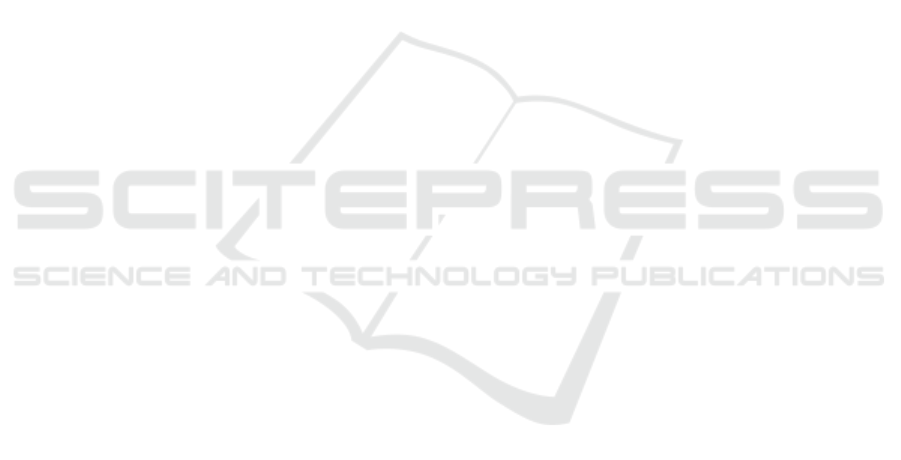
In future, the research can be directed to de-
crease differences between manual and automated
localization. Increasing the Top-1 accuracy of our
approach will match the suggested properties with
the developers preferences more accurately. Better
localization will play a significant role in generating a
more suitable repair solution for the RLFs.
ACKNOWLEDGEMENTS
This research is supported by the Fellowship from
ICT Division, Government of Bangladesh; No-
56.00.0000.052.33.002.24-61, dated 06.06.2024.
REFERENCES
Althomali, I., Kapfhammer, G. M., and McMinn, P. (2019).
Automatic visual verification of layout failures in re-
sponsively designed web pages. In 12th IEEE Confer-
ence on Software Testing, Validation and Verification,
ICST 2019, Xi’an, China, April 22-27, 2019, pages
183–193. IEEE.
Althomali, I., Kapfhammer, G. M., and McMinn, P. (2022).
Automated repair of responsive web page layouts. In
15th IEEE Conference on Software Testing, Verifica-
tion and Validation, ICST 2022, Valencia, Spain, April
4-14, 2022, pages 140–150. IEEE.
Chiou, P. T., Winn, R., Alotaibi, A. S., and Halfond, W. G. J.
(2024). Automatically detecting reflow accessibility
issues in responsive web pages. In Proceedings
of the IEEE/ACM 46th International Conference on
Software Engineering, ICSE 2024, ICSE ’24, pages
1811–1823, New York, NY, USA. ACM.
Choudhary, S. R., Prasad, M. R., and Orso, A. (2013). X-
PERT: accurate identification of cross-browser issues
in web applications. In Proceedings of the IEEE/ACM
35th International Conference on Software Engineer-
ing, ICSE 2013, San Francisco, CA, USA, May 18-26,
2013, pages 702–711. IEEE Computer Society.
Jacquet, S., Chamberland-Thibeault, X., and Hall
´
e, S.
(2021). Automated repair of layout bugs in web pages
with linear programming. In 21st International Con-
ference on Web Engineering, ICWE 2021, Biarritz,
France, May 18-21, 2021, pages 423–439. Springer
International Publishing.
Kim, Y., Kim, M., and Lee, E. (2024). Noisy token removal
for bug localization: The impact of semantically con-
fusing misguiding terms. IEEE Access, 12:172396–
172409.
Le-Cong, T., Le, X. B. D., Huynh, Q. T., and Nguyen, P. L.
(2021). Usability and Aesthetics: Better Together
for Automated Repair of Web Pages . In IEEE
32nd International Symposium on Software Reliability
Engineering, ISSRE 2021, Wuhan, China, October
25-28, 2021, pages 173–183. IEEE Computer Society.
Leotta, M., Paparella, D., and Ricca, F. (2024a). Mutta:
a novel tool for e2e web mutation testing. Software
Quality Journal, 32(1):5–26.
Leotta, M., Ricca, F., Marchetto, A., and Olianas, D.
(2024b). An empirical study to compare three web test
automation approaches: Nlp-based, programmable,
and capture&replay. Journal of Software: Evolution
and Process, 36(5):e2606.
Liu, Z., Chen, C., Wang, J., Huang, Y., Hu, J., and Wang,
Q. (2023). Nighthawk: Fully Automated Localizing
UI Display Issues via Visual Understanding . IEEE
Transactions on Software Engineering, 49(01):403–
418.
Mahajan, S., Abolhassani, N., McMinn, P., and Halfond,
W. G. J. (2018a). Automated repair of mobile friendly
problems in web pages. In Proceedings of the 40th
International Conference on Software Engineering,
ICSE 2018, Gothenburg, Sweden, May 27 - June 03,
2018, pages 140–150. ACM.
Mahajan, S., Alameer, A., McMinn, P., and Halfond, W.
G. J. (2018b). Automated repair of internationaliza-
tion presentation failures in web pages using style
similarity clustering and search-based techniques. In
11th IEEE International Conference on Software Test-
ing, Verification and Validation, ICST 2018, V
¨
aster
˚
as,
Sweden, April 9-13, 2018, pages 215–226. IEEE
Computer Society.
Mahajan, S., Alameer, A., McMinn, P., and Halfond,
W. G. J. (2021). Effective automated repair of in-
ternationalization presentation failures in web appli-
cations using style similarity clustering and search-
based techniques. Software Testing, Verification and
Reliability, 31(1-2):e1746.
Nama, P. (2024). Integrating AI in testing automation: En-
hancing test coverage and predictive analysis for im-
proved software quality. World Journal of Advanced
Engineering Technology and Sciences, (13):01.
Rahman, S., Rahman, M. M., and Sakib, K. (2017).
A statement level bug localization technique using
statement dependency graph. In Proceedings of the
12th International Conference on Evaluation of Novel
Approaches to Software Engineering, ENASE 2017,
Porto, Portugal, April 28-29, 2017, pages 171–178.
SciTePress.
Walsh, T. A., Kapfhammer, G. M., and McMinn, P. (2017a).
Automated layout failure detection for responsive web
pages without an explicit oracle. In Proceedings of
the 26th ACM SIGSOFT International Symposium on
Software Testing and Analysis, Santa Barbara, CA,
USA, July 10 - 14, 2017, pages 192–202. ACM.
Walsh, T. A., Kapfhammer, G. M., and McMinn, P. (2017b).
Redecheck: an automatic layout failure checking tool
for responsively designed web pages. In Proceedings
of the 26th ACM SIGSOFT International Symposium
on Software Testing and Analysis, Santa Barbara, CA,
USA, July 10 - 14, 2017, pages 360–363. ACM.
Walsh, T. A., McMinn, P., and Kapfhammer, G. M. (2015).
Automatic detection of potential layout faults follow-
ing changes to responsive web pages (N). In 30th
IEEE/ACM International Conference on Automated
Software Engineering, ASE 2015, Lincoln, NE, USA,
November 9-13, 2015, pages 709–714. IEEE Com-
puter Society.
A Heuristic Approach to Localize CSS Properties for Responsive Layout Failures
303