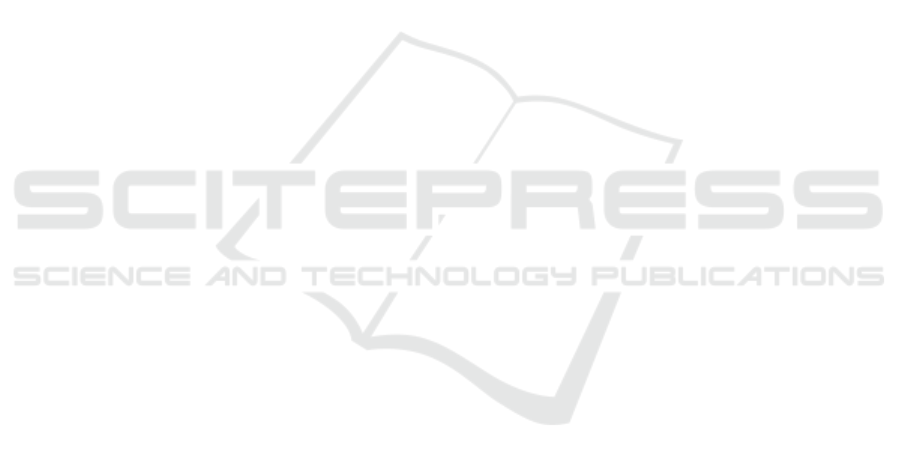
Anxiety via Vehicle-to-Vehicle Social Charging Sys-
tem. In IEEE 85th Vehicular Technology Conference
(VTC Spring), pages 1–5.
Bulut, E., Kisacikoglu, M. C., and Akkaya, K. (2019).
Spatio-Temporal Non-Intrusive Direct V2V Charge
Sharing Coordination. IEEE Transactions on Vehic-
ular Technology, 68(10):9385–9398.
Dhungana, A. and Bulut, E. (2019). Peer-to-peer energy
sharing in mobile networks: Applications, challenges,
and open problems. Ad Hoc Networks, 97:102029.
Foursquare (2024). Foursquare. https://foursquare.com/.
Accessed: 2024-08-26.
Frade, I., Ribeiro, A., Gonßalves, G., and Pais Antunes,
A. (2011). Optimal location of charging stations for
electric vehicles in a neighborhood in lisbon, portu-
gal. TRR, 2252.
International Energy Agency (2019). Global EV Outlook
2019. IEA, Paris. Licence: CC BY 4.0.
International Energy Agency (IEA) (2023). Global ev out-
look 2023. Technical report, IEA, Paris. Licence: CC
BY 4.0.
Kester, J., Sovacool, B. K., Noel, L., and Zarazua de
Rubens, G. (2020). Rethinking the spatiality of nordic
electric vehicles and their popularity in urban environ-
ments: Moving beyond the city? Journal of Transport
Geography, 82:102557.
Kim, O. T. T., Tran, N. H., Nguyen, V., Kang, S. M., and
Hong, C. S. (2018). Cooperative between v2c and
v2v charging: Less range anxiety and more charged
evs. In 2018 International Conference on Information
Networking (ICOIN), pages 679–683.
Kingsford, C. (2019). Maximum bipartite matching. Maxi-
mum Bipartite Matching.
Li, Y., Wu, T., Hui, P., Jin, D., and Chen, S. (2014). Social-
aware d2d communications: Qualitative insights and
quantitative analysis. IEEE Communications Maga-
zine, 52(6):150–158.
Liu, C., Chau, K. T., Wu, D., and Gao, S. (2013). Opportu-
nities and challenges of vehicle-to-home, vehicle-to-
vehicle, and vehicle-to-grid technologies. Proceed-
ings of the IEEE, 101(11):2409–2427.
Lopes, J. A. P., Soares, F. J., and Almeida, P. M. R. (2011).
Integration of electric vehicles in the electric power
system. Proceedings of the IEEE, 99(1):168–183.
Shurrab, M., Singh, S., Otrok, H., Mizouni, R., and Khad-
kikar (2022a). An efficient vehicle-to-vehicle (v2v)
energy sharing framework. IEEE Internet of Things
Journal, 9(7):5315–5328.
Shurrab, M., Singh, S., Otrok, H., Mizouni, R., Khad-
kikar, V., and Zeineldin, H. (2022b). A stable match-
ing game for v2v energy sharing–a user satisfaction
framework. IEEE Transactions on Intelligent Trans-
portation Systems, 23(7):7601–7613.
Tushar, W., Chai, B., Yuen, C., Smith, D. B., Wood, K. L.,
Yang, Z., and Poor, H. V. (2015). Three-party en-
ergy management with distributed energy resources in
smart grid. IEEE Transactions on Industrial Electron-
ics, 62(4):2487–2498.
Ucer, E., Buckreus, R., Kisacikoglu, M. C., Bulut, E., Gu-
ven, M., Sozer, Y., and Giubbolini, L. (2019). A flexi-
ble v2v charger as a new layer of vehicle-grid integra-
tion framework. In 2019 IEEE Transportation Elec-
trification Conference and Expo (ITEC), pages 1–7.
Wang, M., Ismail, M., Zhang, R., Shen, X., Serpedin, E.,
and Qaraqe, K. (2018). Spatio-temporal coordinated
v2v energy swapping strategy for mobile pevs. IEEE
Transactions on Smart Grid, 9(3):1566–1579.
Xiong, Y., Gan, J., An, B., Miao, C., and Bazzan, A. L. C.
(2018). Optimal electric vehicle fast charging sta-
tion placement based on game theoretical framework.
IEEE Transactions on Intelligent Transportation Sys-
tems, 19(8):2493–2504.
Yuan, Y., Jiao, L., Zhu, K., and Zhang, L. (2022). Schedul-
ing online ev charging demand response via v2v auc-
tions and local generation. IEEE Transactions on In-
telligent Transportation Systems, 23(8):11436–11452.
Zhang, R., Cheng, X., and Yang, L. (2017). Stable matching
based cooperative v2v charging mechanism for elec-
tric vehicles. In 2017 IEEE 86th Vehicular Technology
Conference (VTC-Fall), pages 1–5.
Zhang, R., Cheng, X., and Yang, L. (2019). Flexible energy
management protocol for cooperative ev-to-ev charg-
ing. IEEE Transactions on Intelligent Transportation
Systems, 20(1):172–184.
EV-Connect: Energy Efficient & Incentive Cost Based Model for Range Anxious EVs with Multi-Hop Socially Assisted V2V Charging
171