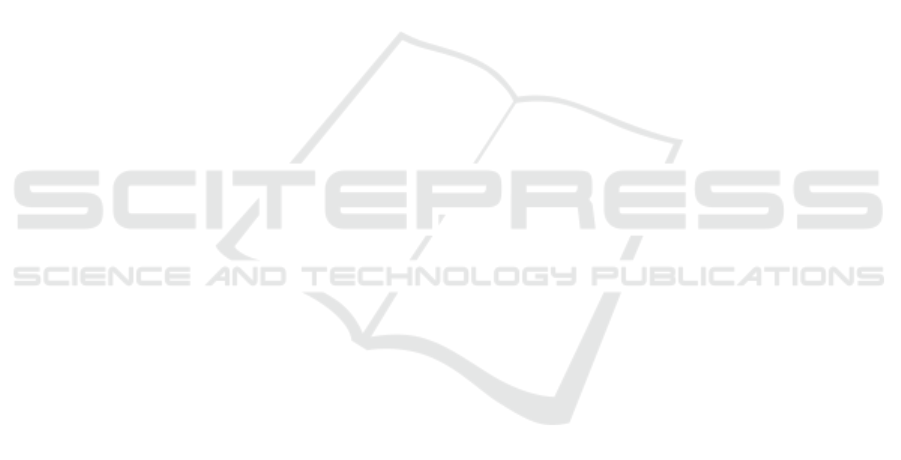
ACKNOWLEDGMENTS
The results presented in this paper have been devel-
oped as part of a project at SiDi, financed by Samsung
Eletr
ˆ
onica da Amazonia Ltda., under the auspices of
the Brazilian Federal Law of Informatics no. 8248/9.
REFERENCES
Alhamad, H. A., Shehab, M., Shambour, M. K. Y., Abu-
Hashem, M. A., Abuthawabeh, A., Al-Aqrabi, H.,
Daoud, M. S., and Shannaq, F. B. (2024). Handwrit-
ten recognition techniques: A comprehensive review.
Symmetry, 16(6):681.
Almazrouei, E., Alobeidli, H., Alshamsi, A., Cappelli, A.,
Cojocaru, R., Debbah, M., Goffinet,
´
E., Hesslow,
D., Launay, J., Malartic, Q., et al. (2023). The fal-
con series of open language models. arXiv preprint
arXiv:2311.16867.
Baek, Y., Lee, B., Han, D., Yun, S., and Lee, H. (2019).
Character region awareness for text detection. In Pro-
ceedings of the IEEE Conference on Computer Vision
and Pattern Recognition, pages 9365–9374.
Beltran, A. (2023). Fiscal data in text: Information ex-
traction from audit reports using natural language pro-
cessing. Data & Policy, 5:e7.
de Elias, E. M., Tasinaffo, P. M., and Hirata, R. (2019).
Alignment, scale and skew correction for optical mark
recognition documents based. In 2019 XV Workshop
de Vis
˜
ao Computacional (WVC), pages 26–31. IEEE.
de Vos, I. M. A., Boogerd, G. L., Fennema, M. D., and Cor-
reia, A. D. (2022). Comparing in context: Improving
cosine similarity measures with a metric tensor. arXiv
preprint arXiv:2203.14996.
Devlin, J. (2018). Bert: Pre-training of deep bidirec-
tional transformers for language understanding. arXiv
preprint arXiv:1810.04805.
Fisher, I. E., Garnsey, M. R., and Hughes, M. E. (2016).
Natural language processing in accounting, auditing
and finance: A synthesis of the literature with a
roadmap for future research. Intelligent Systems in Ac-
counting, Finance and Management, 23(3):157–214.
Grabb, D. (2023). The impact of prompt engineering in
large language model performance: a psychiatric ex-
ample. Journal of Medical Artificial Intelligence, 6.
Harley, A. W., Ufkes, A., and Derpanis, K. G. (2015). Eval-
uation of deep convolutional nets for document image
classification and retrieval. CoRR, abs/1502.07058.
Hegghammer, T. (2022). Ocr with tesseract, amazon tex-
tract, and google document ai: a benchmarking ex-
periment. Journal of Computational Social Science,
5(1):861–882.
Huang, A. et al. (2008). Similarity measures for text doc-
ument clustering. In Proceedings of the sixth new
zealand computer science research student conference
(NZCSRSC2008), Christchurch, New Zealand, vol-
ume 4, pages 9–56.
HuggingFace (2024). Falcon-7b model card. https://
huggingface.co/tiiuae/falcon-7b. Accessed: 2024-11-
08.
Ingle, R. R., Fujii, Y., Deselaers, T., Baccash, J., and Popat,
A. C. (2019). A scalable handwritten text recognition
system. In 2019 International conference on docu-
ment analysis and recognition (ICDAR), pages 17–24.
IEEE.
Karanikolas, N., Manga, E., Samaridi, N., Tousidou, E.,
and Vassilakopoulos, M. (2023). Large language mod-
els versus natural language understanding and genera-
tion. In Proceedings of the 27th Pan-Hellenic Con-
ference on Progress in Computing and Informatics,
pages 278–290.
Kumar, P. (2024). Large language models (llms): survey,
technical frameworks, and future challenges. Artificial
Intelligence Review, 57(10):260.
MetaAI (2024). Llama 2-7b model card. https://
huggingface.co/meta-llama/Llama-2-7b. Accessed:
2024-11-08.
Papineni, K., Roukos, S., Ward, T., and Zhu, W.-J. (2002).
Bleu: a method for automatic evaluation of machine
translation. In Proceedings of the 40th annual meet-
ing of the Association for Computational Linguistics,
pages 311–318.
Rud
ˇ
zionis, V., Lopata, A., Gudas, S., Butleris, R., Veitait
˙
e,
I., Dilijonas, D., Gri
ˇ
sius, E., Zwitserloot, M., and
Rudzioniene, K. (2022). Identifying irregular finan-
cial operations using accountant comments and natu-
ral language processing techniques. Applied sciences,
12(17):8558.
Saini, R. (2015). Document image binarization tech-
niques, developments and related issues: a re-
view. International Journal of Computer Applica-
tions, 116(7):0975–8887.
Santana, A. F. B., de Faria, J. A., and Sena, T. R. (2024).
Editorial volume 05, n
´
umero 02, 2024.: Auditoria e
seus desafios (ainda) atuais! Revista Controladoria e
Gest
˜
ao, 5(2):1–3.
Simunic, D. A. (1980). The pricing of audit services: The-
ory and evidence. Journal of accounting research,
pages 161–190.
Touvron, H., Martin, L., Stone, K., Albert, P., Almahairi,
A., Babaei, Y., Bashlykov, N., Batra, S., Bhargava,
P., Bhosale, S., et al. (2023). Llama 2: Open foun-
dation and fine-tuned chat models. arXiv preprint
arXiv:2307.09288.
Wang, L., Chen, X., Deng, X., Wen, H., You, M., Liu, W.,
Li, Q., and Li, J. (2024). Prompt engineering in con-
sistency and reliability with the evidence-based guide-
line for llms. npj Digital Medicine, 7(1):41.
Yuan, S. and F
¨
arber, M. (2023). Evaluating generative
models for graph-to-text generation. arXiv preprint
arXiv:2307.14712.
Zheng, X., Zhang, C., and Woodland, P. C. (2021). Adapt-
ing gpt, gpt-2 and bert language models for speech
recognition. In 2021 IEEE Automatic speech recogni-
tion and understanding workshop (ASRU), pages 162–
168. IEEE.
Using Large Language Models to Support the Audit Process in the Accountability of Interim Managers in Notary Offices
995