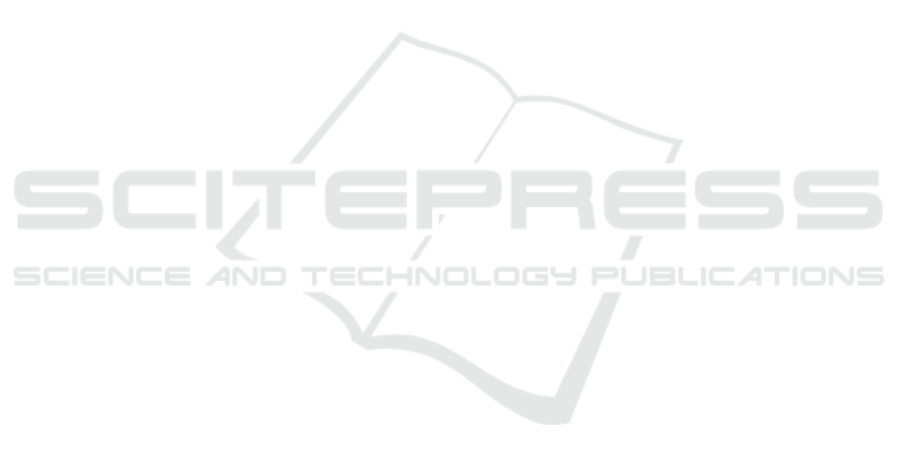
these strategies makes it difficult to verify the correct-
ness of quantitative properties or simulate both de-
sired and unwanted behaviors and this is what limits
their applicability and effectiveness in real scenarios.
8 CONCLUSION
In this paper, we proposed a formal solution for
designing, implementing, and verifying Time-Aware
SoS workflows, focusing on managing mission be-
haviors constrained by temporal conditions. We intro-
duced a set of rewriting rules to specify and prioritize
primary and alternative missions based on quantita-
tive properties and constraints. These rules are gov-
erned by strategies that enforce desired behaviors and
avoid unwanted ones, ensuring the efficient execution
of workflows. The approach was validated through a
case study of the French Emergency SoS, using the
Maude Strategy language for formal verification.
For future work, we aim to extend the formaliza-
tion and verification of self-* properties (e.g., self-
adaptation, self-awareness) to support a broader range
of predictable and unpredictable workflows. Addi-
tionally, we plan to enhance resource prediction and
mission performance evaluation, providing SoSs with
improved tools for planning and optimizing architec-
tures to achieve better performance, cost efficiency,
and resource optimization.
REFERENCES
Dridi, C. E., Hameurlain, N., and Belala, F. (2022). A
maude-based rewriting approach to model and control
system-of-systems’ resources allocation. In Advances
in Model and Data Engineering in the Digitalization
Era - MEDI-DETECT 2022 Workshop Proceedings,
volume 1751 of Communications in Computer and In-
formation Science, pages 207–221. Springer.
Dridi, C. E., Hameurlain, N., and Belala, F. (2023). A
maude-based formal approach to control and analyze
time-resource aware missioned systems-of-systems.
In 31th IEEE International Conference on Enabling
Technologies: Infrastructure for Collaborative Enter-
prises (WETICE-2023). IEEE.
Gassara, A., Rodriguez, I. B. Jmaiel, M., and Drira, K.
(2017). A bigraphical multi-scale modeling method-
ology for system of systems. Computers & Electrical
Engineering, 58:113–125.
Gassara, A. Bouassida, I. and Jmaiel, M. (2017). A tool
for modeling sos architectures using bigraphs. In Pro-
ceedings of the Symposium on Applied Computing,
pages 1787–1792.
Halima, R. B., Klai, K., Sellami, M., and Maamar,
Z. (2021). Formal modeling and verification of
property-based resource consumption cycles. In 2021
IEEE International Conference on Services Comput-
ing (SCC), pages 370–375. IEEE.
Maamar, Z., Faci, N., Sakr, S., Boukhebouze, M., and Bar-
nawi, A. (2016). Network-based social coordination
of business processes. Information Systems, 58:56–
74.
Maier, M. W. (1998). Architecting principles for systems-
of-systems. Systems Engineering: The Journal of
the International Council on Systems Engineering,
1(4):267–284.
Mart
´
ı-Oliet, N. and Meseguer, J. (1996). Rewriting logic as
a logical and semantic framework. Electronic Notes
in Theoretical Computer Science, 4:190–225.
Morrison, R. and Kirby, G. Balasubramaniam, D. e. a.
(2004). Support for evolving software architectures
in the archware adl. Proceedings. Fourth Work-
ing IEEE/IFIP Conference on Software Architecture
(WICSA 2004), pages 69–78.
Oquendo, F. (2016a). Formally describing the archi-
tectural behavior of software-intensive systems-of-
systems with sosadl. In 2016 21st International Con-
ference on Engineering of Complex Computer Systems
(ICECCS), pages 13–22. IEEE.
Oquendo, F. (2016b). Formally describing the software
architecture of systems-of-systems with sosadl. In
2016 11th system of systems engineering conference
(SoSE), pages 1–6. IEEE.
Petitdemange, F., Borne, I., and Buisson, J. (2018). Mod-
eling system of systems configurations. In 2018 13th
Annual Conference on System of Systems Engineering
(SoSE), pages 392–399. IEEE.
Rubio, R., Mart
´
ı-Oliet, N., Pita, I., and Verdejo, A. (2021).
Strategies, model checking and branching-time prop-
erties in maude. Journal of Logical and Algebraic
Methods in Programming, 123:100700.
Seghiri, A., Hameurlain, N., and Belala, F. (2022). A for-
mal language for modelling and verifying systems-of-
systems software architectures. International Journal
of Systems and Service-Oriented Engineering (IJS-
SOE), 12(1):1–17.
Silva, E., Batista, T., and Oquendo, F. (2020). On the
verification of mission-related properties in software-
intensive systems-of-systems architectural design.
Science of Computer Programming, 192:102425.
Stary, C. and Wachholder, D. (2016). System-of-systems
support—a bigraph approach to interoperability and
emergent behavior. Data & Knowledge Engineering,
105:155–172.
¨
Olveczky, P. C. (2004). Real-time maude 2.3
manual. Technical report, Research Report.
http://urn.nb.no/URN:NBN:no-35645.
Maude Strategies-Based SoSs Workflow Modeling
327