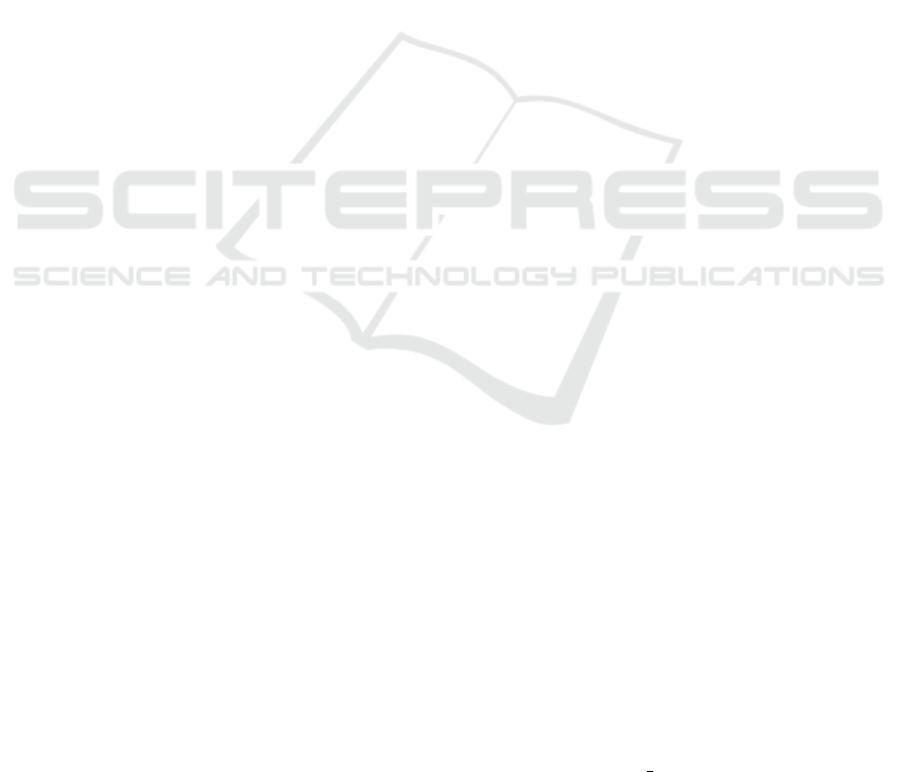
Andel, S. A., De Vreede, T., Spector, P. E., Padmanabhan,
B., Singh, V. K., & De Vreede, G.-J. (2020-12). Do
social features help in video-centric online learning
platforms? A social presence perspective. Computers
in Human Behavior, 113, 106505. https://doi.org/10.
1016/j.chb.2020.106505
Baumann, N. (2022). Zur Wahrnehmung von Online-
Mentoring : Eine qualitative Studie zu den subjektiven
Sichtweisen von Mentorinnen, Mentoren und Mentees.
https://doi.org/10.20378/irb-57500
Bay, B. (2018-11-02). Bloom’s Taxonomy and Depth of
Knowledge (DOK) - Rethinking Learning. Retrieved
2024-03-01, from https://barbarabray.net/2018/11/02/
blooms-taxonomy-and-depth-of-knowledge-dok/
Behnke, J., & Behnke, N. (2006). Grundlagen der statis-
tischen Datenanalyse: eine Einf
¨
uhrung f
¨
ur Politikwis-
senschaftler. VS.
Besa, K., Kochsk
¨
amper, D., Lips, A., Schr
¨
oer, W.,
& Thomas, S. (2022-10-19). Stu diCo III -
Hochschulzukunft gestalten – aus den (digitalen)
Corona-Semestern lernen. https://doi.org/10.18442/
219
Bicak, B. E., Borchert, C., & H
¨
oner, K. (2023-12). Foster-
ing scientific reasoning with inquiry problem-solving
experiments in an organic chemistry lab course.
CHEMKON, 30(8), 326–333. https://doi.org/10.1002/
ckon.202200024
Biggs, J. B., & Tang, C. S.-k. (2011). Teaching for quality
learning at university: What the student does (4. ed).
McGraw-Hill, Society for Research into Higher Edu-
cation & Open University Press.
OCLC: 838444823.
Blackwell, A. F. (Ed.). (2001). Thinking with Diagrams.
Springer Netherlands. https://doi.org/10.1007/978-
94-017-3524-7
Bortz, J., & Schuster, C. (2010). Statistik f
¨
ur Human- und
Sozialwissenschaftler (7., vollst.
¨
uberarb. und erw.
Aufl). Springer.
Chappel, L. (2018-01-31). Wireshark® 101: Einf
¨
uhrung
in die Protokollanalyse - Deutsche Ausgabe
(22018th ed.). mitp.
Chrobak, A. M. (2004-04). Entwicklung von Online-
Trainererfolg in der betrieblichen Weiterbildung
durch die F
¨
orderung von Online-Selbstwirksamkeit, -
Strategie und Koh
¨
asion.
Cohen, J. (1988-07-01). Statistical Power Analysis for the
Behavioral Sciences (Subsequent Edition). Routledge
Member of the Taylor and Francis Group.
