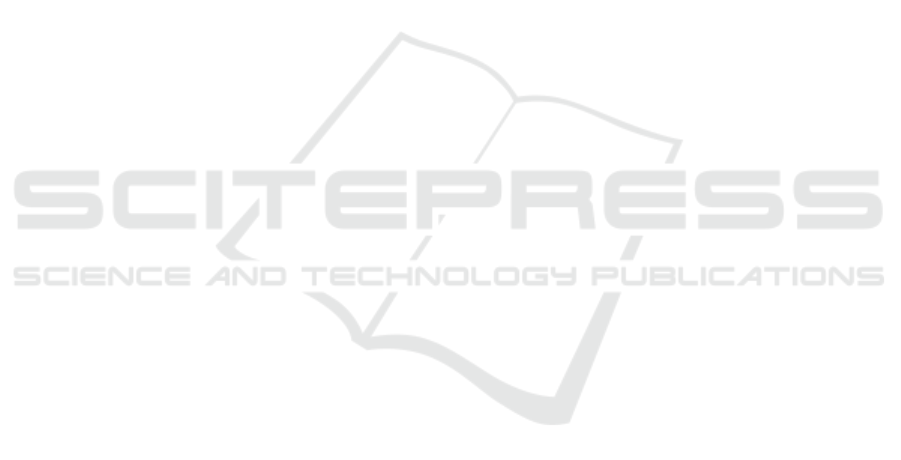
Out, pages 74–81, Barcelona, Spain. Association for
Computational Linguistics.
Liu, Y., Ott, M., Goyal, N., Du, J., Joshi, M., Chen, D.,
Levy, O., Lewis, M., Zettlemoyer, L., and Stoyanov,
V. (2019). Roberta: A robustly optimized bert pre-
training approach. arXiv preprint arXiv:1907.11692.
Miftakhova, A. and Yapparova, V. (2019). The game as a
necessary component of e-learning materials for chil-
dren. EDULEARN19 Proceedings.
Munoz, H. T., Baldiris, S., and Fabregat, R. (2016). Co de-
sign of augmented reality game-based learning games
with teachers using co-creaargbl method. 2016 IEEE
16th International Conference on Advanced Learning
Technologies (ICALT), pages 120–122.
Narayan, S. and Gardent, C. (2014). Hybrid simplification
using deep semantics and machine translation. In The
52nd annual meeting of the association for computa-
tional linguistics, pages 435–445.
Padilla-Zea, N., Vela, F. G., Medina-Medina, N., and
Gonz
´
alez, C. (2018). Involving teachers in the edu-
cational video games design process. pages 152–157.
Praveen Kumar, A., Nayak, A., Shenoy K., M., Manoj,
R. J., and Priyadarshi, A. (2022). Pattern-based syn-
tactic simplification of compound and complex sen-
tences. IEEE Access, 10:53290–53306.
Radford, A., Wu, J., Child, R., Luan, D., Amodei, D.,
Sutskever, I., et al. (2019). Language models are un-
supervised multitask learners. OpenAI blog, 1(8):9.
Raffel, C., Shazeer, N., Roberts, A., Lee, K., Narang, S.,
Matena, M., Zhou, Y., Li, W., and Liu, P. J. (2020).
Exploring the limits of transfer learning with a unified
text-to-text transformer. Journal of machine learning
research, 21(140):1–67.
Rice, J. W. (2007). New media resistance: Barriers to im-
plementation of computer video games in the class-
room. Journal of Educational Multimedia and Hyper-
media, 16(3):249–261.
Saur
´
ı, R., Littman, J., Knippen, B., Gaizauskas, R., Set-
zer, A., and Pustejovsky, J. (2006). Timeml annotation
guidelines. Version, 1(1):31.
Smaldone, R. A., Thompson, C., Evans, M. J., and Voit,
W. (2017). Teaching science through video games.
Nature chemistry, 9 2:97–102.
Speer, R., Chin, J., and Havasi, C. (2017). Conceptnet 5.5:
An open multilingual graph of general knowledge. In
Proceedings of the AAAI conference on artificial intel-
ligence, volume 31.
Sunday, K., Oyelere, S., Agbo, F., Aliyu, M., Balogun,
O., and Bouali, N. (2023). Usability evaluation of
imikode virtual reality game to facilitate learning of
object-oriented programming. Technology, Knowl-
edge and Learning, 28:1871–1902. Publisher Copy-
right: © 2022, The Author(s).
Tazouti, Y. (2020). A virtual reality serious game for lan-
guage learning. International Journal of Advanced
Trends in Computer Science and Engineering.
Walsh, G. (2012). Employing co-design in the video game
design process. In Handbook of Research on Serious
Games as Educational, Business and Research Tools,
pages 1048–1063. IGI Global.
Wang, T., Chen, P., Rochford, J., and Qiang, J. (2016). Text
simplification using neural machine translation. Pro-
ceedings of the AAAI Conference on Artificial Intelli-
gence, 30(1).
Wubben, S., van den Bosch, A., and Krahmer, E.
(2012). Sentence simplification by monolingual ma-
chine translation. In Li, H., Lin, C.-Y., Osborne, M.,
Lee, G. G., and Park, J. C., editors, Proceedings of the
50th Annual Meeting of the Association for Compu-
tational Linguistics (Volume 1: Long Papers), pages
1015–1024, Jeju Island, Korea. Association for Com-
putational Linguistics.
Zhang, T., Kishore, V., Wu, F., Weinberger, K. Q., and
Artzi, Y. (2019). Bertscore: Evaluating text genera-
tion with bert. arXiv preprint arXiv:1904.09675.
Teacher in the Loop: Customizing Educational Games Using Natural Language
457