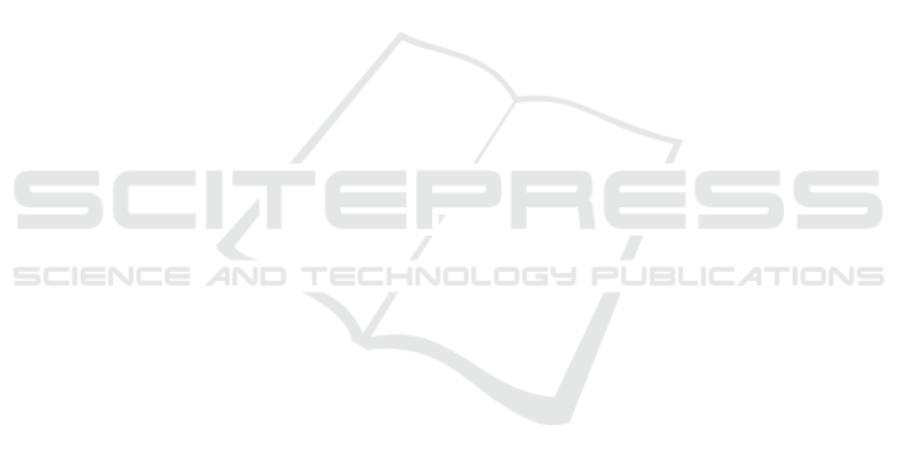
method as the next step.
REFERENCES
Al-Zebari, A. (2024). Ensemble convolutional neural
networks and transformer-based segmentation meth-
ods for achieving accurate sclera segmentation in
eye images. Signal, Image and Video Processing,
18(2):1879–1891.
André, B., Vercauteren, T., Buchner, A. M., Wallace, M. B.,
and Ayache, N. (2012). Learning semantic and visual
similarity for endomicroscopy video retrieval. IEEE
Transactions on Medical Imaging, 31(6):1276–1288.
Apicella, A., Isgrò, F., and Prevete, R. (2024). Don’t
push the button! exploring data leakage risks in ma-
chine learning and transfer learning. arXiv preprint
arXiv:2401.13796.
Baby, A. and Krishnan, H. (2017). A literature survey on
data leak detection and prevention methods. Interna-
tional Journal of Advanced Research in Computer Sci-
ence, 8(5).
Bussola, N., Marcolini, A., Maggio, V., Jurman, G., and
Furlanello, C. (2021). Ai slipping on tiles: Data leak-
age in digital pathology. In International Conference
on Pattern Recognition, pages 167–182. Springer In-
ternational Publishing Cham.
Casas, E., Ramos, L., Bendek, E., and Rivas-Echeverría, F.
(2023). Assessing the effectiveness of yolo architec-
tures for smoke and wildfire detection. IEEE Access,
11:96554–96583.
Cawley, G. C. and Talbot, N. L. (2010). On over-fitting in
model selection and subsequent selection bias in per-
formance evaluation. The Journal of Machine Learn-
ing Research, 11:2079–2107.
De Waal, F. (2016). Are we smart enough to know how
smart animals are? WW Norton & Company.
Drobnjakovi
´
c, F., Suboti
´
c, P., and Urban, C. (2022). Ab-
stract interpretation-based data leakage static analysis.
arXiv preprint arXiv:2211.16073.
Geiger, A., Lenz, P., Stiller, C., and Urtasun, R. (2013).
Vision meets robotics: The kitti dataset. The Inter-
national Journal of Robotics Research, 32(11):1231–
1237.
Götz-Hahn, F., Hosu, V., and Saupe, D. (2022). Crit-
ical analysis on the reproducibility of visual qual-
ity assessment using deep features. Plos one,
17(8):e0269715.
Gupta, A., Anpalagan, A., Guan, L., and Khwaja, A. S.
(2021). Deep learning for object detection and scene
perception in self-driving cars: Survey, challenges,
and open issues. Array, 10:100057.
