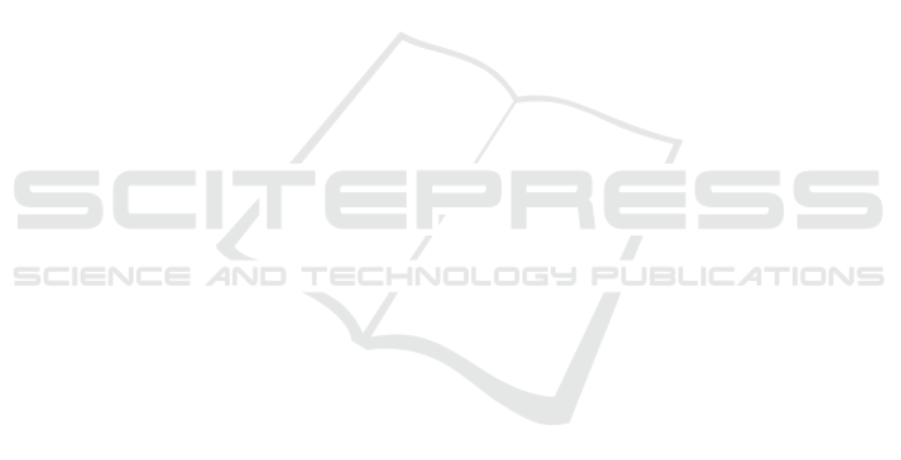
tings. This has implications for urban planning, re-
newable energy investments, and policy-making. The
method can be replicated in other regions, validating
its applicability across diverse geographical and cli-
matic contexts.
ACKNOWLEDGEMENTS
We thank the HMWVW for the part-funding of this
work.
REFERENCES
Breiman, L. (2001). Random forests. Machine Learning,
45(1):5–32.
Chen, T. and Guestrin, C. (2016). Xgboost: A scalable
tree boosting system. In Proceedings of the 22nd
ACM SIGKDD International Conference on Knowl-
edge Discovery and Data Mining, pages 785–794.
ACM.
Dorogush, A. V., Ershov, V., and Gulin, A. (2018). Cat-
boost: Gradient boosting with categorical features
support. ArXiv, abs/1810.11363.
Figueiredo, R., Nunes, P., and Brito, M. C. (2017). The
feasibility of solar parking lots for electric vehicles.
Energy, 140:1182–1197.
Frazier, P. I. (2018). A tutorial on bayesian optimization.
arXiv preprint arXiv:1807.02811.
Geurts, P., Ernst, D., and Wehenkel, L. (2006). Extremely
randomized trees. Machine Learning, 63(1):3–42.
Head, T., Pak, M., Louppe, G., Shcherbatyi, I., Char-
ras, F., Vin
´
ıcius, Z., and Fabisch, A. (2018). scikit-
optimize/scikit-optimize.
HMWEVW (2023). Photovoltaikpflicht f
¨
ur Parkpl
¨
atze und
Landesgeb
¨
aude. Press Release.
IPCC (2022). Climate Change 2022: Mitigation of Cli-
mate Change. Contribution of Working Group III to
the Sixth Assessment Report of the IPCC. Cambridge
University Press. https://www.ipcc.ch/report/ar6/wg3/
(accessed on 16 January 2025).
Ivanova, A., Chassin, D., Aguado, J., Crawford, C., and
Djilali, N. (2020). Techno-economic feasibility of a
photovoltaic-equipped plug-in electric vehicle public
parking lot with coordinated charging. IET Energy
Systems Integration, 2(3):261–272.
Ke, G., Meng, Q., Finley, T., Wang, T., Chen, W., Ma, W.,
Ye, Q., and Liu, T.-Y. (2017). Lightgbm: A highly ef-
ficient gradient boosting decision tree. In Advances in
Neural Information Processing Systems, pages 3146–
3154.
Krishnan, R., Haselhuhn, A., and Pearce, J. M. (2017).
Technical solar photovoltaic potential of scaled park-
ing lot canopies: A case study of walmart usa. Journal
on Innovation and Sustainability RISUS, 8(2):104–
125.
Landes Energie Agentur Hessen (2025). Solar Kataster
Hessen: A Tool for Utilizing Solar Energy. Accessed:
2025-01-22.
Ludwig, D., Tegeler, A., Schmedes, D., Tomhave, L.,
Hensel, A., Forster, J., Kleinhans, A., Heinrich, M.,
John, R., and Schill, C. (2024). Potenziale f
¨
ur Photo-
voltaik an Bundesfernstraßen. Technical report, Bun-
desanstalt f
¨
ur Straßenwesen (BASt), Bergisch Glad-
bach, Germany.
Lundberg, S. M. and Lee, S.-I. (2017). A unified approach
to interpreting model predictions. In Proceedings of
the 31st International Conference on Neural Infor-
mation Processing Systems, pages 4768–4777. Curran
Associates Inc.
Maier, R., L
¨
utz, L., Risch, S., Kullmann, F., Weinand, J.,
and Stolten, D. (2024). Potential of floating, parking,
and agri photovoltaics in germany. Renewable and
Sustainable Energy Reviews, 200:114500.
Marneni, N., Agarwal, N., and Munshi, N. (2021). Techno-
economic analysis of solar carports for a commer-
cial building: A u.s. case study. Energy Reports,
7:160–168. https://www.sciencedirect.com/science/
article/pii/S2352484721000686 (accessed on 16 Jan-
uary 2025).
More, A. (2016). Survey of resampling techniques for
improving classification performance in unbalanced
datasets. ArXiv, abs/1608.06048.
Pedregosa, F., Varoquaux, G., Gramfort, A., Michel, V.,
Thirion, B., Grisel, O., Blondel, M., Prettenhofer, P.,
Weiss, R., Dubourg, V., Vanderplas, J., Passos, A.,
Cournapeau, D., Brucher, M., Perrot, M., and Duch-
esnay, E. (2011). scikit-learn: Machine learning in
python. https://scikit-learn.org/. Version 0.24.2.
Santamouris, M. (2020). Recent progress on urban over-
heating and heat island research: A review. Current
Opinion in Environmental Science & Health, 13:89–
97.
Solar Cluster BW (2022). Photovoltaik-Parkpl
¨
atze: So-
lar
¨
uberdachung von Park- und Stellpl
¨
atzen. Tech-
nical report, Solar Cluster Baden-W
¨
urttemberg e.V.,
Stuttgart, Germany. Faktenpapier des Photovoltaik-
Netzwerks Baden-W
¨
urttemberg. Original authors:
Solar Cluster Baden-W
¨
urttemberg e.V., Gerhard
Stryi-Hipp, Thomas Uhland.
Wirth, H. (2023). Aktuelle Fakten zur Photovoltaik in
Deutschland. Technical report, Fraunhofer-Institut f
¨
ur
Solare Energiesysteme ISE. Available at www.pv-
fakten.de, accessed on January 20, 2025.
GISTAM 2025 - 11th International Conference on Geographical Information Systems Theory, Applications and Management
252