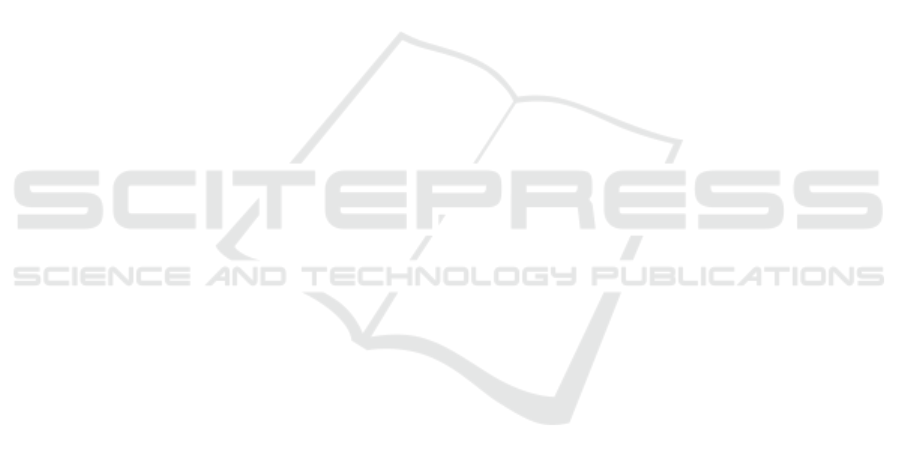
proposed evaluation could involve a case study to test
the personas’ utility in studying mentorship dynamics
in online communities. We also intend to explore how
these personas could guide study designs, such as cre-
ating tailored interview guides or targeted surveys.
6 CONCLUSION
Our work demonstrates the potential of data-driven
personas as a methodological tool for SE research.
The main contributions of this paper are (1) the
methodology for developing data-driven personas,
applied in the context of SE research, and (2) the
accompanying practical insights and lessons learned
from this application.
By segmenting behavioral patterns from survey
data, we created personas that capture distinct moti-
vations, preferences, and expertise levels within the
StackOverflow community. Key methodological in-
sights include refining data for realism, balancing de-
tail with generality, and integrating quantitative find-
ings with practical applications. Limitations still ex-
ist, for example the reliance on the availability of suf-
ficient good quality data.
Personas provide a human-centered approach to
SE research, guiding the design of tailored instru-
ments like interview guides and validating study sce-
narios. Ultimately, data-driven personas can help
bridge theoretical research with real-world behavior,
providing a structured framework for exploring the
human aspects of software development.
REFERENCES
Billestrup, J., Stage, J., Bruun, A., Nielsen, L., and Nielsen,
K. S. (2014). Creating and using personas in software
development: experiences from practice. In Human-
Centered Software Engineering: 5th IFIP WG 13.2 Inter-
national Conference, HCSE 2014, Paderborn, Germany,
September 16-18, 2014. Proceedings 5, pages 251–258.
Springer.
Chapman, C. N. and Milham, R. P. (2006). The personas’
new clothes: methodological and practical arguments
against a popular method. In Proceedings of the hu-
man factors and ergonomics society annual meeting, vol-
ume 50, pages 634–636. SAGE Publications Sage CA:
Los Angeles, CA.
Chua, A. Y. and Banerjee, S. (2015). Answers or no an-
swers: Studying question answerability in stack over-
flow. Journal of Information Science, 41(5):720–731.
Faily, S. and Lyle, J. (2013). Guidelines for integrating per-
sonas into software engineering tools. In Proceedings of
the 5th ACM SIGCHI symposium on Engineering inter-
active computing systems, pages 69–74.
Ford, D., Zimmermann, T., Bird, C., and Nagappan,
N. (2017). Characterizing software engineering work
with personas based on knowledge worker actions. In
2017 ACM/IEEE International Symposium on Empirical
Software Engineering and Measurement (ESEM), pages
394–403. IEEE.
Junior, P. T. A. and Filgueiras, L. V. L. (2005). User mod-
eling with personas. In Proceedings of the 2005 Latin
American conference on Human-computer interaction,
pages 277–282.
Karolita, D., McIntosh, J., Kanij, T., Grundy, J., and Obie,
H. O. (2023). Use of personas in requirements engineer-
ing: A systematic mapping study. Information and Soft-
ware Technology, 162:107264.
Moll
´
eri, J. S. (2024). Supplementary material for creating
data-driven personas for software engineering research.
Available at: https://doi.org/10.5281/zenodo.14182731.
Ramos, H., Fonseca, M., and Ponciano, L. (2021). Mod-
eling and evaluating personas with software explain-
ability requirements. In Human-Computer Interaction:
7th Iberoamerican Workshop, HCI-COLLAB 2021, Sao
Paulo, Brazil, September 8–10, 2021, Proceedings 7,
pages 136–149. Springer.
Schneidewind, L., H
¨
orold, S., Mayas, C., Kr
¨
omker, H.,
Falke, S., and Pucklitsch, T. (2012). How personas sup-
port requirements engineering. In 2012 First Interna-
tional Workshop on Usability and Accessibility Focused
Requirements Engineering (UsARE), pages 1–5. IEEE.
Stack Overflow (2024a). Stack Overflow Developer Sur-
vey 2024. Available at: https://survey.stackoverflow.co/
2024/.
Stack Overflow (2024b). Stack Overflow Insights - Devel-
oper Hiring, Marketing, and User Research. Available
at: https://survey.stackoverflow.co/.
Steinmacher, I., Penney, J. M., Felizardo, K. R., Garcia,
A. F., and Gerosa, M. A. (2024). Can chatgpt emulate hu-
mans in software engineering surveys? In Proceedings of
the 18th ACM/IEEE International Symposium on Empir-
ical Software Engineering and Measurement, pages 414–
419.
Vasilescu, B., Filkov, V., and Serebrenik, A. (2013). Stack-
overflow and github: Associations between software de-
velopment and crowdsourced knowledge. In 2013 In-
ternational conference on social computing, pages 188–
195. IEEE.
Wang, S., Lo, D., and Jiang, L. (2013). An empirical study
on developer interactions in stackoverflow. In Proceed-
ings of the 28th annual ACM symposium on applied com-
puting, pages 1019–1024.
Wang, Y., Arora, C., Liu, X., Hoang, T., Malhotra, V.,
Cheng, B., and Grundy, J. (2024). Who uses personas
in requirements engineering: The practitioners’ perspec-
tive. arXiv preprint arXiv:2403.15917.
Data-Driven Personas for Software Engineering Research
805