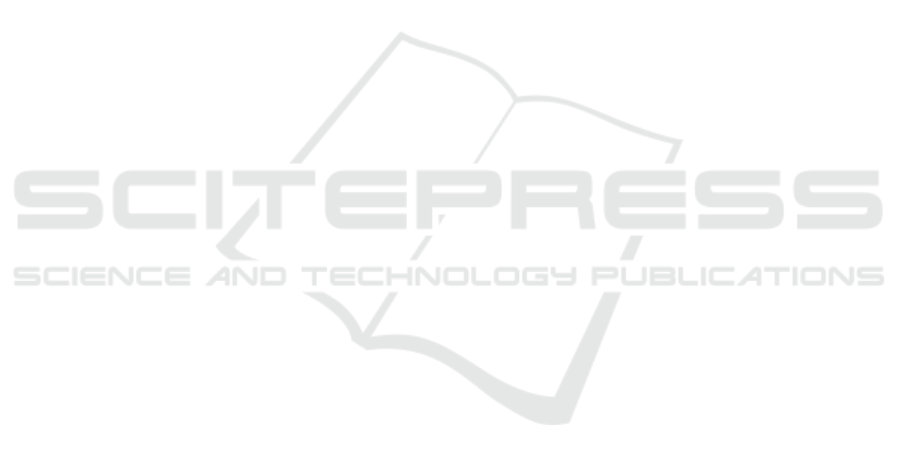
by an object tracking algorithm, was trained for
counting and recognizing honey bee into pollen or
non-pollen bearing class. The YOLOv3-tiny model
was applied to accurately recognize the pollen and
non-pollen bearing honey bees. The F1-score was
0.94 for pollen and non-pollen bearing honey bee
recognition, and the precision and recall values were
0.91 and 0.99, respectively.
In Berkaya et al. (Berkaya et al., 2021), deep
learning-based image classification models are pro-
posed for beehive monitoring. The proposed mod-
els particularly classify honey bee images captured at
beehives and recognize different conditions, such as
healthy bees, pollen-bearing bees, and certain abnor-
malities, such as Varroa parasites, ant problems, hive
rob beries, and small hive beetles. These models uti-
lize transfer learning with seven pre-trained deep neu-
ral networks (AlexNet, DenseNet-201, GoogLeNet,
ResNet- 101, ResNet-18, VGG-16 and VGG-19) and
also a support vector machine classifier with deep fea-
tures, shallow features, and both deep and shallow
features extracted from these DNNs. Three bench-
mark datasets, consisting of a total of 19,393 honey
bee images for different conditions, were used to train
and evaluate the models. The best results were ob-
tained using GoogLeNet which achieved an accuracy
of 0.9907, a recall of 1.0, and a F1-score of 0.9905.
In Monteiro et al. (Monteiro et al., 2021), the
authors analyzed nine Convolutional Neural Net-
works (VGG16, VGG19, ResNet50, ResNet101,
InceptionV3, Inception-ResNetV2, Xception,
DenseNet201 and DarkNet53) for detection of pollen
bearing bees in images obtained at hive entrance.
They also evaluated four different color image
preprocessing techniques (original images, grayscale
images, Contrast Limit Adaptive Histogram Equal-
ization, and Unsharp Masking). The best results
were achieved by applying image pre-processing
with Unsharp Masking, yielding 99.1% accuracy
with the DarkNet53 model and 98.6% accuracy with
the VGG16 model. This work provided a baseline
for pollen bearing bees recognition based in deep
learning.
In Benahmed et al. (Benahmed et al., 2022), the
authors evaluated the use of two deep learning mod-
els, YOLO and StrongSORT, for detecting and track-
ing honey bees. Initially, for detection, they employed
the YOLO model using a data set of 1000 ground truth
images. Next, for tracking, they used the StrongSORT
approach. Results show that the detector performs
well in both classes of honey bees (with or without
pollen).
In Pat-Cetina et al. (Pat-Cetina et al., 2023), the
authors proposed a method for classifying images
of bees based on their pollen-carrying status. They
present two approaches: the first method utilizes a
convolutional neural network (CNN) to classify orig-
inal RGB images, while the second method enhances
pollen regions in the images using digital image pro-
cessing techniques before training the CNN. The re-
sults of the classification metrics demonstrated the ef-
fectiveness of the proposed methods, with the second
method achieving higher accuracy values and reduced
loss compared to the first one.
In Nguyen et al. (Nguyen et al., 2024), the authors
introduced an efficient method for pollen-bearing bee
detection. Initially, they furnish a comprehensive
dataset, dubbed VnPollenBee, meticulously anno-
tated for pollen-bearing honey bee detection and clas-
sification. The dataset comprises 60,826 annotated
boxes that delineate both pollen-bearing and non-
pollen-bearing bees in 2,051 images captured at the
entrances of beehives under various environmental
conditions. Next, they propose the incorporation of
diverse techniques into two baseline models, namely
YOLOv5 and Faster RCNN, to effectively address the
imbalance that arises during the detection of pollen-
bearing bees due to their number being typically
much lower than the total number of bees present at
hive entrances. The experimental results demonstrate
that the proposed approach outperforms the baseline
models on the VnPollenBee dataset, yielding Preci-
sion, Recall, and F1 score of 99%, 93%, and 95%,
respectively. Specifically, the improvements obtained
are 3% and 2% in Recall and F1 score when using
YOLOv5, and 3%, 2%, and 2% in Precision, Recall,
and F1 score when using Faster RCNN.
In Bilik et al. (Bilik et al., 2024), the authors con-
ducted a survey analyzing over 50 research projects
on automated beehive monitoring methods utilizing
computer vision techniques. The majority of the ap-
proaches reviewed focus on facilitating pollen detec-
tion, Varroa mite identification, and bee traffic moni-
toring.
In Rohafauzi et al. (Rohafauzi et al., 2024), the
authors presented a review on honey production and
prediction modeling for stingless bees. The study an-
alyzed and compared approximately 54 previous re-
search works to identify research gaps. Addition-
ally, a framework for modeling the prediction of stin-
gless bee honey production was developed. The re-
sults include a detailed comparison and analysis of
Internet of Things (IoT) monitoring systems, honey
production estimation methods, convolutional neural
networks (CNNs), and automatic identification tech-
niques for bee species. This study provides valuable
insights for researchers aiming to develop predictive
models for stingless bee honey production, which is
Influence of Quantization of Convolutional Neural Networks on Image Classification of Pollen-Bearing Bees
149