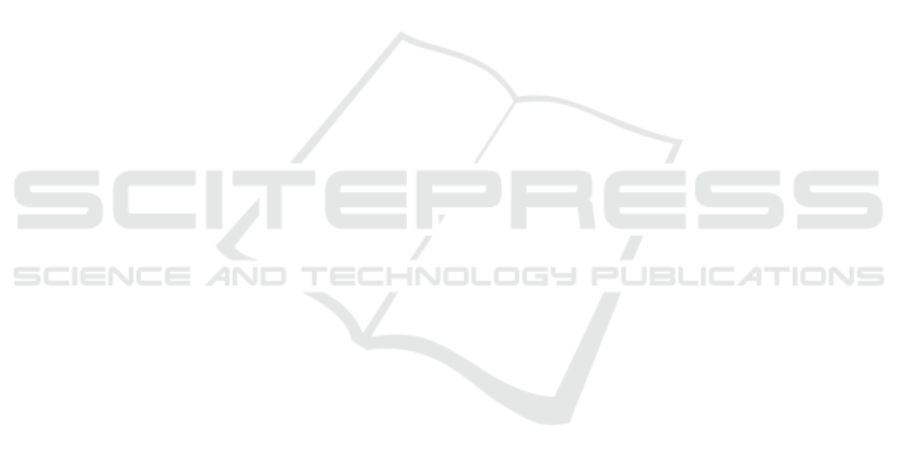
emerged as a compelling alternative for edge deploy-
ment due to its significantly faster inference times and
close performance to LSTM, making DNN a better
candidate for edge implementation.
Next steps include further evaluation of the PGDT
developed in this study using synthetic data and
its integration with multi-objective optimization and
techno-economic analysis components. First, we
will develop a synthetic dataset using the greenhouse
dataset described in this paper as training material
with generative AI algorithms such as generative
adversarial networks and variational auto-encoders
and evaluate the reliance of the emerging forecasting
model using metrics such as discriminative and pre-
dictive scores (Yoon et al., 2019) (Desai et al., 2021).
Then, we will select the emerging best-in-class fore-
casting model as input to multi-objective optimization
to develop a framework that helps farmers identify
optimal resource trade-offs in securing robust crop
yields following the approach described in (Sanfil-
ippo et al., 2024). Finally, we will evaluate the eco-
nomic sustainability of optimimal trade-off scenar-
ios through techno-economic analysis, as discussed in
(Sanfilippo et al., 2024).
ACKNOWLEDGEMENTS
The authors would like to acknowledge Nasser Al-
Khalaf, Jovelyn Beltran, and Mohamed Batran at
AGRICO for their collaboration with data and setup
to conduct the study and test the systems. This
work was supported by the Ministry of Municipal-
ity and Environment (MME) under Grant MME02-
0911-200015 from the Qatar National Research Fund
(a member of Qatar Foundation) / QRDIC. The find-
ings herein reflect the work and are solely the respon-
sibility of the authors.
REFERENCES
Alhnaity, B., Pearson, S., Leontidis, G., and Kollias, S.
(2019). Using deep learning to predict plant growth
and yield in greenhouse environments. In Inter-
national Symposium on Advanced Technologies and
Management for Innovative Greenhouses: Green-
Sys2019 1296, pages 425–432.
Chen, T. and Guestrin, C. (2016). Xgboost: A scalable
tree boosting system. In Proceedings of the 22nd acm
sigkdd international conference on knowledge discov-
ery and data mining, pages 785–794.
Desai, A., Freeman, C., Wang, Z., and Beaver, I.
(2021). Timevae: A variational auto-encoder for
multivariate time series generation. arXiv preprint
arXiv:2111.08095.
Ehret, D. L., Hill, B. D., Helmer, T., and Edwards, D. R.
(2011). Neural network modeling of greenhouse
tomato yield, growth and water use from automated
crop monitoring data. Computers and electronics in
agriculture, 79(1):82–89.
Gong, L., Yu, M., Jiang, S., Cutsuridis, V., and Pearson,
S. (2021). Deep learning based prediction on green-
house crop yield combined tcn and rnn. Sensors,
21(13):4537.
Hochreiter, S. (1997). Long short-term memory. Neural
Computation MIT-Press.
Huang, J., Zhang, G., Zhang, Y., Guan, X., Wei, Y., and
Guo, R. (2020). Global desertification vulnerability to
climate change and human activities. Land Degrada-
tion & Development, 31(11):1380–1391.
Iba, W. and Langley, P. (1992). Induction of one-level de-
cision trees. In Machine learning proceedings 1992,
pages 233–240. Elsevier.
Planning, Q. and Authority, S. (2021). Qatar voluntary na-
tional review 2021. https://sustainabledevelopment.
un.org/memberstates/qatar.
Qaddoum, K., Hines, E., and Iliescu, D. (2013). Yield pre-
diction for tomato greenhouse using efunn. Interna-
tional Scholarly Research Notices, 2013(1):430986.
Sanfilippo, A., Kafi, A., Jovanovic, R., Ahmad, N., Wanik,
Z., et al. (2024). Sustainable energy management for
indoor farming in hot desert climates. Sustainable En-
ergy Technologies and Assessments, 71:103958.
Sivakumar, M. V. (2007). Interactions between climate and
desertification. Agricultural and forest meteorology,
142(2-4):143–155.
Sze, V., Chen, Y.-H., Yang, T.-J., and Emer, J. S. (2017). Ef-
ficient processing of deep neural networks: A tutorial
and survey. Proceedings of the IEEE, 105(12):2295–
2329.
Vanthoor, B., De Visser, P., Stanghellini, C., and Van Hen-
ten, E. J. (2011a). A methodology for model-based
greenhouse design: Part 2, description and validation
of a tomato yield model. Biosystems engineering,
110(4):378–395.
Vanthoor, B., Stanghellini, C., Van Henten, E. J., and
De Visser, P. (2011b). A methodology for model-
based greenhouse design: Part 1, a greenhouse cli-
mate model for a broad range of designs and climates.
Biosystems Engineering, 110(4):363–377.
Yoon, J., Jarrett, D., and Van der Schaar, M. (2019). Time-
series generative adversarial networks. Advances in
neural information processing systems, 32.
A Predictive Greenhouse Digital Twin for Controlled Environment Agriculture
987