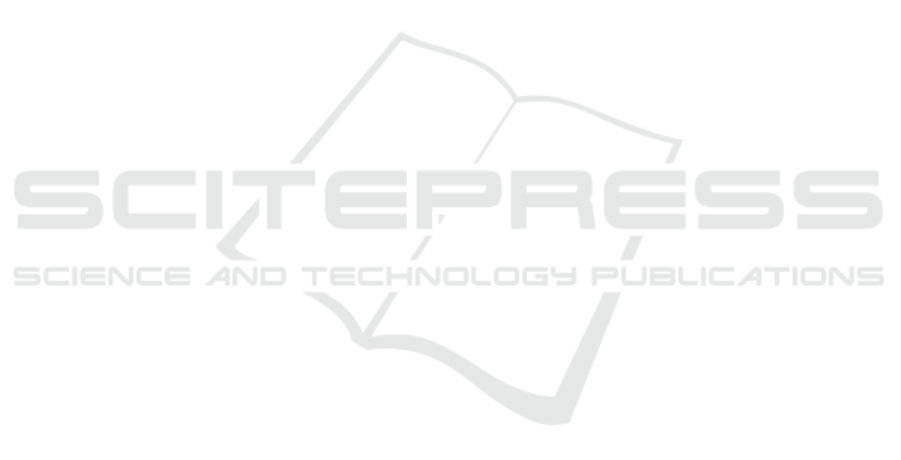
Bird, S., Klein, E., and Loper, E. (2009). Natural language
processing with Python: analyzing text with the natu-
ral language toolkit. ” O’Reilly Media, Inc.”.
Bucci, E. M. (2018). Automatic detection of image ma-
nipulations in the biomedical literature. Cell Death &
Disease, 9(400).
ClearSkies (n.d.). Papermill alarm. https://clear-skies.co.
uk/. Accessed: 2024-09-27.
Dadkhah, M., Oermann, M. H., Heged
¨
us, M., Raman, R.,
and D
´
avid, L. D. (2023). Detection of fake papers in
the era of artificial intelligence. Diagnosis, 10(4):390–
397.
Desaire, H., Chua, A. E., Kim, M.-G., and Hua, D. (2023).
Accurately detecting ai text when chatgpt is told to
write like a chemist. Cell Reports Physical Science,
4(11):101672.
Elsevier (2024). Scopus api.
Face, H. (2023). Transformers: State-of-the-art machine
learning for pytorch, tensorflow, and jax. https://
huggingface.co/transformers.
Gaudino, M., Robinson, N. B., Audisio, K., Rahouma,
M., Benedetto, U., Kurlansky, P., and Fremes, S. E.
(2021). Trends and Characteristics of Retracted Arti-
cles in the Biomedical Literature, 1971 to 2020. JAMA
Internal Medicine, 181(8):1118–1121.
Gpt, A. O. T. and Steingrimsson, S. (2022). Can gpt-3 write
an academic paper on itself, with minimal human in-
put? {hal-03701250}.
International Association of Scientific, Technical and Med-
ical Publishers (STM) (n.d.). Stm integrity hub. https:
//www.stm-assoc.org/stm-integrity-hub/. Accessed:
2024-09-27.
Kung, T. H., Cheatham, M., , Medenilla, A., Sillos, C.,
De Leon, L., Elepa
˜
no, C., Madriaga, M., Aggabao, R.,
Diaz-Candido, G., Maningo, J., and Tseng, V. (2022).
Performance of chatgpt on usmle: Potential for ai-
assisted medical education using large language mod-
els. medRxiv.
Liu, Q., Barhoumi, A., and Labb
´
e, C. (2024). Miscitations
in scientific papers: dataset and detection. Preprint.
Mikolov, T., Chen, K., Corrado, G., and Dean, J. (2013).
Efficient estimation of word representations in vector
space. In Proceedings of the International Conference
on Learning Representations (ICLR).
Noorden, R. V. (2023). More than 10,000 research pa-
pers were retracted in 2023 — a new record. Nature,
624:479–481.
Oksvold, M. P. (2016). Incidence of data duplications in
a randomly selected pool of life science publications.
Science and Engineering Ethics, 22(2):487–496.
O’Connor, S. and ChatGPT (2023). Open artificial intelli-
gence platforms in nursing education: Tools for aca-
demic progress or abuse? Nurse Education in Prac-
tice, 66:103537.
Pedregosa, F., Varoquaux, G., Gramfort, A., Michel, V.,
Thirion, B., Grisel, O., Blondel, M., Prettenhofer, P.,
Weiss, R., Dubourg, V., Vanderplas, J., Passos, A.,
Cournapeau, D., Brucher, M., Perrot, M., and
´
Edouard
Duchesnay (2011). Scikit-learn: Machine learning
in python. Journal of Machine Learning Research,
12(85):2825–2830.
Razis, G., Anagnostopoulos, K., Metaxas, O., Stefanidis,
S.-D., Zhou, H., and Anagnostopoulos, I. (2023). Pa-
permill detection in scientific content. pages 1–6.
Sabel, B. A., Knaack, E., Gigerenzer, G., and Bilc, M.
(2023). Fake publications in biomedical science: Red-
flagging method indicates mass production. medRxiv,
pages 2023–05.
Salton, G. and Buckley, C. (1988). Term-weighting ap-
proaches in automatic text retrieval. Information Pro-
cessing & Management, 24(5):513–523.
Shen, H. (2020). Meet this super-spotter of duplicated im-
ages in science papers. Nature, 581:132–136.
Stokel-Walker, C. (2023). Chatgpt listed as author on re-
search papers: many scientists disapprove. Nature,
613:620–621.
Theocharopoulos, P. C., Anagnostou, P., Tsoukala, A.,
Georgakopoulos, S. V., Tasoulis, S. K., and Pla-
gianakos, V. P. (2023). Detection of fake generated
scientific abstracts. In 2023 IEEE Ninth International
Conference on Big Data Computing Service and Ap-
plications (BigDataService), volume 33, page 33–39.
IEEE.
Transformer, C. and Zhavoronkov, A. (2022). Rapamycin in
the context of pascal’s wager: generative pre-trained
transformer perspective. Oncoscience, 9:82–84.
Williams, K. and Giles, C. L. (2015). On the use of similar-
ity search to detect fake scientific papers. In Amato,
G., Connor, R., Falchi, F., and Gennaro, C., editors,
Similarity Search and Applications, pages 332–338,
Cham. Springer International Publishing.
Xiong, J. and Huang, T. (2009). An effective method to
identify machine automatically generated paper. In
2009 Pacific-Asia Conference on Knowledge Engi-
neering and Software Engineering, pages 101–102.
ICEIS 2025 - 27th International Conference on Enterprise Information Systems
670