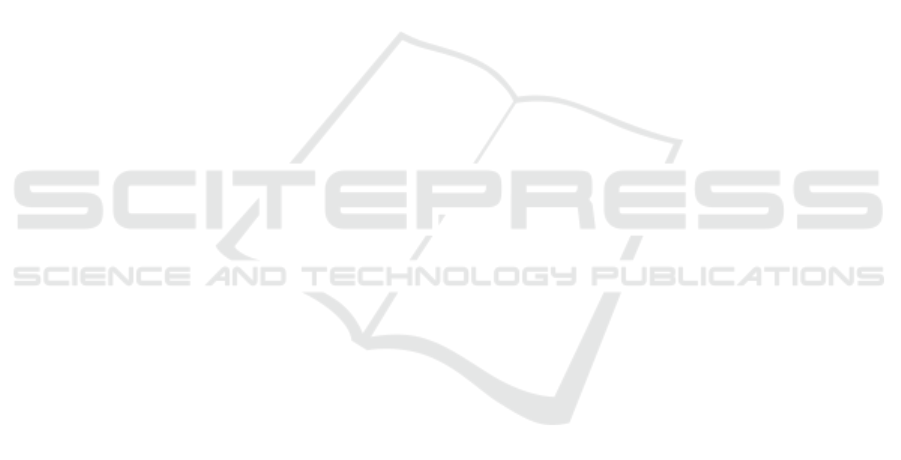
5.2 Experiment Workflows and
Research Practices
Different kinds of artifacts can be considered in ex-
periments, such as scripts, diagrams, and code. Dif-
ferent types of data are also considered. Tabulars,
measurements, metrics, and graphs are examples.
As for data storage locations, personal devices, ver-
sion control repositories, and institutional repositories
were mentioned.
5.3 Measures to Ensure Reproducibility
of Results
In general, the researchers responsible for the exper-
iment considered it easy to recover experimental
data for both input data and results, as well as the
metadata about the methods, steps, and experimental
setup. In the case of data retrieval to be performed by
a novice researcher without any instruction, it was ob-
served that it was difficult to obtain metadata about
methods, steps, and experimental setup.
Regarding the reproduction of experiments to ver-
ify results, we observed that the majority of partici-
pants reported that they did not perform this activity,
which is a concerning factor.
5.4 FAIR Data Principles
Considering the knowledge about the FAIR princi-
ples, we find that a considerable number of partici-
pants know the principles, even if they do not know
exactly what they mean. About application, we ac-
knowledge that some principles can be applied more
frequently. This is the case of the Findable and Ac-
cessible principles, which are essential characteristics
for effective research data.
5.5 Research Practices to Improve
Reproducibility
The sharing of experimental data is considered ab-
solutely essential by participants. Regarding the shar-
ing of metadata about materials, and settings, time,
duration, location, steps, and software used in the
experiment, the participants also considered it abso-
lutely essential. For the sharing of results, we saw
that the sharing of intermediate results was evalu-
ated as medium importance. The sharing of final re-
sults is considered absolutely essential.
6 VALIDITY EVALUATION
In this section, we discuss the main threats to the
validity of our survey based on the guidelines by
Lin
˚
aker et al. (2015) regarding face, content, crite-
rion, and construct. To ensure face validity, the sur-
vey form was reviewed by three researchers during a
pilot project. We focused on achieving content va-
lidity by conducting an unstructured interview with
researchers experienced in experimentation in SE to
review the questionnaire.
To address criterion validity, we organized the
questionnaire into distinct sections, each correspond-
ing to a specific research question. To assess con-
struct validity, we conducted activities during the in-
strument pilot project, the interviews, and the litera-
ture review.
7 PROSPECTIVE ACTIONS
The observed results and respective discussions
present clear evidence that reproducibility must be ad-
dressed in prospective investigations. Therefore, we
will provide some actions to be taken regarding the
topic discussed.
Data and metadata storage options should be ana-
lyzed regarding different options, assessing their ad-
vantages and disadvantages. It might include personal
devices, version-controlled repositories, local servers,
data management platforms, and electronic or physi-
cal lab notebooks. This analysis can aid in identi-
fying best practices for ensuring data accessibility
and integrity, facilitating the reproducibility of ex-
periments.
Creating frameworks for sharing data and meta-
data that can include data repositories, tools for meta-
data management, and best practice guidelines might
encourage data sharing. These frameworks can
help to overcome the lack of availability of data and
complete information on methods and settings, which
have been identified as the main problems for repro-
ducibility.
8 FINAL REMARKS
Based on the results presented and discussed in the
paper, it is clear that there is a need to address the is-
sue of reproducibility in future research in the field
of SE. This study corroborated an apparent repro-
ducibility crisis in the field, caused mainly by the lack
of public data and incomplete information about the
ICEIS 2025 - 27th International Conference on Enterprise Information Systems
378