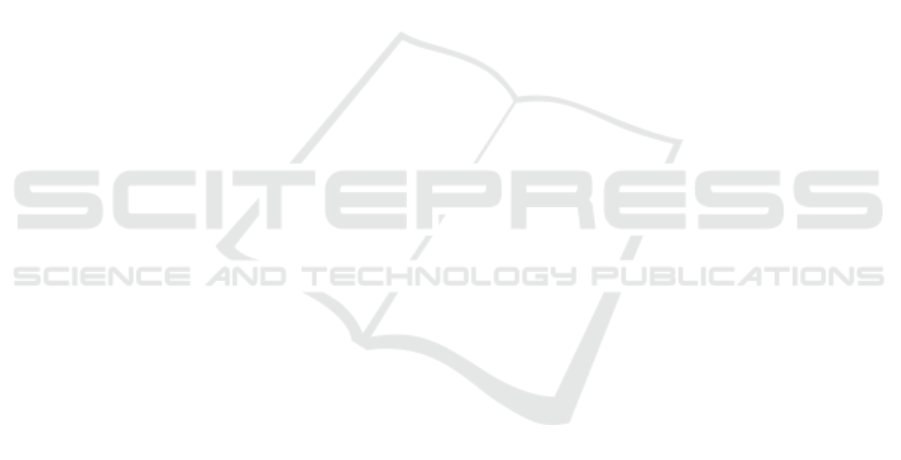
and mean absolute errors below 4 points on a 40-point
scale.
Claude and GPT emerged as the most reliable
automated graders, demonstrating strong consistency
with human evaluation patterns across multiple met-
rics. Their performance suggests that, when provided
with well-structured inputs and clear grading criteria,
LLMs can effectively assess even subjective aspects
of software design exercises. The semantic similar-
ity approach, while less sophisticated, showed mod-
erate effectiveness, indicating potential for simpler
automated solutions in specific contexts. However,
Llama’s significantly lower performance highlights
that not all language models are equally suited for this
task.
These findings have important implications for
scaling software engineering education. By demon-
strating that LLMs can reliably grade UML diagrams
when working with carefully constrained case stud-
ies and clear rubrics, we open new possibilities for
managing larger student cohorts without compromis-
ing assessment quality. However, we emphasize that
these tools should complement rather than replace
human graders, particularly for handling edge cases
and providing personalized feedback on creative so-
lutions.
Future work should explore how to combine the
strengths of different approaches, perhaps integrating
semantic similarity checks with LLM-based evalua-
tion to create more robust grading systems. Addition-
ally, investigating the applicability of this approach to
other types of UML diagrams and more open-ended
design tasks could further expand its utility in soft-
ware engineering education.
REFERENCES
Acu
˜
na, R., Baron, T., and Bansal, S. (2023). Autograder
impact on software design outcomes. In 2023 IEEE
Frontiers in Education Conference (FIE), pages 1–9.
Ahmed, F., Bouali, N., and Gerhold, M. (2024). Teach-
ing assistants as assessors: An experience based nar-
rative. In Poquet, O., Ortega-Arranz, A., Viberg, O.,
Chounta, I., McLaren, B. M., and Jovanovic, J., edi-
tors, Proceedings of the 16th International Conference
on Computer Supported Education, CSEDU 2024,
Angers, France, May 2-4, 2024, Volume 1, pages 115–
123. SCITEPRESS.
Albluwi, I. (2018). A closer look at the differences be-
tween graders in introductory computer science ex-
ams. IEEE Transactions on Education, 61(3):253–
260.
Ardimento, P., Bernardi, M. L., and Cimitile, M. (2024).
Teaching uml using a rag-based llm. In 2024 Interna-
tional Joint Conference on Neural Networks (IJCNN),
pages 1–8. IEEE.
Balse, R., Valaboju, B., Singhal, S., Warriem, J. M., and
Prasad, P. (2023). Investigating the potential of gpt-3
in providing feedback for programming assessments.
In Proceedings of the 2023 Conference on Innovation
and Technology in Computer Science Education V. 1,
ITiCSE 2023, page 292–298, New York, NY, USA.
Association for Computing Machinery.
Becker, B. A., Denny, P., Finnie-Ansley, J., Luxton-Reilly,
A., Prather, J., and Santos, E. A. (2023). Program-
ming is hard - or at least it used to be: Educational
opportunities and challenges of ai code generation. In
Proceedings of the 54th ACM Technical Symposium
on Computer Science Education V. 1, SIGCSE 2023,
page 500–506, New York, NY, USA. Association for
Computing Machinery.
Bergmans, L., Bouali, N., Luttikhuis, M., and Rensink, A.
(2021). On the efficacy of online proctoring using
proctorio. In Csap
´
o, B. and Uhomoibhi, J., editors,
Proceedings of the 13th International Conference on
Computer Supported Education, CSEDU 2021, On-
line Streaming, April 23-25, 2021, Volume 1, pages
279–290. SCITEPRESS.
Bian, W., Alam, O., and Kienzle, J. (2019). Automated
grading of class diagrams. In 2019 ACM/IEEE 22nd
International Conference on Model Driven Engineer-
ing Languages and Systems Companion (MODELS-
C), pages 700–709. IEEE.
Bian, W., Alam, O., and Kienzle, J. (2020). Is automated
grading of models effective? assessing automated
grading of class diagrams. In Proceedings of the
23rd ACM/IEEE International Conference on Model
Driven Engineering Languages and Systems, pages
365–376.
Boubekeur, Y., Mussbacher, G., and McIntosh, S. (2020).
Automatic assessment of students’ software models
using a simple heuristic and machine learning. In Pro-
ceedings of the 23rd ACM/IEEE International Con-
ference on Model Driven Engineering Languages and
Systems: Companion Proceedings, pages 1–10.
Caiza, J. C. (2013). Automatic grading : Review of tools
and implementations.
Foss, S., Urazova, T., and Lawrence, R. (2022a). Auto-
matic generation and marking of uml database design
diagrams. In Proceedings of the 53rd ACM Technical
Symposium on Computer Science Education-Volume
1, pages 626–632.
Foss, S., Urazova, T., and Lawrence, R. (2022b). Learn-
ing uml database design and modeling with autoer. In
Proceedings of the 25th International Conference on
Model Driven Engineering Languages and Systems:
Companion Proceedings, pages 42–45.
Matthews, K., Janicki, T., He, L., and Patterson, L. (2012).
Implementation of an automated grading system with
an adaptive learning component to affect student feed-
back and response time. Journal of Information Sys-
tems Education, 23(1):71–84.
Prather, J., Denny, P., Leinonen, J., Becker, B. A., Albluwi,
I., Craig, M., Keuning, H., Kiesler, N., Kohn, T.,
Luxton-Reilly, A., MacNeil, S., Petersen, A., Pettit,
CSEDU 2025 - 17th International Conference on Computer Supported Education
168