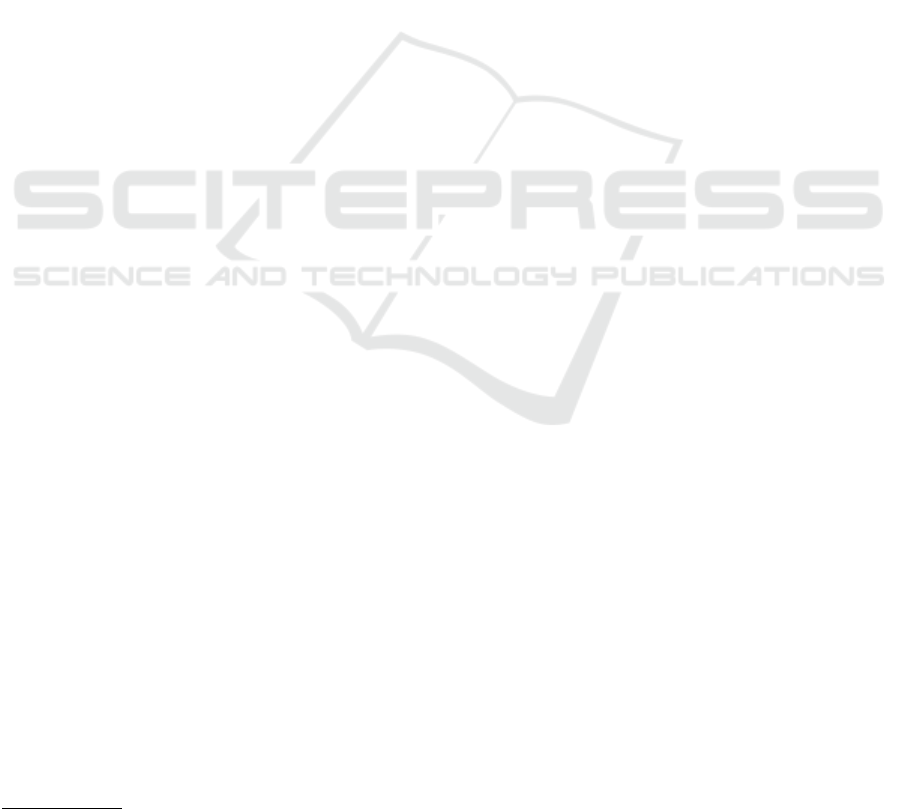
6 CONCLUSION
This paper presents a four-phase design science re-
search approach to explore how explainability re-
quirements can be documented and elicited. In the
related work 18 articles have been reviewed and an-
alyzed, revealing the importance of detailed docu-
mentation and visual diagrams to cater to different
stakeholder preferences. A sentence template for doc-
umenting these requirements is proposed, which is
combined with a scenario method for elicitation. Ex-
pert interviews confirm the usefulness of this com-
bined method. Additionally, the process includes re-
fined elicitation techniques and provides a one-page
information sheet to capture details. This research is
specifically evaluated in the context of a surgery assis-
tance system, showing it improves the completeness
and detail of the SRS. Future work could focus on
integrating this workflow into existing RE processes
and creating a tutorial video for practitioners.
APPENDIX
Additional research data is available as supplemen-
tary material at EU’s Zenodo portal
7
.
It contains (i) The existing requirements and our
classification for explainability requirements. (ii) Ad-
ditional tables and figures and guidance information.
(iii) The artifacts of the first design science iteration.
(iv) The created scenarios.
REFERENCES
Afzal, S., Chaudhary, A., Gupta, N., Patel, H., Spina, C.,
and Wang, D. (2021). Data-debugging through in-
teractive visual explanations. In Trends a. Appl. in
Knowl. Disc. a. Data Mining, Delhi, India, pages 133–
142. Springer.
Ahmad, K., Abdelrazek, M., Arora, C., Bano, M., and
Grundy, J. (2023). Requirements engineering for ar-
tificial intelligence systems: A systematic mapping
study. Information a. Software Techn.