Hussein, E. A., Ghaziasgar, M., Thron, C., Vaccari, M., and
Jafta, Y. (2022). Rainfall Prediction Using Machine
Learning Models: Literature Survey, pages 75–108.
Springer International Publishing, Cham.
Kapoor, S. and Narayanan, A. (2023). Leakage and the
reproducibility crisis in machine-learning-based sci-
ence. Patterns, 4(9).
Kernbach, J. M. and Staartjes, V. E. (2022). Foundations
of machine learning-based clinical prediction model-
ing: Part ii—generalization and overfitting. Machine
Learning in Clinical Neuroscience: Foundations and
Applications, pages 15–21.
Kiran, B. R., Sobh, I., Talpaert, V., Mannion, P., Al Sallab,
A. A., Yogamani, S., and Pérez, P. (2021). Deep rein-
forcement learning for autonomous driving: A survey.
IEEE Transactions on Intelligent Transportation Sys-
tems, 23(6):4909–4926.
Lapuschkin, S., Wäldchen, S., Binder, A., Montavon, G.,
Samek, W., and Müller, K.-R. (2019). Unmasking
clever hans predictors and assessing what machines
really learn. Nature communications, 10(1):1096.
Li, Y., Li, S., Du, H., Chen, L., Zhang, D., and Li, Y. (2020).
Yolo-acn: Focusing on small target and occluded ob-
ject detection. IEEE Access, 8:227288–227303.
Li, Huaxin, Ma, Di, Medjahed, Brahim, Wang, Qianyi,
Kim, Yu Seung, and Mitra, Pramita (2017). Secure
and privacy-preserving data collection mechanisms
for connected vehicles. In WCX™ 17: SAE World
Congress Experience. SAE International.
Ma, X., Ouyang, W., Simonelli, A., and Ricci, E. (2023). 3d
object detection from images for autonomous driving:
a survey. IEEE Transactions on Pattern Analysis and
Machine Intelligence.
Monga, V., Banerjee, A., and Evans, B. L. (2006). A clus-
tering based approach to perceptual image hashing.
IEEE Transactions on Information Forensics and Se-
curity, 1(1):68–79.
Pulini, A. A., Kerr, W. T., Loo, S. K., and Lenartowicz,
A. (2019). Classification accuracy of neuroimaging
biomarkers in attention-deficit/hyperactivity disorder:
effects of sample size and circular analysis. Biological
Psychiatry: Cognitive Neuroscience and Neuroimag-
ing, 4(2):108–120.
Rashed, H., Mohamed, E., Sistu, G., Kumar, V. R., Eis-
ing, C., El-Sallab, A., and Yogamani, S. (2021). Gen-
eralized object detection on fisheye cameras for au-
tonomous driving: Dataset, representations and base-
line. In Proceedings of the IEEE/CVF Winter Con-
ference on Applications of Computer Vision, pages
2272–2280.
Rouzrokh, P., Khosravi, B., Faghani, S., Moassefi, M.,
Vera Garcia, D. V., Singh, Y., Zhang, K., Conte,
G. M., and Erickson, B. J. (2022). Mitigating bias
in radiology machine learning: 1. data handling. Ra-
diology: Artificial Intelligence, 4(5):e210290.
Sculley, D., Holt, G., Golovin, D., Davydov, E., Phillips,
T., Ebner, D., Chaudhary, V., Young, M., Crespo, J.-
F., and Dennison, D. (2015). Hidden technical debt in
machine learning systems. Advances in neural infor-
mation processing systems, 28.
Shim, M., Lee, S.-H., and Hwang, H.-J. (2021). Inflated
prediction accuracy of neuropsychiatric biomarkers
caused by data leakage in feature selection. Scientific
Reports, 11(1):7980.
Silva, G. F., Fagundes, T. P., Teixeira, B. C., and Chiave-
gatto Filho, A. D. (2022). Machine learning for hy-
pertension prediction: a systematic review. Current
Hypertension Reports, 24(11):523–533.
VEHITS 2025 - 11th International Conference on Vehicle Technology and Intelligent Transport Systems
220