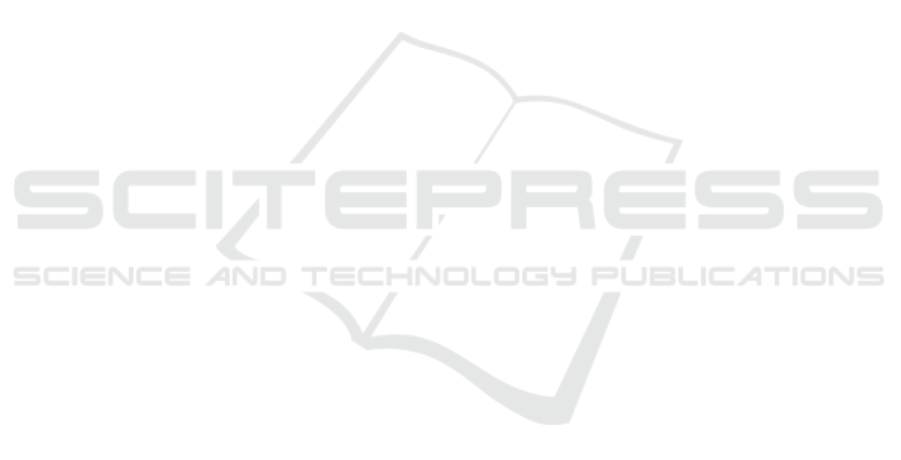
Privacy Protection, pages 124–139. Springer Interna-
tional Publishing.
Earp, J. B., Vail, M., and Anton, A. I. (2007). Privacy pol-
icy representation in web-based healthcare. In 40th
Annual Hawaii International Conference on System
Sciences (HICSS’07), pages 138–138.
European Parliament and Council of the European Union
(2016). Regulation 2016/679 of the European Parlia-
ment and of the Council of 27 April 2016 (General
Data Protection Regulation). Official Journal of the
European Union, pages 1–88.
Fischer-H
¨
ubner, S. and Karegar, F. (2024). Challenges
of Usable Privacy, chapter 4, pages 103–131. Syn-
thesis Lectures on Information Security, Privacy, and
Trust. Springer International Publishing.
Fox, G., Lynn, T., and Rosati, P. (2022). Enhancing con-
sumer perceptions of privacy and trust: a GDPR la-
bel perspective. Information Technology & People,
35(8):181–204.
Gerl, A. (2020). Modelling of a Privacy Language and Effi-
cient Policy-Based De-Identification. PhD thesis, Uni-
versit
¨
at Passau.
Ibdah, D., Lachtar, N., Raparthi, S. M., and Bacha, A.
(2021). ‘‘Why Should I Read the Privacy Policy,
I Just Need the Service”: A study on attitudes and
perceptions toward privacy policies. IEEE Access,
9:166465–166487.
Jesus, V. (2020). Towards an accountable web of per-
sonal information: The web-of-receipts. IEEE Access,
8:25383–25394.
Kaili, M. and Kapitsaki, G. M. (2023). Improving the repre-
sentation choices of privacy policies for end-users. In
WEBIST, pages 42–59. Springer Nature Switzerland.
Kelley, P. G., Cesca, L., Bresee, J., and Cranor, L. F. (2010).
Standardizing privacy notices: an online study of the
nutrition label approach. In SIGCHI, pages 1573–
1582.
Knijnenburg, B. P., Kobsa, A., and Jin, H. (2013). Coun-
teracting the negative effect of form auto-completion
on the privacy calculus. In Thirty Fourth International
Conference on Information Systems. Citeseer.
Leicht, J. and Heisel, M. (2023). P2BAC: Privacy pol-
icy based access control using P-LPL. In 9th Inter-
national Conference on Information Systems Security
and Privacy, pages 686–697. SciTePress.
Leicht, J. and Heisel, M. (2024). Extending PriPoCoG:
A Privacy Policy Editor for GDPR-Compliant Privacy
Policies. In ENASE, pages 307–318.
Leicht, J. and Heisel, M. (2025). Management of cus-
tomized privacy policies. In 11th International Con-
ference on Information Systems Security and Privacy,
volume 2, pages 385–396.
Leicht, J., Heisel, M., and Gerl, A. (2022). PriPoCoG:
Guiding policy authors to define GDPR-compliant
privacy policies. In TrustBus 2022, pages 1–16.
Springer.
Leicht, J., Wagner, M., and Heisel, M. (2023). Creat-
ing privacy policies from data-flow diagrams. In ES-
ORICS 2023 International Workshops, pages 433–
453. Springer Nature Switzerland.
Lipford, H. R., Watson, J., Whitney, M., Froiland, K., and
Reeder, R. W. (2010). Visual vs. compact: A compar-
ison of privacy policy interfaces. In SIGCHI, pages
1111–1114.
Norberg, P. A., Horne, D. R., and Horne, D. A. (2007). The
privacy paradox: Personal information disclosure in-
tentions versus behaviors. Journal of Consumer Af-
fairs, 41(1):100–126.
Papaioannou, T., Tsohou, A., Karyda, M., and Karagiannis,
S. (2022). Requirements for an information privacy
pedagogy based on the constructivism learning theory.
In ARES, pages 1–8. ACM.
Paudel, R., Shrestha, A., Dumaru, P., and Al-Ameen,
M. N. (2023). “it doesn’t just feel like something
a lawyer slapped together.”- mental-model-based pri-
vacy policy for third-party applications on facebook.
In CSCW, page 298–306. ACM.
Proctor, R. W., Ali, M. A., and Vu, K.-P. L. (2008). Exam-
ining usability of web privacy policies. International
Journal of Human-Computer Interaction, 24(3):307–
328.
Raschke, P. and Eichinger, T. (2022). D4.2 - Privacy dash-
boards. Deliverable, TRAPEZE - TRAnsparency, Pri-
vacy and security for European citiZEns. https://bscw.
ercim.eu/pub/bscw.cgi/1274072.
Reinhardt, D., Borchard, J., and Hurtienne, J. (2021). Visual
interactive privacy policy: The better choice? In CHI,
pages 1–12.
Rossi, A. and Palmirani, M. (2019). DaPIS: a data pro-
tection icon set to improve information transparency
under the GDPR. Knowledge of the Law in the Big
Data Age, 252:181–195.
Sailaja, N. and Jones, R. (2017). Industry ideals barriers
in using alternative privacy policies. In HCI, volume
2017-July. BCS Learning and Development Ltd.
Tsolakidou, A., Raptis, G. E., Katsini, C., and Katsanos,
C. (2024). Exploring the impact of cognitive styles
on the visualization of privacy policies. In PCI, page
109–115. ACM.
Windl, M., Ortloff, A.-M., Henze, N., and Schwind, V.
(2022). Privacy at a glance: A process to learn mod-
ular privacy icons during web browsing. In CHIIR,
pages 102–112.
Zimmeck, S., Kuller, E., Ma, C., Tassone, B., and Cham-
peau, J. (2024). Generalizable active privacy choice:
Designing a graphical user interface for global privacy
control. Proceedings on Privacy Enhancing Technolo-
gies.
PriPoCoG: Empowering End-Users’ Data Protection Decisions
679