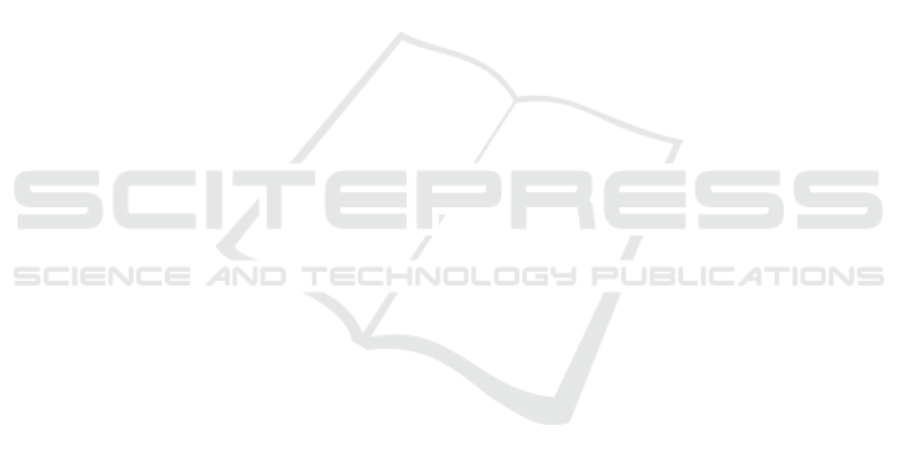
problems related to game and software development
were extracted from the 200 post-mortems that were
considered. The collected video game development
problems were grouped into three groups: manage-
ment, production, and business, with each group be-
ing further divided into various subgroups of prob-
lems. Business problems arose due to issues related
to marketing and monetization, while management
problems were further divided as problems based on
communication, delays, crunch time, cutting features,
team, security, budget, feature creep, planning, scope,
and multiple projects. Finally, production related
problems included technical problems like bugs, de-
sign, testing, tools, prototyping, and documentation.
3.2 Word Embeddings
The proposed research framework makes use of Word
embeddings to represent the post-mortems of video
games in terms of real-valued vectors. These fea-
ture vectors encode the meaning of the words in
such a manner that words with a similar meaning are
closer in the vector space, thereby reducing the fea-
ture space. In this work, we have used 07-word em-
bedding techniques: TFIDF, Skipgram (SKG), CBOW,
FastText (FAT), Word2Vec (W2V), GLoVe, and BERT.
Although TFIDF is a frequency-based method while
the others are neural network-based, all of them
try to represent a given word as a vector in the n-
dimensional vector space. Before using the word em-
beddings, the data was pre-processed to remove stop-
words, bad symbols, spaces, etc. Finally, the predic-
tive power of the word embeddings has been com-
pared in the upcoming sections.
3.3 SMOTE
The dataset collected by Politowski et al., has 430
data points for the majority class and less than 100
data points for the minority class, meaning that it is
imbalanced (Politowski et al., 2020). Training ML
models on imbalanced data could lead to a bias since
conventional ML algorithms like logistic regression,
DT, etc., possess a bias towards the majority class
(Hoens and Chawla, 2013). This is because such al-
gorithms increase accuracy by reducing the error and
do not consider class distribution in general. In fact,
this problem is prevalent in other domains such as
fraud detection, face identification, anomaly detec-
tion, etc. Hence, the considered dataset is balanced
using SMOTE (Fern
´
andez et al., 2018) by artificially
replicating minority class instances.
3.4 Feature Selection
In this work, we use three feature selection techniques
(PCA, LDA, and ANOVA) to eliminate redundant or
irrelevant features before performing the classifica-
tion task. The predictive power of the classifiers af-
ter performing SMOTE and feature selection is com-
pared using AUC, F-measure, and Accuracy values.
3.5 MLP-Based Classification Models
In this work, we use the multi-layer perceptron (MLP)
to perform the classification task. Having already
worked with basic ML algorithms (Anirudh et al.,
2021), and with previous research indicating that
MLP has a better predictive ability than traditional
ML algorithms like KNN, SVC, this work performs
experiments on MLP to test its predictive ability. An
MLP is a fully connected dense neural network that
has one input layer with one neuron for each input.
Further, it has one output layer with a single node for
each output (in our case, one node for each group of
game development problems). In this work, we have
used implemented nine MLPs with combinations of
one, two, and three hidden layers, and Adam, LBFGS,
and Stochastic Gradient optimizers. ReLU is used as
the activation function for all nine classifiers, with a
maximum limit of 300 iterations.
4 RESEARCH METHODOLOGY
The proposed pipeline aims to build a game devel-
opment community by identifying game development
problems based on their descriptions. This is achieved
through a technical analysis and comparative study of
nine MLP models and five ML classifiers (Anirudh
A. et al., 2021) using 5-fold cross-validation. A clas-
sifier is trained to predict problem types from given
descriptions, helping developers recognize issues and
find solutions efficiently.
Figure 1 outlines the research framework. First,
problem descriptions are converted into numerical
vectors using word embeddings. The dataset is then
balanced with SMOTE before feature selection, en-
suring that feature importance is not skewed by im-
balance. SMOTE is applied only to the training
data to avoid artificially generated points in test-
ing/validation. Finally, classification is performed
following feature selection. The performance of var-
ious word embedding and classification techniques is
evaluated and compared. Results from the original
dataset are analyzed alongside those from SMOTE-
sampled data using metrics such as accuracy, F-
ENASE 2025 - 20th International Conference on Evaluation of Novel Approaches to Software Engineering
816