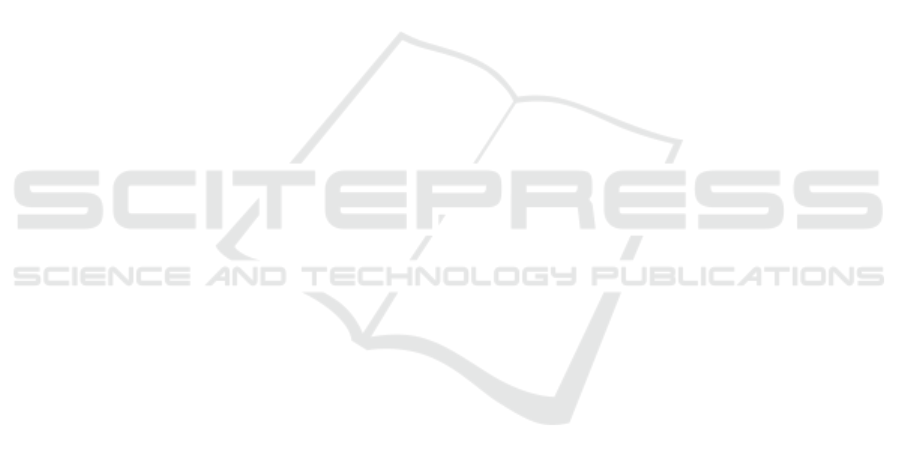
documents and made accessible through our platform.
Exam questions are seamlessly integrated into a learn-
ing system that employs a spaced repetition algorithm
to optimize knowledge retention.
Our approach prioritizes content relevance over
efficiency, distinguishing it from typical RAG-based
systems. Key enhancements include a Query
Rephraser, an advanced retrieval system, and a re-
fined reranker. These improvements significantly
increased retrieval performance, notably raising the
number of total relevant documents from 4.59 to 6.83
out of 10.
To ensure quality and reliability, the output under-
went rigorous manual verification by medical special-
ists. The system is now in its final development stages
and will soon be deployed in production.
ACKNOWLEDGEMENTS
We acknowledge CEM’s courtesy in permitting the
use of past PES questions and extend our gratitude to
all medical annotators for their contributions to devel-
opment and validation. This article has been written
with the help of GPT-4o (GPT, 2024) and Grammarly
AI Assitant (Grammarly, ).
REFERENCES
(2024). Gpt-4 technical report.
Ahlberg, G., Enochsson, L., Gallagher, A. G., Hedman,
L., Hogman, C., McClusky, D. A., Ramel, S., Smith,
C. D., and Arvidsson, D. (2007). Proficiency-based
virtual reality training significantly reduces the error
rate for residents during their first 10 laparoscopic
cholecystectomies. The American Journal of Surgery,
193(6):797–804.
Ankit Pal, M. S. (2024). Openbiollms: Advancing
open-source large language models for healthcare
and life sciences. https://huggingface.co/aaditya/
OpenBioLLM-Llama3-70B.
Camlet, A., Kusiak, A., and
´
Swietlik, D. (2025). Applica-
tion of conversational ai models in decision making
for clinical periodontology: Analysis and predictive
modeling. AI, 6(1):3.
Colt, H. G., Crawford, S. W., and Galbraith, O. (2001). Vir-
tual reality bronchoscopy simulation: A revolution in
procedural training. Chest, 120(4):1333–1339.
Dadas, S. and Gr˛ebowiec, M. (2024). Assessing generaliza-
tion capability of text ranking models in polish.
Elsevier (2025). ClinicalKey AI. Accessed: 2025-01-22.
Grammarly. Grammarly - ai writing assistant. https://www.
grammarly.com. Accessed: 2025-01-22.
Grzybowski, Ł., Pokrywka, J., Ciesiółka, M., Kacz-
marek, J. I., and Kubis, M. (2024). Polish med-
ical exams: A new dataset for cross-lingual medi-
cal knowledge transfer assessment. arXiv preprint
arXiv:2412.00559.
Horst, R., Witsch, L.-M., Hazunga, R., Namuziya, N.,
Syakantu, G., Ahmed, Y., Cherkaoui, O., Andreadis,
P., Neuhann, F., and Barteit, S. (2023). Evaluating the
effectiveness of interactive virtual patients for medi-
cal education in zambia: Randomized controlled trial.
JMIR Med Educ, 9:e43699.
Huang, L., Yu, W., Ma, W., Zhong, W., Feng, Z., Wang, H.,
Chen, Q., Peng, W., Feng, X., Qin, B., et al. (2023).
A survey on hallucination in large language models:
Principles, taxonomy, challenges, and open questions.
ACM Transactions on Information Systems.
johnsnowlabs (2024). Jsl-medllama-3-8b-
v2.0. https://huggingface.co/johnsnowlabs/
JSL-MedLlama-3-8B-v2.0. Accessed: 2024-11-
02.
Karabacak, M. and Margetis, K. (2023). Embracing large
language models for medical applications: opportuni-
ties and challenges. Cureus, 15(5).
Labrak, Y., Bazoge, A., Morin, E., Gourraud, P.-A., Rou-
vier, M., and Dufour, R. (2024). Biomistral: A collec-
tion of open-source pretrained large language models
for medical domains.
Lewandowski, M., Łukowicz, P.,
´
Swietlik, D., and
Bara
´
nska-Rybak, W. (2023). Chatgpt-3.5 and chatgpt-
4 dermatological knowledge level based on the spe-
cialty certificate examination in dermatology. Clin
Exp Dermatol, page llad255.
Lewis, P., Perez, E., Piktus, A., Petroni, F., Karpukhin, V.,
Goyal, N., Küttler, H., Lewis, M., Yih, W.-t., Rock-
täschel, T., et al. (2020). Retrieval-augmented gen-
eration for knowledge-intensive nlp tasks. Advances
in Neural Information Processing Systems, 33:9459–
9474.
Mestre, A., Muster, M., El Adib, A. R., Egilsdottir, H., By-
ermoen, K., Padilha, M., Aguilar, T., Tabagari, N.,
Betts, L., Sales, L., Garcia, P., Ling, L., Café, H.,
Binnie, A., and Marreiros, A. (2022). The impact of
small-group virtual patient simulator training on per-
ceptions of individual learning process and curricular
integration: a multicentre cohort study of nursing and
medical students. BMC Medical Education, 22.
Nicikowski, J., Szczepa
´
nski, M., Miedziaszczyk, M., and
Kudli
´
nski, B. (2024). The potential of chatgpt in
medicine: an example analysis of nephrology spe-
cialty exams in poland. Clinical Kidney Journal,
17(8):sfae193.
Obuchowski, A., Klaudel, B., and Jasik, P. (2023). Infor-
mation extraction from Polish radiology reports using
language models. In Piskorski, J., Marci
´
nczuk, M.,
Nakov, P., Ogrodniczuk, M., Pollak, S., P
ˇ
ribá
ˇ
n, P.,
Rybak, P., Steinberger, J., and Yangarber, R., editors,
Proceedings of the 9th Workshop on Slavic Natural
Language Processing 2023 (SlavicNLP 2023), pages
113–122, Dubrovnik, Croatia. Association for Com-
putational Linguistics.
OpenMeditron (2024). Meditron3-70b. https://huggingface.
co/OpenMeditron/Meditron3-70B. Accessed: 2024-
11-02.
Optimizing Retrieval-Augmented Generation of Medical Content for Spaced Repetition Learning
185