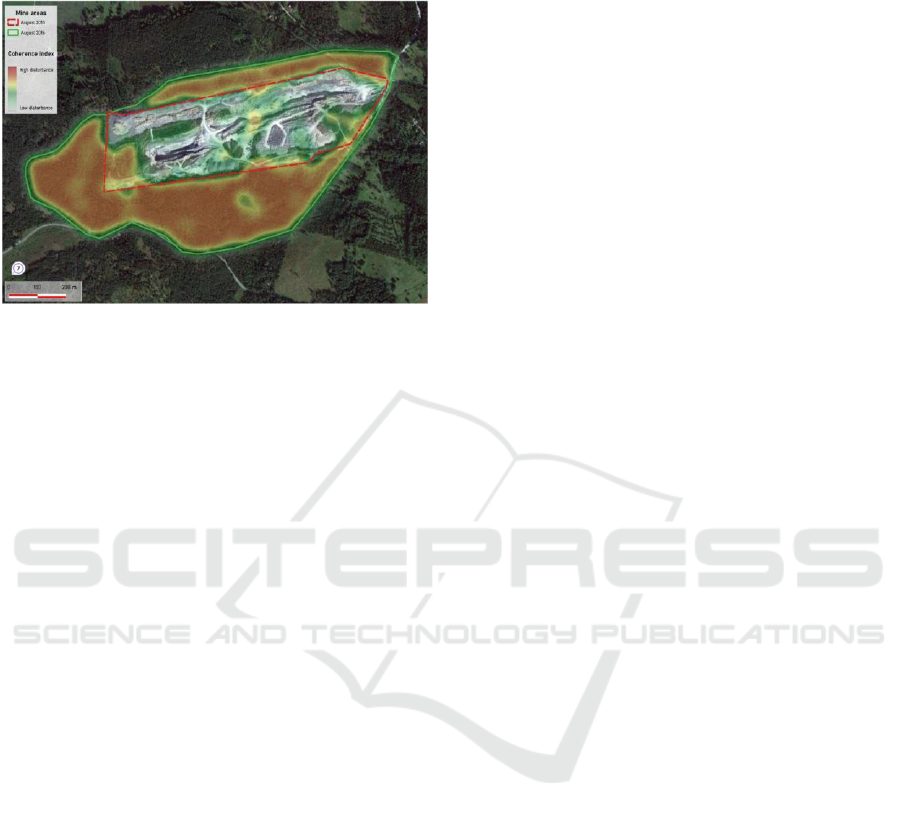
Sentinel 2A images and relevant background
information about the Aijala site.
Figure 11: NDCI depicting ongoing high disturbances in the
Aijala post-closure site between 2015-2017.
5 CONCLUSIONS
In this work, we present the preliminary results of the
S34I HORIZON project. At the moment, the methods
are being validated and verified in the pilots and also
with the end-users.
Based on the methods developed under the scope
of S34I, we prototyped EO-based services that cater
to the specific needs of mining stakeholders. These
services aim to address three key areas:
1. RM Deposits Mapping: This involves using
EO data to identify and map potential mineral
deposits, both on land and in shallow waters.
2. Early Warnings: The focus here is on
developing EO-based systems that can provide early
warnings of potential hazards at mining sites, such as
ground instability.
3. Environmental Monitoring: This
encompasses the use of EO data to monitor the
environmental impact of mining activities, such as the
detection of AMD.
In the future, these services will be available for
the stakeholders and end-users.
The result of this project will be an important step
forward in monitoring all phases of the mining cycle
using EO data, contributing to more sustainable
mining practices.
ACKNOWLEDGEMENTS
This study is funded by the European Union under
grant agreement no. 101091616
(https://doi.org/10.3030/101091616), project S34I –
Secure and Sustainable Supply of Raw Materials for
EU Industry.
REFERENCES
Beiranvand Pour, A., Park, T.-Y. S., Park, Y., Hong, J. K.,
Zoheir, B., Pradhan, B., Ayoobi, I., & Hashim, M.
(2018). Application of Multi-Sensor Satellite Data for
Exploration of Zn–Pb Sulfide Mineralization in the
Franklinian Basin, North Greenland. Remote
Sensing, 10(8), 1186.
https://doi.org/10.3390/rs10081186.
Cardoso-Fernandes, J. (2024). S34I. D4.1: Catalogue of
EO-based Prototyped Services for CRM and non-CRM
Deposits Mapping. S34I Horizon Europe GA
101091616. Available at:
https://cordis.europa.eu/project/id/101091616/results
(accessed on 15 January 2025).
Carvalho, M., Cardoso-Fernandes, J., González, F. J., &
Teodoro, A. C. (2025). Comparative Performance of
Sentinel-2 and Landsat-9 Data for Raw Materials'
Exploration Onshore and in Coastal Areas. Remote
Sensing, 17(2). doi: 10.3390/rs17020305.
Chen, L., Xie, Y., Wang, Y., Ge, S., Wang, F.Y (2024).
Sustainable Mining in the Era of Artificial Intelligence.
IEEE/CAA Journal of Automatica Sinica, 11(1), 1-4,
doi.org/10.1109/JAS.2023.124182.
European Commission (2023). European Critical Raw
Materials Act. European Commission - Press release.
https://ec.europa.eu/commission/presscorner/detail/en/
ip_23_1661. Accessed 14 January 2025.
Farahnakian, F., Luodes, N., & Karlsson, T. (2024).
Machine Learning Algorithms for Acid Mine Drainage
Mapping Using Sentinel-2 and Worldview-3. Remote
Sensing, 16(24), 4680.
https://doi.org/10.3390/rs16244680.
Gulicovski, J., Stojmenović, M., Rosić, M., Vasić, A.,
Ristović, I., Janković-Častvan, I., & Kragović, M.
(2024). Mineralogical Characterization of the Grot
Lead and Zinc Mine Tailings from Aspects of Their
Further Use as Raw Material. Applied Sciences, 14(3),
1167. https://doi.org/10.3390/app14031167.
Hool, A., Helbig, C., Wierink, G. (2024). Challenges and
opportunities of the European Critical Raw Materials
Act. Mineral Economics, 37(3), 661-668,
doi.org/10.1007/s13563-023-00394-y.
Laurence, D. (2011). Establishing a sustainable mining
operation: an overview. Journal of Cleaner Production,
19(2), 278-284, doi.org/10.1016/j.jclepro.2010.08.019.
Li, S., Wang, R., Wang, L., Liu, S., Ye, J., Xu, H., & Niu,
R. (2023). An Approach for Monitoring Shallow
Surface Outcrop Mining Activities Based on
Multisource Satellite Remote Sensing Data. Remote
Sensing, 15(16), 4062.
https://doi.org/10.3390/rs15164062.
Oštir, K. et al. (2024). S34I. D3.3: Catalogue of Innovative
New Methods to Analyze Earth Observation Data. S34I
S34I 2025 - Special Session on S34I - From the Sky to the Soil
284