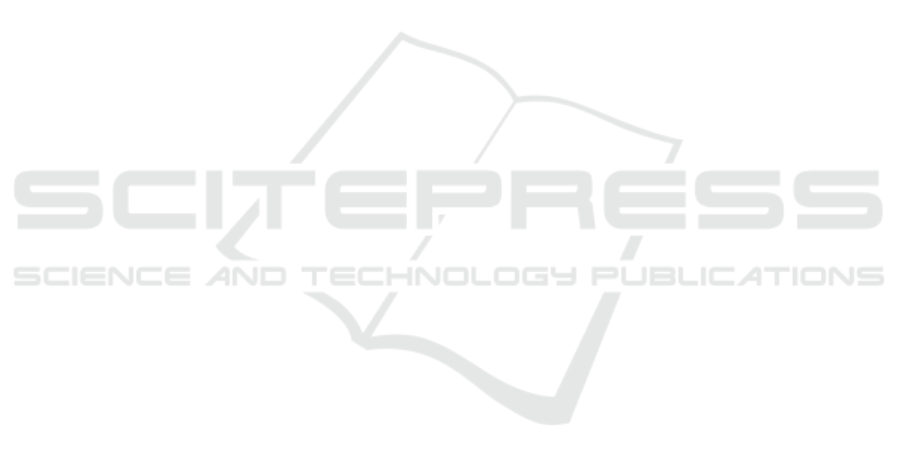
Lozynskyi, V. (2024). Improving the risk management
process in quality management systems of higher ed-
ucation. Dental science reports.
Casta
˜
neda, L. and Gourlay, L. (2023). Data ecosystems in
education: opportunities and challenges.
Chu, S. and Wang, Y. (2023). Application of data man-
agement capability maturity assessment model in the
automotive industry.
Curry, E., Auer, S., Berre, A. J., Metzger, A., Perez, M. S.,
and Zillner, S. (2022). Technologies and applications
for big data value. In Technologies and Applications
for Big Data Value, pages 1–15. Springer.
Dur
˜
ao, L. F. C., Zancul, E., and Sch
¨
utzer, K. (2024). Digi-
tal twin data architecture for product-service systems.
Procedia CIRP, 121:79–84.
Feng, Y., Chen, W., Zhang, Y., Yu, G., Liu, Y., and Xu, H.
(2021). Research on the architecture of smart cam-
pus system based on data middleground in univer-
sity. In 2021 IEEE 3rd International Conference on
Computer Science and Educational Informatization
(CSEI), pages 18–21. IEEE.
Haneem, F., Kama, N., Taskin, N., Pauleen, D., and Bakar,
N. A. A. (2019). Determinants of master data manage-
ment adoption by local government organizations: An
empirical study. International Journal of Information
Management, 45:25–43.
Hendrawan, F. R., Kusumasari, T. F., and Praditya, D.
(2023). (2) a comprehensive framework of role data
governance in ensuring data quality: Literature re-
view.
Henninger, A. (2022). Data Governance. Enablers, In-
hibitors, Practices, and Outcomes. PhD thesis,
Toronto Metropolitan University.
Hillman, V. and Ganesh, V. (2019). Kratos: A secure, au-
thenticated and publicly verifiable system for educa-
tional data using the blockchain. In 2019 IEEE Inter-
national Conference on Big Data (Big Data), pages
5754–5762. IEEE.
Huang, R. (2023). Research on technical path and practice
of digital transformation of colleges based on ipaas.
In Second International Conference on Digital Society
and Intelligent Systems (DSInS 2022), volume 12599,
pages 269–275. SPIE.
Inep (2023a). Censo escolar da educac¸
˜
ao b
´
asica 2023: Re-
sumo t
´
ecnico. Accessed on January 20, 2025.
Inep (2023b). Mec e inep divulgam resultados do censo
escolar 2023. Accessed on January 20, 2025.
International, D. (2017). DAMA-DMBOK: Data manage-
ment body of knowledge. Technics Publications, LLC.
ISACA (2018). COBIT® 2019 Framework: Introduction
and Methodology. ISACA.
Kawtrakul, A., Chanlekha, H., Waiyamai, K., Kangka-
chit, T., d’Orazio, L., Kotzinos, D., Laurent, D., and
Spyratos, N. (2021). Towards data-and-innovation
driven sustainable and productive agriculture: Bio-
agri-watch as a use case study. In 2021 IEEE Inter-
national Conference on Big Data (Big Data), pages
3407–3415. IEEE.
Kshirsagar, O. R. (2024). Big data analytics: Future of
database. International Journal for Research in Ap-
plied Science and Engineering Technology.
Kush, R. D., Warzel, D., Kush, M. A., Sherman, A.,
Navarro, E. A., Fitzmartin, R., P
´
etavy, F., Galvez, J.,
Becnel, L. B., Zhou, F., et al. (2020). Fair data sharing:
the roles of common data elements and harmoniza-
tion. Journal of biomedical informatics, 107:103421.
Li, W. and Quinn, P. (2024). The european health data
space: An expanded right to data portability? Com-
puter Law & Security Review, 52:105913.
Miao, Z., Zhai, J., Lin, Y., and Yuan, C. (2019). A compar-
ative study of open education data between china and
uk. In 2019 16th International Conference on Service
Systems and Service Management (ICSSSM), pages 1–
5. IEEE.
Mojun, X. and Tingmin, L. (2022). Architecture optimiza-
tion and core code de-redundancy of educational man-
agement information system in higher vocational col-
leges under the background of data governance algo-
rithm. In 2022 4th International Conference on Inven-
tive Research in Computing Applications (ICIRCA),
pages 532–535. IEEE.
Otoo-Arthur, D. and van Zyl, T. L. (2020). A scalable
heterogeneous big data framework for e-learning sys-
tems. In 2020 international conference on artificial
intelligence, big data, computing and data communi-
cation systems (icABCD), pages 1–15. IEEE.
Rani, P. S., Janani, J., Krithika, C., Keerthika, S. L., and
Joshika, J. (2023). Blockchain-based file tracking
and data management system for education sector. In
2023 International Conference on Advances in Com-
puting, Communication and Applied Informatics (AC-
CAI), pages 1–6. IEEE.
Soares, S. (2010). The IBM data governance unified pro-
cess: driving business value with IBM software and
best practices. MC Press, LLC.
Stahl, B., H
¨
ackel, B., Leuthe, D., and Ritter, C. (2023).
Data or business first?—manufacturers’ transforma-
tion toward data-driven business models. Schmalen-
bach Journal of Business Research, 75(3):303–343.
Volkov, A., Otbetkina, T., and Vidmanova, A. (2023). Im-
proving the effectiveness of data-driven learning man-
agement. International journal of social science and
human research.
Wang, Z. and Huang, R. (2021). Research on the implemen-
tation path and practice of data driven university gov-
ernance modernization—taking shandong youth col-
lege of political science as an example. In 2021 11th
International Conference on Information Technology
in Medicine and Education (ITME), pages 500–504.
IEEE.
Williamson, B. (2018). The hidden architecture of higher
education: Building a big data infrastructure for the
‘smarter university’. International Journal of Educa-
tional Technology in Higher Education, 15:1–26.
Zanti, S., Berkowitz, E., Katz, M., Nelson, A. H., Burnett,
T., Culhane, D., and Zhou, Y. (2022). Leveraging in-
tegrated data for program evaluation: Recommenda-
tions from the field. Evaluation and Program Plan-
ning, 95:102093.
ICEIS 2025 - 27th International Conference on Enterprise Information Systems
374