Alonso, J. M., Ducange, P., Pecori, R., and Vilas, R. (2020).
Building explanations for fuzzy decision trees with the
expliclas software. In 2020 IEEE Int. Conf. on Fuzzy
Systems, pages 1–8. IEEE.
Balasubramaniam, N., Kauppinen, M., Rannisto, A.,
Hiekkanen, K., and Kujala, S. (2023). Transparency
and explainability of ai systems: From ethical guide-
lines to requirements. Information and Software Tech-
nology, 159:107197.
7
https://zenodo.org/doi/10.5281/zenodo.10854274
Brunotte, W., Chazette, L., Klös, V., and Speith, T. (2022).
Quo vadis, explainability?–a research roadmap for ex-
plainability engineering. In International Working
Conference on Requirements Engineering: Founda-
tion for Software Quality, pages 26–32. Springer.
Calegari, R. and Sabbatini, F. (2022). The psyke technology
for trustworthy artificial intelligence. In Int. Conf. of
the Italian Assoc. for AI, pages 3–16. Springer.
Cepeda Zapata, K. A., Ward, T., Loughran, R., and McCaf-
fery, F. (2022). Challenges associated with the adop-
tion of artificial intelligence in medical device soft-
ware. In Irish Conf. on AI and Cognitive Science,
pages 163–174. Springer.
Chazette, L., Brunotte, W., and Speith, T. (2021). Exploring
explainability: A definition, a model, and a knowledge
catalogue. In 2021 IEEE 29th International Require-
ments Engineering Conference (RE), pages 197–208.
Chazette, L., Karras, O., and Schneider, K. (2019). Do end-
users want explanations? analyzing the role of ex-
plainability as an emerging aspect of non-functional
requirements. In 27th RE Conf., pages 223–233.
IEEE.
Chazette, L., Klös, V., Herzog, F., and Schneider, K. (2022).
Requirements on explanations: A quality framework
for explainability. In 30th Int.RE Conf., pages 140–
152.
Chazette, L. and Schneider, K. (2020). Explainability as
a non-functional requirement: challenges and recom-
mendations. Requirements Engineering, 25:493–514.
Cirqueira, D., Nedbal, D., Helfert, M., and Bezbradica, M.
(2020). Scenario-based requirements elicitation for
user-centric explainable ai: A case in fraud detection.
In Int. cross-domain conf. for ML a. knowledge ex-
tract., pages 321–341. Springer.
Duque Anton, S. D., Schneider, D., and Schotten, H. D.
(2022). On explainability in ai-solutions: A cross-
domain survey. In Computer Safety, Reliability, and
Security. SAFECOMP Workshops, pages 235–246,
Cham. Springer Int. Pub.
Ebert, C. (2022). Systematisches Requirements En-
gineering:Anforderungen ermitteln, dokumentieren,
analysieren u.verwalten. dpunkt verlag.
Habiba, U.-E., Bogner, J., and Wagner, S. (2022). Can re-
quirements engineering support explainable artificial
intelligence? towards a user-centric approach for ex-
plainability requirements. In 2022 IEEE 30th Inter-
national Requirements Engineering Conference Work-
shops (REW), pages 162–165. IEEE.
Habibullah, K. M., Gay, G., and Horkoff, J. (2022). Non-
functional requirements for machine learning: An ex-
ploration of system scope and interest. In Proceedings
of the 1st Workshop on Software Engineering for Re-
sponsible AI, pages 29–36.
Hevner, A., Ram, S., March, S., and Park, J. (2004). Design
science in information systems research. MIS Quar-
terly, 28:75–105.
Junger, D., Hirt, B., and Burgert, O. (2022). Concept
and basic framework prototype for a flexible and
intervention-independent situation recognition system
in the or. Computer Methods in Biomechanics and
Elicitation and Documentation of Explainability Requirements in a Medical Information Systems Context
93