Cross, N. (2023). Design thinking: Understanding how de-
signers think and work (Second edition). Bloomsbury
Visual Arts.
Decius, J., Dannowsky, J., & Schaper, N. (2021-12-03).
Die Studierenden im Mittelpunkt: Personale Unter-
schiede beim informellen und formalen Lernen in
Zeiten virtueller Lehre. In I. Neiske, J. Osthushenrich,
N. Schaper, U. Trier, & N. V
¨
oing (Eds.), Hochschul-
bildung: Lehre und Forschung (1st ed., pp. 89–114,
Vol. 3). transcript Verlag. https://doi.org/10.14361/
9783839456903-008
Erpenbeck, J., Sauter, S., & Sauter, W. (2015). E-Learning
und Blended Learning. Selbstgesteuerte Lernprozesse
zum Wissensaufbau und zur Qualifizierung. Springer
Gabler.
Fiedler, M., Kaliske, J., & K
¨
astner, M. (2022-07-05).
Studieren 2.0 - Pr
¨
asenz, Digital oder Hybrid? Lessons
Learned, 2(1). https://doi.org/10.25369/ll.v2i1.34
Field, A., Miles, J., & Field, Z. (2012-04-05). Discovering
Statistics Using R (1st ed.). SAGE Publications Ltd.
Graham, C. R. (2006). Blended learning systems. The hand-
book of blended learning: Global perspectives, local
designs, 1, 3–21.
Gr
¨
uner, C. (2018-02). Das Ph
¨
anomen Lurking. In-
dividuelle Lernprozesse
”
aktiver“ und
”
passiver“
Nutzer*innen virtueller Lernumgebungen im Fern-
studium am Beispiel der FernUniversit
¨
at in Hagen.
Hein, M., Ladner, R., & Scholz, U. (2021). Wireshark
- Standard-Tool zur Analyse von Netzwerken: Prax-
iswissen: Netzwerkprotokolle aufzeichnen, darstellen
und analysieren. Independently published.
Heinzel, A., Mottaghy, F. M., Brenner, W., & Marienhagen,
J. (2020-02). Statuserhebung der studentischen Lehre
f
¨
ur das Fach Nuklearmedizin in Deutschland. Nuk-
learmedizin - NuclearMedicine, 59(01), 38–46. https:
//doi.org/10.1055/a-1011-3481
Hurzlmeier, M., Watzka, B., Hoyer, C., Girwidz, R., &
Ertl, B. (2024-01). Lernergebnisse und individuelle
Prozesse des Physik-Lernens mit auditiven und vi-
suellen Hinweisen. Zeitschrift f
¨
ur P
¨
adagogische Psy-
chologie, 38(1-2), 99–116. https://doi.org/10.1024/
1010-0652/a000331
Hußner, I., Lazarides, R., & Westphal, A. (2022-10-01).
COVID-19-bedingte Online- vs. Pr
¨
asenzlehre: Dif-
ferentielle Entwicklungsverl
¨
aufe von Beanspruchung
und Selbstwirksamkeit in der Lehrkr
¨
aftebildung?
Zeitschrift f
¨
ur Erziehungswissenschaft, 25(5), 1243–
1266. https://doi.org/10.1007/s11618-022-01072-5
Jagoe, C. (2014-01-28). Open Everything Exams. @col-
injagoe.com. Retrieved 2024-08-14, from https : / /
colinjagoe.com/2014/01/28/open-everything-exams/
Ketter, V., Brauch, C., Elser, P., Freier, I., & Roob,
Y. (2022-05-02). Online-LEERprozesse? Von den
Bildungs(un)m
¨
oglichkeiten des digitalen Studierens
aus Lehrenden- und Studierendenperspektive: Ein
Dilemma in drei Akten. Ludwigsburger Beitr
¨
age zur
Medienp
¨
adagogik, 22, 1–16. https://doi.org/10.21240/
lbzm/22/11
Klug, J., & Seethaler, E. (2021-11-30). (Un)Freiwillig
Online-Lehre. Wie Lehramtsstudierende in unter-
schiedlichen Lernsettings Lerngewinn, Motivation
und emotionales Befinden einsch
¨
atzen. Ludwigs-
burger Beitr
¨
age zur Medienp
¨
adagogik, 21, 1–16.
https://doi.org/10.21240/lbzm/21/05
Kossack, F., & Bender, B. (2022). Heterogeneous groups
of students as a challenge in engineering design edu-
cation. DS 119: Proceedings of the 33rd Symposium
Design for X (DFX2022), 10–10. https://doi.org/10.
35199/dfx2022.07
Krathwohl, D. R. (2002-11-01). A Revision of Bloom’s
Taxonomy: An Overview. Theory Into Practice,
41(4), 212–218. https : / / doi . org / 10 . 1207 /
s15430421tip4104
2
Kuhn, S., Huettl, F., Deutsch, K., Kirchg
¨
assner, E., Huber,
T., & Kneist, W. (2021-02). Chirurgische Ausbildung
CSEDU 2025 - 17th International Conference on Computer Supported Education
904