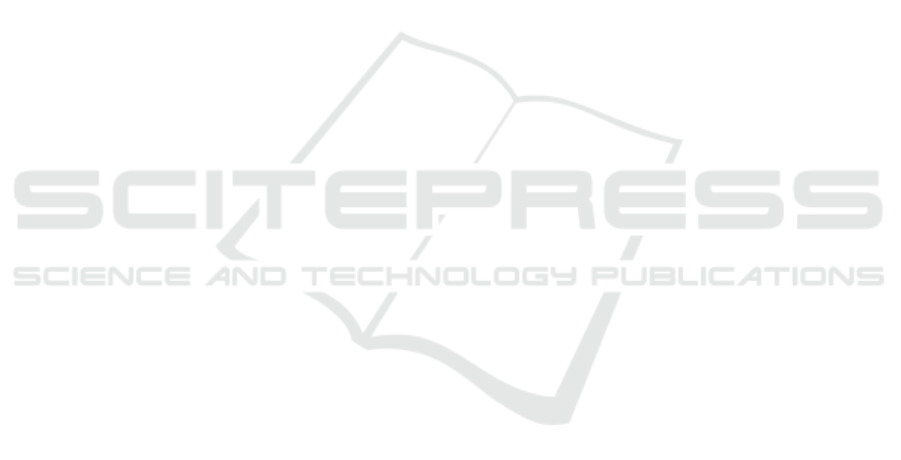
The results presented provide valuable insights for
the development of more efficient models in the con-
text of the Brazilian financial market, and future stud-
ies could explore the application of other attribute se-
lection methods or the adaptation of the methodology
in different financial scenarios.
For future works, we intend to explore the poten-
tial of the selected features for new analyses, lever-
aging these optimized features in advanced machine
learning models, such as deep learning architectures,
to enhance prediction accuracy.
ACKNOWLEDGEMENTS
The authors would like to thank FAPERGS
(24/2551-0001396-2, 23/2551-0000773-8), CNPq
(305805/2021-5) and FAPERGS/CNPq (23/ 2551-
0000126-8). Fabian thanks to Fesurv-UniRV for the
pay leave, which helped to collaborate in this work.
REFERENCES
Aha, D. W. and Bankert, R. L. (1995). A comparative eval-
uation of sequential feature selection algorithms. In
Pre-proceedings of the Fifth International Workshop
on Artificial Intelligence and Statistics.
Altman, D. G. and Bland, J. M. (2005). Standard deviations
and standard errors. In Bmj. British Medical Journal
Publishing Group.
Araujo, G. S. and Gaglianone, W. P. (2023). Machine learn-
ing methods for inflation forecasting in brazil: New
contenders versus classical models. In Latin Ameri-
can Journal of Central Banking. Elsevier.
Bansal, S. (2016). Investigating the efficacy of rsi in the
nifty 50 index. In Global journal of Business and In-
tegral Security.
Billah, M. M., Sultana, A., Bhuiyan, F., and Kaosar, M. G.
(2024). Stock price prediction: comparison of dif-
ferent moving average techniques using deep learn-
ing model. In Neural Computing and Applications.
Springer.
Bouri, E., Demirer, R., Gupta, R., and Sun, X. (2020).
The predictability of stock market volatility in emerg-
ing economies: Relative roles of local, regional, and
global business cycles. In Journal of Forecasting. Wi-
ley Online Library.
Breiman, L. (2001). Random forests. In Machine learning.
Springer.
Cardoso, F. C., Malska, J. A. V., Ramiro, P. J., Lucca, G.,
Borges, E. N., de Mattos, V. L. D., and Berri, R. A.
(2022). Bovdb: a data set of stock prices of all com-
panies in b3 from 1995 to 2020. In Journal of Infor-
mation and Data Management.
Chandrashekar, G. and Sahin, F. (2014). A survey on fea-
ture selection methods. In Computers & electrical en-
gineering. Elsevier.
Chen, C.-P. and Metghalchi, M. (2012). Weak-form market
efficiency: Evidence from the brazilian stock market.
In International Journal of Economics and Finance.
Citeseer.
Cuervo, R. (2023). Predictive ai for sme and large enterprise
financial performance management. In arXiv preprint
arXiv:2311.05840.
Dash, R. K., Nguyen, T. N., Cengiz, K., and Sharma, A.
(2023). Fine-tuned support vector regression model
for stock predictions. In Neural Computing and Ap-
plications. Springer.
Halilbegovic, S. (2016). Macd-analysis of weaknesses of
the most powerful technical analysis tool. In Indepen-
dent Journal of Management & Production. Instituto
Federal de Educac¸
˜
ao, Ci
ˆ
encia e Tecnologia de S
˜
ao
Paulo.
Htun, H. H., Biehl, M., and Petkov, N. (2023). Survey of
feature selection and extraction techniques for stock
market prediction. In Financial Innovation. Springer.
Jain, R. and Vanzara, R. (2023). Emerging trends in
ai-based stock market prediction: A comprehensive
and systematic review. In Engineering Proceedings.
MDPI.
Janecek, A., Gansterer, W., Demel, M., and Ecker, G.
(2008). On the relationship between feature selection
and classification accuracy. In New challenges for fea-
ture selection in data mining and knowledge discov-
ery. PMLR.
Kamalov, F., Smail, L., and Gurrib, I. (2019). Stock
price prediction using technical indicators: a predic-
tive model using optimal deep learning. In Learning.
Kamalov, F., Smail, L., and Gurrib, I. (2020). Forecasting
with deep learning: S&p 500 index. In 2020 13th In-
ternational Symposium on Computational Intelligence
and Design (ISCID). IEEE.
Kang, B.-K. (2021). Improving macd technical analysis by
optimizing parameters and modifying trading rules:
evidence from the japanese nikkei 225 futures market.
In Journal of Risk and Financial Management. MDPI.
Kohn, K. and Moraes, C. d. (2007). O impacto das
novas tecnologias na sociedade: conceitos e carac-
ter
´
ısticas da sociedade da informac¸
˜
ao e da sociedade
digital. In XXX Congresso Brasileiro de Ci
ˆ
encias da
Comunicac¸
˜
ao.
Shi, Y., Li, B., Long, W., and Dai, W. (2022). Method for
improving the performance of technical analysis indi-
cators by neural network models. In Computational
Economics. Springer.
Souza, A. S., Lucca, G., Borges, E. N., Cardoso, F. C., Dal-
mazo, B. L., and Berri, R. (2024). Dataset for Intraday
Analysis of B3 stock prices.
Urbanowicz, R. J., Olson, R. S., Schmitt, P., Meeker, M.,
and Moore, J. H. (2018). Benchmarking relief-based
feature selection methods for bioinformatics data min-
ing. In Journal of biomedical informatics. Elsevier.
Yu, L. and Liu, H. (2016). Feature selection for high-
dimensional data: A fast correlation-based filter so-
lution. In Proceedings of the 20th international con-
ference on machine learning (ICML-03).
Feature Selection for Stock Market Prediction: A Comparison of Relief and Information Gain Methods
1003