Ueno, Y.,
and Sato, T. N. (2021). Tree edit distance with vari-
ables. measuring the similarity between mathematical
formulas.
Bangert-Drowns, R. L., Kulik, C.-L. C., Kulik, J. A., and
Morgan, M. (1991). The instructional effect of feed-
back in test-like events. Review of Educational Re-
search, 61(2):213–238.
Barana, A., Marchisio, M., and Sacchet, M. (2019). Advan-
tages of Using Automatic Formative Assessment for
Learning Mathematics, pages 180–198. Springer In-
ternational Publishing.
Beevers, C. E., Cherry, B. S. G., Clark, D. E. R., Foster,
M. G., McGuire, G. R., and Renshaw, J. H. (1989).
Software tools for computer-aided learning in mathe-
matics. International Journal of Mathematical Edu-
cation in Science and Technology, 20(4):561–569.
Bevilacqua, J., Chiodini, L., Moreno Santos, I., and
Hauswirth, M. (2024). Assessing the understanding of
expressions: A qualitative study of notional-machine-
based exam questions. In Proceedings of the 24th Koli
Calling International Conference on Computing Ed-
ucation Research, Koli Calling ’24, New York, NY,
USA. Association for Computing Machinery.
Cohen, J. (1988). Statistical Power Analysis for the Behav-
ioral Sciences. Routledge.
Freire-Mor
´
an, M. (2023). Combining similarity metrics
with abstract syntax trees to gain insights into how
students program. LASI-SPAIN.
Hattie, J. and Timperley, H. (2007). The power of feedback.
Review of Educational Research, 77(1):81–112.
Higuchi, S. and Nakamura, Y. (2024). Classification of
answers in math online tests by visualizing graph
similarity. Companion Proceedings 14th Interna-
tional Conference on LearningAnalytics & Knowl-
edge (LAK24), pages 197–199.
Kirk-Johnson, A., Galla, B. M., and Fraundorf, S. H.
(2019). Perceiving effort as poor learning: The
misinterpreted-effort hypothesis of how experienced
effort and perceived learning relate to study strategy
choice. Cognitive Psychology, 115:101237.
Kluger, A. N. and DeNisi, A. (1996). The effects of
feedback interventions on performance: A histori-
cal review, a meta-analysis, and a preliminary feed-
back intervention theory. Psychological Bulletin,
119(2):254–284.
Lai, H., Wang, B., Liu, J., He, F., Zhang, C., Liu, H., and
Chen, H. (2024). Solving mathematical problems us-
ing large language models: A survey. Available at
SSRN.
Lan, A. S., Vats, D., Waters, A. E., and Baraniuk, R. G.
(2015). Mathematical language processing: Auto-
matic grading and feedback for open response math-
ematical questions. In Proceedings of the Second
(2015) ACM Conference on Learning @ Scale, L@S
2015, pages 167–176. ACM.
Levenshtein, V. I. (1966). Binary codes capable of correct-
ing deletions, insertions, and reversals. Soviet Physics
Doklady, 10(8):707–710.
Li, J. and Racine, J. S. (2008). Maxima: An open source
computer algebra system. Journal of Applied Econo-
metrics, 23(4):515–523.
Liu, W., Hu, H., Zhou, J., Ding, Y., Li, J., Zeng, J., He,
M., Chen, Q., Jiang, B., Zhou, A., and He, L. (2023).
Mathematical language models: A survey.
Macaluso, J. A., Beuford, R. R., and Fraundorf, S. H.
(2022). Familiar strategies feel fluent: The role of
study strategy familiarity in the misinterpreted-effort
model of self-regulated learning. Journal of Intelli-
gence, 10(4):83.
Mandouit, L. and Hattie, J. (2023). Revisiting “the power of
feedback” from the perspective of the learner. Learn-
ing and Instruction, 84:101718.
Narciss, S. (2004). The impact of informative tutoring
feedback and self-efficacy on motivation and achieve-
ment in concept learning. Experimental Psychology,
51(3):214–228.
Narciss, S. (2006). Informatives tutorielles Feedback:
Entwicklungs- und Evaluationsprinzipien auf der Ba-
sis instruktionspsychologischer Erkenntnisse. Wax-
mann.
Narciss, S. (2017). Conditions and effects of feedback
viewed through the lens of the interactive tutoring
feedback model. In Carless, D., Bridges, S. M., Chan,
C. K., and Glofcheski, R., editors, Scaling up assess-
ment for learning in higher education, pages 173–189.
Springer.
Narciss, S. and Zumbach, J. (2022). Formative Assessment
and Feedback Strategies, pages 1–28. Springer Inter-
national Publishing.
Neugebauer, M., Erlebach, R., Kaufmann, C., Mohr, J., and
Frochte, J. (2024). Efficient learning processes by
design: Analysis of usage patterns in differently de-
signed digital self-learning environments. In Proceed-
ings of the 16th International Conference on Com-
puter Supported Education. SCITEPRESS - Science
and Technology Publications.
Pridemore, D. R. and Klein, J. D. (1995). Control of
practice and level of feedback in computer-based in-
struction. Contemporary Educational Psychology,
20(4):444–450.
Rahaman, M. A. and Hoque, A. S. M. L. (2022). An effec-
tive evaluation system to grade programming assign-
Robust & Reliable Automated Feedback Using Tree Edit Distance for Solving Open Response Mathematical Questions
625