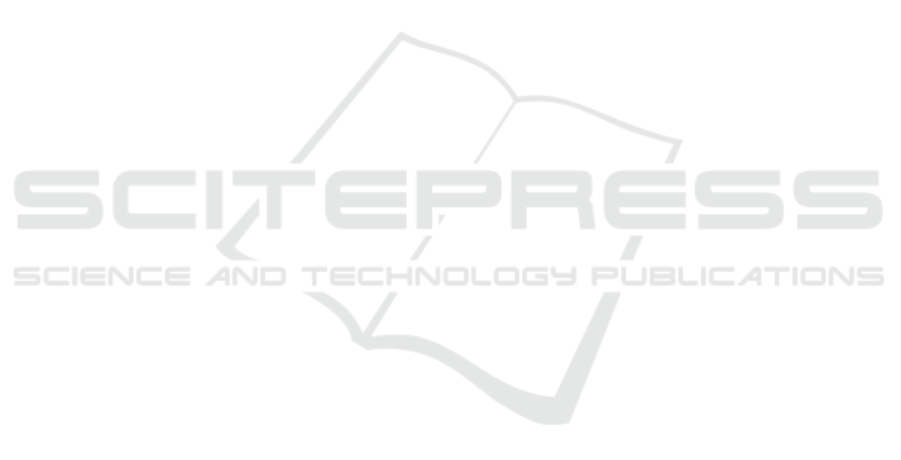
• R
2
: Represents the proportion of variance in the
observed data that is explained by the model.
An R
2
value close to 1 indicates strong agree-
ment between the predicted and observed values,
while values near 0 suggest poor predictive per-
formance.
3 RESULTS
Figure 3 presents canopy height estimation results for
the three test areas, including absolute error maps and
quantitative metrics such as mean absolute error, root
mean square error and R
2
. These figures provide a vi-
sual comparison of the errors associated with the pre-
trained and fine-tuned models across the test areas.
Specifically, for Test Area 1, the fine-tuned model
achieved a MAE of 1.09m, RMSE of 2.09m, and R
2
of 0.88, compared to the pretrained model’s MAE of
1.27m, RMSE of 2.24m, and R
2
of 0.87. In Test Area
2, the fine-tuned model achieved a MAE of 2.69m,
RMSE of 3.63m, and R
2
of 0.83, compared to the pre-
trained model’s MAE of 4.45m, RMSE of 5.52m, and
R
2
of 0.62. Similarly, in Test Area 3, the fine-tuned
model achieved a MAE of 1.48m, RMSE of 2.51m,
and R
2
of 0.66, compared to the pretrained model’s
MAE of 2.04m, RMSE of 3.18m, and R
2
of 0.46. The
visual comparison further underscores the improve-
ment, with reduced absolute error maps for fine-tuned
models.
Based on the quantitative results and visual anal-
ysis shown in Figure 3, the fine-tuned model is over-
all accurate for all three test areas in different years,
comparing to the state-of-the-art (Alagialoglou et al.,
2022; Lang et al., 2023; Tolan et al., 2024), with as
little as 1.4km
2
fine-tuning ground-truth area. Fur-
thermore, the fine-tune model consistently demon-
strated superior performance compared to the pre-
trained model across all test areas and all vegeta-
tion types, as evidenced by all three metrics: MAE,
RMSE, and R
2
. This consistent trend across all test
areas validates the efficacy of the fine-tuning process
in improving model performance.
Similar conclusions are demonstrated in tables 3
and 4. Test Area 2 showed the most significant reduc-
tions in the overall MAE and RMSE for the fine-tuned
models, particularly for deciduous species. This can
be attributed to two factors:
1. The fine-tuning area is a subregion of Test Area
2 for the same year, allowing the models to better
adapt to the specific distribution characteristics of
the area and time.
2. Test Areas 1 and 3 contain large regions domi-
nated by the ”Plantation/Other” class, which gen-
erally exhibits lower canopy height values and,
consequently, smaller errors and lower margin for
improvement.
Detailed quantitative results for each species
across the three test areas are summarized in Tables
3 and 4. These tables demonstrate that the fine-tuned
model consistently outperforms the pretrained model
across all test areas and species, with significant re-
ductions in both MAE and RMSE. The results show
metrics for six species categories, as well as overall
metrics for each test area. Species categories include
Spruce, Pine, Oak, Other Deciduous, Other Conifer-
ous, and Plantation/Other. The metrics are provided
for both pretrained and fine-tuned models to enable
direct comparison.
The Oak class, representing approximately 50%
of the fine-tuning dataset, dominates the model’s
learning and exhibits the most consistent accuracy im-
provements across all test areas with sufficient land
cover percentages. For instance, in Test Area 2, the
fine-tuned model achieves a MAE of 2.89 m, com-
pared to 5.13 m for the pretrained model. Simi-
larly, in Test Area 1, which corresponds to a different
year, the fine-tuned model yields a MAE of 3.23 m,
outperforming the pretrained model’s MAE of 4.51
m. This trend is also evident in Figure 4. In Test
Area 2, the MAE difference between the pretrained
and fine-tuned models for shorter oak trees (1–4 me-
ters) exceeds 1.5 times the actual canopy height. In
contrast, the ”Other deciduous” and ”Plantation &
Other” classes show MAE improvements closer to 1
times the actual canopy height. Additionally, the fine-
tuned model demonstrates consistent accuracy im-
provements for the class Oak across all canopy height
bins, as seen in the left panel of Figure 4. This im-
provement aligns with the class proportions in the
fine-tuning dataset, where Oak dominates (50%), fol-
lowed by ”Plantation & Other” and ”Other Decidu-
ous”.
Test Area 2, at 10 km
2
, is significantly larger than
Test Area 1 (3.6 km
2
) and Test Area 3 (3 km
2
). The
species distribution in Test Areas 1 and 2 primarily
incudes deciduous trees, while Test Area 3 is mostly
coniferous, excluding the ”Plantation/Other” class.
For this reason, the analysis focuses on the dominant
forest classes, avoiding those with small sample sizes.
It is noted that the performance of classes with few
pixels, such as the oak in Test Area 3 or the spruce
and pine in Test Area 1, should not be taken into con-
sideration. Due to the limited number of pixels avail-
able for these classes, results are likely affected by
label noise in the species classification map. How-
ever, although oak is generally absent in Test Area 3,
similarly to spruce and pine in Test Area 1, the results
Assessment of Fine-Tuned Canopy Height Maps from Satellite Imagery: A Case Study in the Czech Republic
239