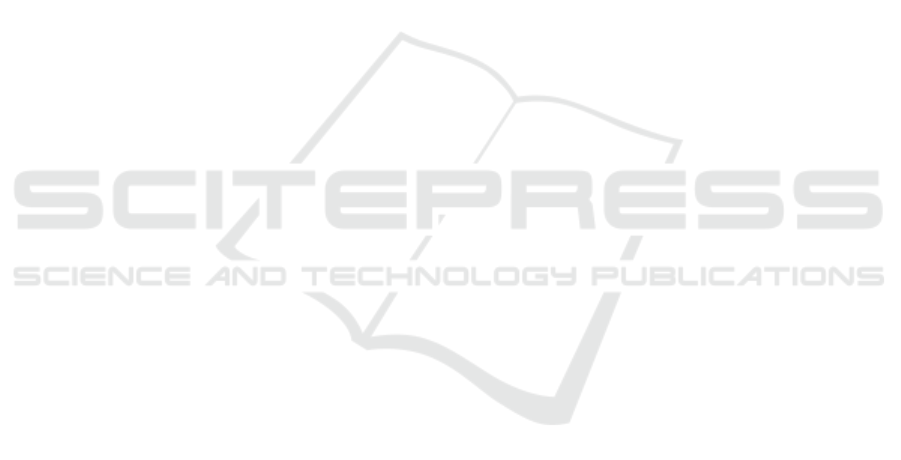
tation methods, especially at an augmentation ratio
of approximately 100%. Ablation studies further
highlight the importance of the architectural modi-
fications, establishing that self-attention and multi-
conditional conditioning both contribute to robust
performance improvements. These findings confirm
that advanced generative techniques can play a vital
role in supporting data-driven medical research and
decision-making, even when available data is limited.
REFERENCES
Al-Bwana, E. K., Sayahi, I., Alauthman, M., and Mahjoub,
M. A. (2024). Adverserial network augmentation
and tabular data for a new covid-19 diagnostics
approach. In 2024 10th International Conference
on Control, Decision and Information Technologies
(CoDIT), pages 2000–2005. IEEE.
Chawla, N. V., Bowyer, K. W., Hall, L. O., and Kegelmeyer,
W. P. (2002). Smote: synthetic minority over-
sampling technique. Journal of artificial intelligence
research, 16:321–357.
Creswell, A., White, T., Dumoulin, V., Arulkumaran, K.,
Sengupta, B., and Bharath, A. A. (2018). Generative
adversarial networks: An overview. IEEE signal pro-
cessing magazine, 35(1):53–65.
Dong, E., Du, H., and Gardner, L. (2020). An interactive
web-based dashboard to track covid-19 in real time.
The Lancet infectious diseases, 20(5):533–534.
Gigante, G., Guidotti, G. M., et al. (2021). Do chinese-
focused us listed spacs perform better than others
do? Investment management & financial innovations,
18(3):229–248.
Goodfellow, I., Pouget-Abadie, J., Mirza, M., Xu, B.,
Warde-Farley, D., Ozair, S., Courville, A., and Ben-
gio, Y. (2014). Generative adversarial nets. Advances
in neural information processing systems, 27.
Javadi-Moghaddam, S.-M., Gholamalinejad, H., and Fard,
H. M. (2023). Coviddcgan: Oversampling model us-
ing dcgan network to balance a covid-19 dataset. In-
ternational Journal of Information Technology & De-
cision Making, 22(05):1533–1549.
Jordon, A., Hawkins-Seagram, A., Norrie, S., Ossorio, J.,
and Stege, U. (2023). Qwalkvis: Quantum walks vi-
sualization application. In 2023 IEEE International
Conference on Quantum Computing and Engineering
(QCE), volume 03, pages 87–93.
Mozaffari, J., Amirkhani, A., and Shokouhi, S. B. (2023).
A survey on deep learning models for detection
of covid-19. Neural Computing and Applications,
35(23):16945–16973.
Mumuni, A. and Mumuni, F. (2024). Data augmentation
with automated machine learning: approaches and
performance comparison with classical data augmen-
tation methods. ArXiv, abs/2403.08352.
Nik, A. H. Z., Riegler, M. A., Halvorsen, P., and Stor
˚
as,
A. M. (2023). Generation of synthetic tabular health-
care data using generative adversarial networks. In
International Conference on Multimedia Modeling,
pages 434–446. Springer.
Rounaq, S., Shaikh, M., Siddiqui, D. R., et al. (2023). De-
tection of covid-19 cases using gan (generative adver-
sarial network). Ghulam and Siddiqui, Dr. Raheel,
Detection of Covid-19 Cases Using Gan (Generative
Adversarial Network).
Wu, Z. and McGoogan, J. M. (2020). Characteristics of and
important lessons from the coronavirus disease 2019
(covid-19) outbreak in china: summary of a report of
72 314 cases from the chinese center for disease con-
trol and prevention. jama, 323(13):1239–1242.
Xu, L., Skoularidou, M., Cuesta-Infante, A., and Veera-
machaneni, K. (2019). Modeling tabular data using
conditional gan. Advances in neural information pro-
cessing systems, 32.
TGAN and CTGAN: A Comparative Analysis for Augmenting COVID 19 Tabular Data
393