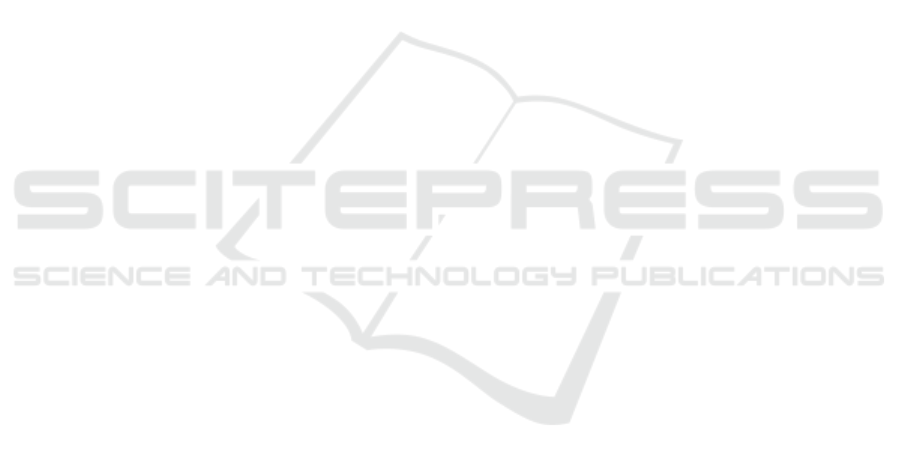
alignment. We recognize that the methodology pre-
sented in this paper is general and can be applied to
different sectors. However, it offers a valuable guide-
line for researchers and practitioners to implement
and evaluate RAG-based question-answering systems
in an educational setting.
REFERENCES
Akari Asai, Zeqiu Wu, e. a. (2023). Self-rag: Learning to
retrieve, generate, and critique through self-reflection.
https://arxiv.org/abs/2310.11511.
Barnett, S., Kurniawan, S., Thudumu, S., Brannelly, Z., and
Abdelrazek, M. (2024). Seven failure points when en-
gineering a retrieval augmented generation system.
Browning, J. G. (2024). Robot lawyers don’t have dis-
ciplinary hearings—real lawyers do: The ethical
risks and responses in using generative artificial in-
telligence. Georgia State University Law Review,
40(4):917–958.
Chen, D., Fisch, A., Weston, J., and Bordes, A. (2017).
Reading wikipedia to answer open-domain questions.
ChromaDB (2024). Chromadb: Open-source vector
database for ai applications, version 0.6.3. Accessed:
2024-01-07.
Crompton, H. and Burke, D. (2023). Artificial intelligence
in higher education: the state of the field. Interna-
tional Journal of Educational Technology in Higher
Education, 20(1):22.
D-Star-AI (2025). dsrag: An implementation of retrieval-
augmented generation (rag). Accessed: 2025-01-09.
Dakshit, S. (2024). Faculty perspectives on the potential of
rag in computer science higher education.
Damiano, A. D., Laur
´
ıa, E. J., Sarmiento, C., and Zhao,
N. (2024). Early perceptions of teaching and learning
using generative ai in higher education. Journal of
Educational Technology Systems, 52(3):346–375.
Edge, D., Trinh, H., Cheng, N., Bradley, J., Chao, A., Mody,
A., Truitt, S., and Larson, J. (2024). From local to
global: A graph rag approach to query-focused sum-
marization.
Es, S., James, J., Espinosa-Anke, L., and Schockaert, S.
(2023). Ragas: Automated evaluation of retrieval aug-
mented generation.
Feldman, P., Foulds, J. R., and Pan, S. (2024).
Ragged edges: The double-edged sword of retrieval-
augmented chatbots.
Gonzalez-Bonorino, A., Laur
´
ıa, E. J. M., and Presutti,
E. (2022). Implementing open-domain question-
answering in a college setting: An end-to-end method-
ology and a preliminary exploration. In Proceedings
of the 14th International Conference on Computer
Supported Education - Volume 2: CSEDU, pages 66–
75. INSTICC, SciTePress.
Guu, K., Lee, K., Tung, Z., Pasupat, P., and Chang, M.-W.
(2020). Realm: Retrieval-augmented language model
pre-training.
Karpukhin, V., O
˘
guz, B., Min, S., Lewis, P., Wu, L.,
Edunov, S., Chen, D., and tau Yih, W. (2020). Dense
passage retrieval for open-domain question answer-
ing.
LangChain (2024). Langchain: Building applications with
llms through composability. Accessed: 2024-01-07.
Lewis, P., Perez, E., Piktus, A., Petroni, F., Karpukhin,
V., Goyal, N., K
¨
uttler, H., Lewis, M., tau
Yih, W., Rockt
¨
aschel, T., Riedel, S., and Kiela,
D. (2020). Retrieval-augmented generation for
knowledge-intensive nlp tasks. In Advances in Neu-
ral Information Processing Systems, volume 33, pages
9459–9474.
Lyu, W., Wang, Y., Chung, T. R., Sun, Y., and Zhang, Y.
(2024). Evaluating the effectiveness of llms in intro-
ductory computer science education: A semester-long
field study. In Proceedings of the Eleventh ACM Con-
ference on Learning @ Scale, L@S ’24, page 63–74.
ACM.
Modran, H., Bogdan, I. C., Ursut
,
iu, D., Samoila, C., and
Modran, P. L. (2024). Llm intelligent agent tutor-
ing in higher education courses using a rag approach.
Preprints.
OpenAI (2024). Openai: Advancing ai for everyone. Ac-
cessed: 2024-01-07.
RAGAS (2024). Ragas: Robust evaluation for retrieval-
augmented generation systems. Accessed: 2024-01-
07.
Sanniboina, S., Trivedi, S., and Vijayaraghavan, S. (2024).
Lore: Logit-ranked retriever ensemble for enhancing
open-domain question answering.
Shi-Qi Yan, Jia-Chen Gu, e. (2024). Corrective re-
trieval augmented generation. https://arxiv.org/abs/
2401.15884.
Thway, M., Recatala-Gomez, J., Lim, F. S., Hippalgaonkar,
K., and Ng, L. W. T. (2024). Battling botpoop using
genai for higher education: A study of a retrieval aug-
mented generation chatbots impact on learning.
Th
¨
us, D., Malone, S., and Br
¨
unken, R. (2024). Explor-
ing generative ai in higher education: a rag system to
enhance student engagement with scientific literature.
Frontiers in Psychology, 15:1474892.
Wong, L. (2024). Gaita: A rag system for personalized
computer science education.
Wu, K., Wu, E., Cassasola, A., Zhang, A., Wei, K., Nguyen,
T., Riantawan, S., Riantawan, P. S., Ho, D. E., and
Zou, J. (2024). How well do llms cite relevant medical
references? an evaluation framework and analyses.
Yu, H., Gan, A., Zhang, K., Tong, S., Liu, Q., and Liu, Z.
(2024). Evaluation of retrieval-augmented generation:
A survey. https://arxiv.org/abs/2405.07437.
Yunfan Gao, Yun Xiong, e. a. (2024). Retrieval-augmented
generation for large language models: A survey. https:
//arxiv.org/abs/2312.10997.
CSEDU 2025 - 17th International Conference on Computer Supported Education
428