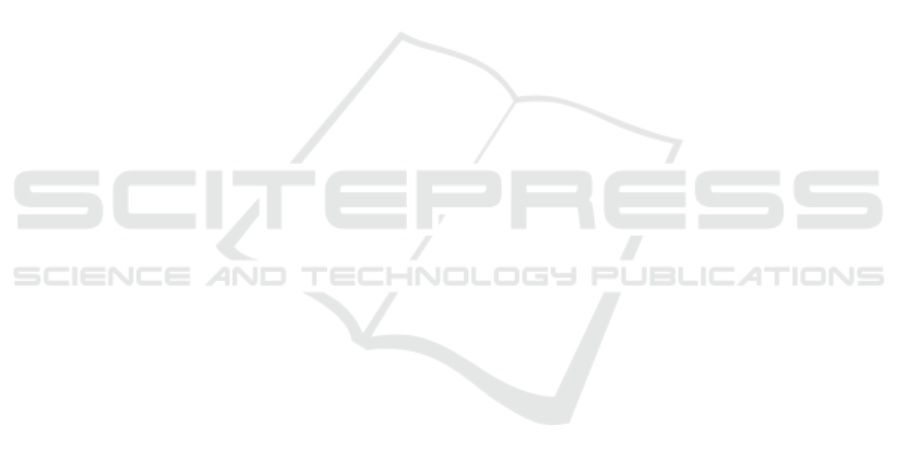
ACKNOWLEDGMENT
This research has supported by the EU Horizon 2020
Research and Innovation Programme, grant agree-
ment No. 856602 and Horizon Europe project No.
101057369.
REFERENCES
Alves, J., Lima, T. M., and Gaspar, P. D. (2023). Is industry
5.0 a human-centred approach? a systematic review.
Processes, 11(1):193.
Chance, G., Ghobrial, A., McAreavey, K., Lemaignan, S.,
Pipe, T., and Eder, K. (2022). On determinism of
game engines used for simulation-based autonomous
vehicle verification. IEEE Transactions on Intelligent
Transportation Systems, 23(11):20538–20552.
Dosovitskiy, A., Ros, G., Codevilla, F., Lopez, A., and
Koltun, V. (2017). Carla: An open urban driving sim-
ulator. In Conference on robot learning, pages 1–16.
PMLR.
Hensher, D. A., Mulley, C., Ho, C., Wong, Y., Smith, G.,
and Nelson, J. D. (2020). Understanding Mobility as
a Service (MaaS): Past, present and future. Elsevier.
Hu, X., Li, S., Huang, T., Tang, B., Huai, R., and Chen,
L. (2024). How simulation helps autonomous driving:
A survey of sim2real, digital twins, and parallel in-
telligence. IEEE Transactions on Intelligent Vehicles,
9(1):593–612.
Kalda, K., Pizzagalli, S.-L., Soe, R.-M., Sell, R., and Bel-
lone, M. (2022). Language of driving for autonomous
vehicles. Applied Sciences, 12(11):5406.
Kalda, K., Sell, R., and Kivim
¨
ae, M. (2024). Enhancing
mobility as a service with autonomous last-mile shut-
tles and data exchange layer for public transport. In
AIP Conference Proceedings, volume 2989.
Malayjerdi, M., Baykara, B. C., Sell, R., and Malayjerdi,
E. (2021). Autonomous vehicle safety evaluation
through a high-fidelity simulation approach. Proceed-
ings of the Estonian Academy of Sciences, 70(4):413–
421.
Malayjerdi, M., Kuts, V., Sell, R., Otto, T., and Baykara,
B. C. (2020). Virtual simulations environment devel-
opment for autonomous vehicles interaction. In ASME
International Mechanical Engineering Congress and
Exposition, volume 84492, page V02BT02A009.
American Society of Mechanical Engineers.
Medrano-Berumen, C., Malayjerdi, M., Akbas¸, M.
˙
I., Sell,
R., and Razdan, R. (2020). Development of a Valida-
tion Regime for an Autonomous Campus Shuttle. In
IEEE SoutheastCon, pages 1–8.
