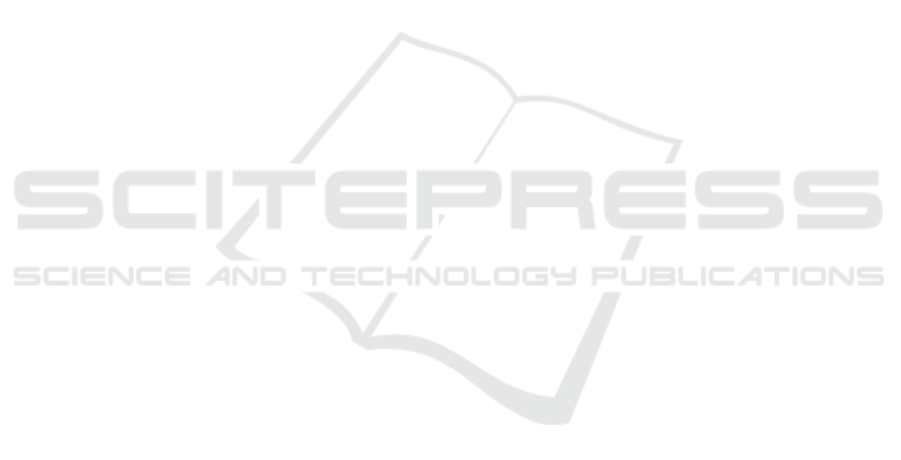
chitecture and components of the system, describing
their interactions. Section V focuses on the imple-
mentation, explaining how the proposed design was
realized in practice. Section VI discusses the algo-
rithm evaluation to ensure the functionality, reliabil-
ity, and performance of the developed system. Fi-
nally, Section VII presents the conclusions, summa-
rizing the main findings and potential directions for
future work.
2 STATE OF THE ART
Public data sets play an essential role in traffic man-
agement systems, offering reliable and standardized
information for large-scale analysis. The European
Union has established a legal framework that pro-
motes the reuse of public sector information, recog-
nizing its potential to boost the economy and innova-
tion. Directive (EU) 2019/1024 on open data and the
re-use of public sector information requires Member
States to provide access to data, such as geographical,
cadastral, statistical, or legal information, in open and
machine-readable formats, ensuring its accessibility
and re-use (eu-, 2019).
In the area of traffic, the European Union has im-
plemented specific measures to ensure the availability
of essential data. Commission Delegated Regulation
(EU) 2015/962 requires Member States to improve
the accessibility, exchange, and updating of road and
traffic infrastructure data necessary for the provision
of real-time traffic information services across the
Union (eu-, 2015).
To centralise and facilitate access to this informa-
tion, National Access Points (NAPs) have been cre-
ated. The NAPs collect data provided by the traf-
fic management entities of the national territory, in-
cluding road authorities, infrastructure operators and
service providers (nap, 2016). One notable exam-
ple is the Dutch National Data Warehouse (NDW),
which aggregates data from traffic sensors, cameras,
and monitoring stations throughout the Netherlands.
NDW datasets, standardized under frameworks like
Data Exange V2 (DATEX II), ensure consistency and
reliability, providing critical inputs for real-time and
historical traffic analysis (II, 2019). These data are
particularly valuable for public systems focused on
large-scale traffic planning and management.
DATEX II, a standard for traffic information ex-
change throughout Europe, provides a unified lan-
guage for traffic systems, ensuring interoperability
among diverse platforms. By integrating real-time
data from multiple sources, such as sensors and mon-
itoring centers, DATEX II facilitates robust solutions
for incident detection, congestion monitoring, and
itinerary optimization (for Standardization, 2019). Its
adaptability has made it essential for the management
of urban and interurban traffic.
On the other hand, private companies such as
Google, Apple, and Waze have developed proprietary
systems that focus on real-time navigation and traffic
updates. These platforms rely heavily on their own
data ecosystems, including user-generated reports,
satellite imagery, and historical traffic patterns ob-
tained through their users. For example, Waze lever-
ages community input to identify incidents, while
Google Maps combines live traffic data with predic-
tive algorithms to recommend the optimal itinerary
(Inc., 2022). However, these proprietary systems
are often optimized for commercial goals, and their
datasets may not align with the regulated and val-
idated information provided by public authorities.
This divergence can result in quality discrepancies,
particularly in regions where public data sets are more
comprehensive (Smith and Taylor, 2021).
Private platforms excel at leveraging hyperlocal-
ized data, thanks to their widespread user bases and
advanced algorithms. Google Maps, for instance, in-
tegrates real-time movement data from millions of
users to predict congestion patterns, while Waze in-
corporates live incident reports to improve accuracy.
These approaches provide highly detailed traffic in-
formation, particularly in urban environments with
high user engagement. However, their dependence on
decentralized user contributions and historical traffic
data introduces limitations in high-demand, dynami-
cally changing environments such as logistics hubs or
port access roads.
One of the main weaknesses of private naviga-
tion systems is their limited ability to optimize traffic
flow on a network-wide scale. These systems prior-
itize individual travel-time minimization, often lead-
ing to suboptimal congestion management. A com-
mon issue is the re-routing of vehicles to alterna-
tive roads without considering their overall traffic ab-
sorption capacity, which can unintentionally generate
new congestion points rather than alleviating existing
ones. Moreover, since these systems are primarily
based on reactive mechanisms, their response time to
unforeseen disruptions, such as accidents or sudden
road closures, is inherently delayed, making them less
suitable for critical infrastructure management (Jalota
et al., 2021). Bridging the gap between these two
paradigms remains a significant challenge. The inte-
gration of public standards like DATEX II and NDW
with the rich, dynamic data sets of private platforms
offers the potential to create more accurate and scal-
able traffic management solutions (Brown and Jones,
VEHITS 2025 - 11th International Conference on Vehicle Technology and Intelligent Transport Systems
646