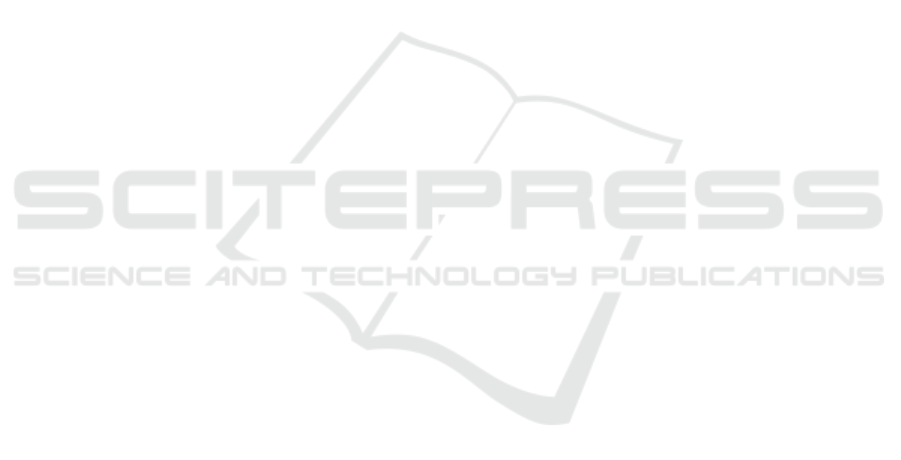
REFERENCES
Bellman, R. (1958). On a routing problem. Quarterly of
Applied Mathematics, 16(1):87–90.
Dijkstra, E. W. (1959). A note on two problems in connex-
ion with graphs. Numerische Mathematik, 1:269–271.
Falcione, S., Campbell, E., McCollum, B., Chamberlain, J.,
Macias, M., Morsch, L., and Pinder, C. (2019). Emer-
gence of different perspectives of success in collabo-
rative learning. Canadian Journal for the Scholarship
of Teaching and Learning, 10(2):n2.
Floyd, R. W. (1962). Algorithm 97: Shortest path. Commu-
nications of the ACM, 5(6):345.
Gny
´
s, P. (2017). Mereogeometry based approach for be-
havioral robotics. In Rough Sets: International Joint
Conference, IJCRS 2017, Olsztyn, Poland, July 3–7,
2017, Proceedings, Part II, pages 70–80. Springer.
Hamming, R. W. (1950). Error detecting and error cor-
recting codes. The Bell system technical journal,
29(2):147–160.
Hart, P. E., Nilsson, N. J., and Raphael, B. (1968). A for-
mal basis for the heuristic determination of minimum
cost paths. IEEE Transactions on Systems Science and
Cybernetics, 4(2):100–107.
Johnson, D. B. (1977). Efficient algorithms for short-
est paths in sparse networks. Journal of the ACM,
24(1):1–13.
Komorowski, J., Pawlak, Z., Polkowski, L., and Skowron,
A. (1999). Rough sets: A tutorial. Rough fuzzy hy-
bridization: A new trend in decision-making, pages
3–98.
Korf, R. E. (1985). Depth-first iterative-deepening: An op-
timal admissible tree search. Artificial Intelligence,
27(1):97–109.
Moore, E. F. (1959). The shortest path through a maze. Pro-
ceedings of the International Symposium on the The-
ory of Switching, pages 285–292.
Nurdin, S. and Setiawan, W. (2016). Improving students’
cognitive abilities and creative thinking skills on tem-
perature and heat concepts through an exelearning-
assisted problem based learning. International Jour-
nal of Scientific & Technology Research, 5(12):59–63.
Osmialowski, P. (2011). Planning and navigation for mobile
autonomous robots spatial reasoning in player. Stage
System.
O
´
smiałowski, P. and Polkowski, L. (2010). Spatial reason-
ing based on rough mereology: a notion of a robot for-
mation and path planning problem for formations of
mobile autonomous robots. In Transactions on rough
sets XII, pages 143–169.
Othman, M. and Zaın, N. (2015). Online collaboration
for programming: Assessing students’ cognitive abil-
ities. Turkish Online Journal of Distance Education,
16(4):84–97.
Pawlak, Z. (1992). Rough sets: Theoretical aspects of rea-
soning about data.
Planas-Llad
´
o, A., Feliu, L., Arbat, G., Pujol, J., Su
˜
nol, J. J.,
Castro, F., and Mart
´
ı, C. (2021). An analysis of team-
work based on self and peer evaluation in higher edu-
cation. Assessment and Evaluation in Higher Educa-
tion, 46(2):191–207.
Polkowski, L. and O
´
smiałowski, P. (2008). A framework for
multiagent mobile robotics: Spatial reasoning based
on rough mereology in player/stage system. In Chan,
C.-C., Grzymala-Busse, J. W., and Ziarko, W. P., ed-
itors, Rough Sets and Current Trends in Computing,
pages 142–149, Berlin, Heidelberg. Springer Berlin
Heidelberg.
Polkowski, L. and O
´
smiałowski, P. (2008). A framework for
multiagent mobile robotics: Spatial reasoning based
on rough mereology in player/stage system. In Rough
Sets and Current Trends in Computing: 6th Inter-
national Conference, RSCTC 2008 Akron, OH, USA,
October 23-25, 2008 Proceedings 6, pages 142–149.
Springer.
Polkowski, L. and Skowron, A. (1996). Rough mereology:
A new paradigm for approximate reasoning. Interna-
tional Journal of Approximate Reasoning, 15(4):333–
365.
Polkowski, L., Zmudzinski, L., and Artiemjew, P. (2018).
Robot navigation and path planning by means of
rough mereology. In 2018 Second IEEE International
Conference on Robotic Computing (IRC), pages 363–
368. IEEE.
Szpakowska, A., Artiemjew, P., and Cybowski, W. (2023).
Navigational strategies for mobile robots using rough
mereological potential fields and weighted distance to
goal. In International Joint Conference on Rough Sets,
pages 549–564. Springer.
Tarjan, R. E. (1972). Depth-first search and linear graph
algorithms. SIAM Journal on Computing, 1(2):146–
160.
Taxirovna, A. S. (2024). Collaborative learning: Fo-
cusing on techniques that promote teamwork and
shared knowledge among students. SO ‘NGI ILMIY
TADQIQOTLAR NAZARIYASI, 7(1):118–123.
Waggener, B. and Waggener, W. N. (1995). Pulse code
modulation techniques. Springer Science & Business
Media.
Zmudzinski, L. and Artiemjew, P. (2017). Path planning
based on potential fields from rough mereology. In
Rough Sets: International Joint Conference, IJCRS
2017, Olsztyn, Poland, July 3–7, 2017, Proceedings,
Part II, pages 158–168. Springer.
Training Telemedicine Robots: A Path Planning Optimization Method for Educational and Medical Application
157