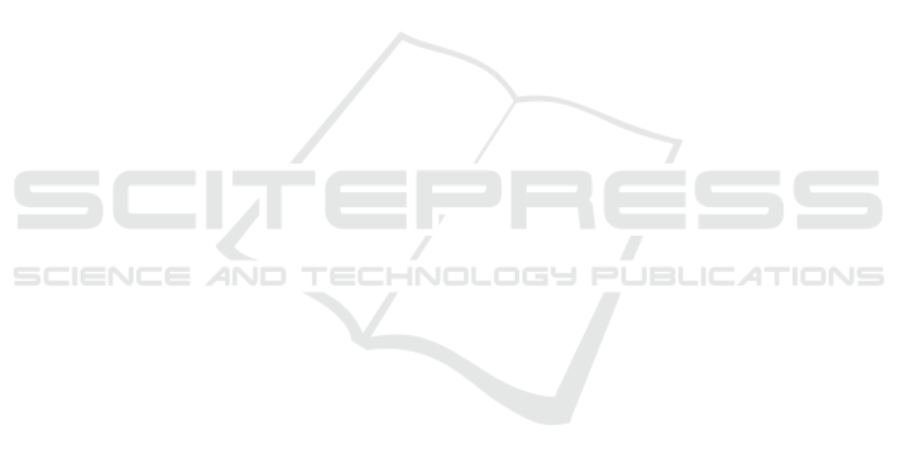
ble for data and verification messages to be sent and
received in a flexible, asynchronous way. The pro-
totype implementation of the solution has been eval-
uated experimentally to analyze the overhead of the
solution as a cost indicator, compared to the bene-
fit of reducing the spread of malicious data. Evalua-
tion results have shown the solution to require ≈ 12%
longer response time per data entity and reduce the
response throughput by ≈ 20%. At the same time, the
results show the ability to accurately detect over 95%
of malicious data, allowing FIWARE to handle them
accordingly.
REFERENCES
Alberti, A. M., Santos, M. A., Souza, R., Da Silva, H.
D. L., Carneiro, J. R., Figueiredo, V. A. C., and Ro-
drigues, J. J. (2019). Platforms for smart environments
and future internet design: A survey. IEEE Access,
7:165748–165778.
Amara, F. Z., Hemam, M., Djezzar, M., and Maimor, M.
(2022). Semantic web and internet of things: Chal-
lenges, applications and perspectives. Journal of ICT
Standardization, 10(2):261–291.
Anwar, F. and Saravanan, S. (2022). Comparison of artifi-
cial intelligence algorithms for iot botnet detection on
apache spark platform. Procedia Computer Science,
215:499–508.
Ataei, M., Eghmazi, A., Shakerian, A., Landry Jr, R., and
Chevrette, G. (2023). Publish/subscribe method for
real-time data processing in massive iot leveraging
blockchain for secured storage. Sensors, 23(24):9692.
Baee, M. A. R., Simpson, L., and Armstrong, W. (2024).
Anomaly detection in the key-management interoper-
ability protocol using metadata. IEEE Open Journal
of the Computer Society.
Barriga, J. A., Clemente, P. J., Hern
´
andez, J., and P
´
erez-
Toledano, M. A. (2022). Simulateiot-fiware: Domain
specific language to design, code generation and ex-
ecute iot simulation environments on fiware. IEEE
Access, 10:7800–7822.
Bellini, P., Palesi, L. A. I., Giovannoni, A., and Nesi, P.
(2023). Managing complexity of data models and per-
formance in broker-based internet/web of things ar-
chitectures. Internet of Things, 23:100834.
Lazidis, A., Tsakos, K., and Petrakis, E. G. (2022). Publish–
subscribe approaches for the iot and the cloud: Func-
tional and performance evaluation of open-source sys-
tems. Internet of Things, 19:100538.
Mart
´
ın, D. G., Florez, S. L., Gonz
´
alez-Briones, A., and Cor-
chado, J. M. (2023). Cosibas platform—cognitive ser-
vices for iot-based scenarios: Application in p2p net-
works for energy exchange. Sensors, 23(2):982.
Martins, I., Resende, J. S., Sousa, P. R., Silva, S., Antunes,
L., and Gama, J. (2022). Host-based ids: A review
and open issues of an anomaly detection system in iot.
Future Generation Computer Systems, 133:95–113.
Munoz-Arcentales, A., L
´
opez-Pernas, S., Conde, J.,
Alonso,
´
A., Salvach
´
ua, J., and Hierro, J. J. (2021).
Enabling context-aware data analytics in smart envi-
ronments: An open source reference implementation.
Sensors, 21(21):7095.
Neto, E. C. P., Dadkhah, S., Ferreira, R., Zohourian, A., Lu,
R., and Ghorbani, A. A. (2023). Ciciot2023: A real-
time dataset and benchmark for large-scale attacks in
iot environment. Sensors, 23(13):5941.
Razzaque, M. A., Milojevic-Jevric, M., Palade, A., and
Clarke, S. (2015). Middleware for internet of things:
a survey. IEEE Internet of things journal, 3(1):70–95.
Shukla, P., Krishna, C. R., and Patil, N. V. (2024). Kafka-
shield: Kafka streams-based distributed detection
scheme for iot traffic-based ddos attacks. Security and
Privacy, 7(6):e416.
Sirisha, A., Chaitanya, K., Krishna, K., and Kanumalli,
S. S. (2021). Intrusion detection models using super-
vised and unsupervised algorithms-a comparative es-
timation. International Journal of Safety and Security
Engineering, 11(1):51–58.
Zyrianoff, I., Heideker, A., Sciullo, L., Kamienski, C., and
Di Felice, M. (2021). Interoperability in open iot plat-
forms: Wot-fiware comparison and integration. In
2021 IEEE International Conference on Smart Com-
puting (SMARTCOMP), pages 169–174. IEEE.
Intelligent Anomaly Detection for Context-Oriented Data Brokerage Systems
449