Michal
´
ık, D., Jirgl, M., Arm, J., and Fiedler, P. (2021).
Developing an unreal engine 4-based vehicle driving
simulator applicable in driver behavior analysis—a
technical perspective. Safety, 7(2):25.
Paide, K., Pappel, I., Vainsalu, H., and Draheim, D. (2018).
On the systematic exploitation of the estonian data ex-
change layer x-road for strengthening public-private
partnerships. In proceedings of the 11th international
conference on theory and practice of electronic gov-
ernance, pages 34–41.
Pikner, H., Malayjerdi, M., Bellone, M., and Sell, R. (2024).
Autonomous driving validation and verification using
digital twins. In International Conference on Vehi-
cle Technology and Intelligent Transport Systems (VE-
HITS), pages 204–211.
Pikner, H., Sell, R., Majak, J., and Karjust, K. (2022).
Safety system assessment case study of automated ve-
hicle shuttle. Electronics, 11:1162.
Pisarov, J. L. and Mester, G. (2021). The use of autonomous
vehicles in transportation. Tehnika, 76(2):171–177.
Rensfeldt, S. and Kniele, L. (2024). Navigating global sup-
ply chain disruptions: A case study of volvo trucks for
enhanced resilience and risk management in the auto-
motive sector.
Roberts, A., Maennel, O., and Snetkov, N. (2021). Cyber-
security test range for autonomous vehicle shuttles. In
2021 IEEE European Symposium on Security and Pri-
vacy Workshops (EuroS&PW), pages 239–248.
Roberts, A., Malayjerdi, M., Bellone, M., Maennel, O.,
and Malayjerdi, E. (2023). Analysing adversarial
threats to rule-based local-planning algorithms for au-
tonomous driving. In Network and Distributed System
Security (NDSS) Symposium.
Robles, G., Gamalielsson, J., and Lundell, B. (2019).
Setting up government 3.0 solutions based on open
source software: the case of x-road. In Electronic
Government: 18th IFIP WG 8.5 International Con-
ference, EGOV 2019, San Benedetto Del Tronto, Italy,
September 2–4, 2019, Proceedings 18, pages 69–81.
Springer.
Sarhadi, P. and Yousefpour, S. (2015). State of the art: hard-
ware in the loop modeling and simulation with its ap-
plications in design, development and implementation
of system and control software. International Journal
of Dynamics and Control, 3:470–479.
Sell, R., Malayjerdi, E., Malayjerdi, M., and Baykara, B. C.
(2022). Safety toolkit for automated vehicle shuttle
- practical implementation of digital twin. In 2022
International Conference on Connected Vehicle and
Expo (ICCVE), pages 1–6.
Sell, R., Malayjerdi, M., Pikner, H., Razdan, R., Malay-
jerdi, E., and Bellone, M. (2024). Open-source level
4 autonomous shuttle for last-mile mobility. In 2024
IEEE 29th International Conference on Emerging
Technologies and Factory Automation (ETFA), pages
01–06. IEEE.
Slamnik-Krije
ˇ
storac, N., Yilma, G. M., Liebsch, M.,
Yousaf, F. Z., and Marquez-Barja, J. M. (2023). Col-
laborative orchestration of multi-domain edges from
a connected, cooperative and automated mobility
(ccam) perspective. IEEE Transactions on Mobile
Computing, 22(4):2001–2020.
Umang, G. S. R., Shivanandaswamy, K. K., Pallavi, S.,
and Rajagopal, S. (2024). Software-in-the-loop (sil)
method. In Proceedings of the 10th International
Conference on Mechanical, Automotive and Materi-
als Engineering: CMAME 2023, 20–22 December, Da
Nang, Vietnam, page 243. Springer Nature.
Volvo Autonomous Solutions (2025). Autonomous trans-
port solution by volvo. Accessed: 2025-01-19.
Autonomous Vehicle for Industry 5.0: Digital Twin for System Safety Validation
667