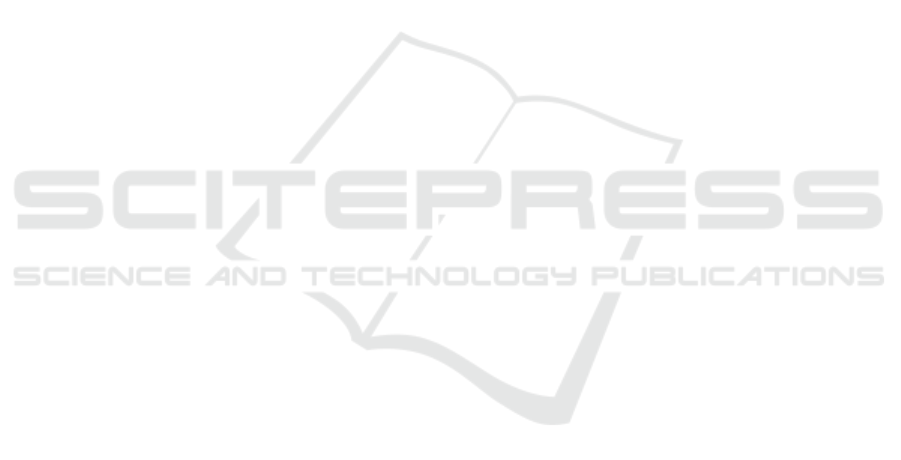
Lanier, J. and Biocca, F. (1992). An insider’s view of the
future of virtual reality. Journal of Communication,
42(4):150–172.
Leng, J., Sha, W., Wang, B., Zheng, P., Zhuang, C., Liu, Q.,
Wuest, T., Mourtzis, D., and Wang, L. (2022). Indus-
try 5.0: Prospect and retrospect. Journal of Manufac-
turing Systems, 65:279–295.
Nguyen, V. T. and Dang, T. (2017). Setting up virtual re-
ality and augmented reality learning environment in
unity. In Adjunct Proceedings of the 2017 IEEE Inter-
national Symposium on Mixed and Augmented Real-
ity, ISMAR-Adjunct 2017, pages 315–320. Institute of
Electrical and Electronics Engineers Inc.
Nouira, A., Cheniti-Belcadhi, L., and Braham, R. (2018).
An enhanced xapi data model supporting assessment
analytics. Procedia Computer Science, 126:566–575.
Knowledge-Based and Intelligent Information And
Engineering Systems: Proceedings of the 22nd Inter-
national Conference, KES-2018, Belgrade, Serbia.
Paszkiewicz, A., Salach, M., Dymora, P., Bolanowski, M.,
Budzik, G., and Kubiak, P. (2021). Methodology of
implementing virtual reality in education for industry
4.0. Sustainability, 13(9).
Pedram, S., Perez, P., Palmisano, S., and Farrelly, M.
(2017). Evaluating 360-virtual reality for mining in-
dustry’s safety training. In HCI International 2017–
Posters’ Extended Abstracts: 19th International Con-
ference, HCI International 2017, Vancouver, BC,
Canada, July 9–14, 2017, Proceedings, Part I 19, vol-
ume 713, pages 555–561. Springer Verlag.
Pooladvand, S., Chang, W.-C., and Hasanzadeh, S. (2024).
Identifying at-risk workers using fnirs-based mental
load classification: A mixed reality study. Automation
in Construction, 164:105453.
Porr, B. and Bohollo, L. M. (2022). Bci-walls:
A robust methodology to predict success or fail-
ure in brain computer interfaces. arXiv preprint
arXiv:2210.16939.
Pottle, J. (2019). Virtual reality and the transformation
of medical education. Future healthcare journal,
6(3):181.
Rashid, M., Sulaiman, N., P. P. Abdul Majeed, A., Musa,
R. M., Ab. Nasir, A. F., Bari, B. S., and Khatun, S.
(2020). Current status, challenges, and possible solu-
tions of eeg-based brain-computer interface: A com-
prehensive review. Frontiers in Neurorobotics, 14.
Schardosim Simao, J. P., Mellos Carlos, L., Saliah-Hassane,
H., Da Silva, J. B., and Da Mota Alves, J. B. (2018).
Model for recording learning experience data from re-
mote laboratories using xapi. In Proceedings - 13th
Latin American Conference on Learning Technolo-
gies, LACLO 2018, page 450 – 457. Cited by: 7.
Scorgie, D., Feng, Z., Paes, D., Parisi, F., Yiu, T. W., and
Lovreglio, R. (2024). Virtual reality for safety train-
ing: A systematic literature review and meta-analysis.
Slater, M., Banakou, D., Beacco, A., Gallego, J., Macia-
Varela, F., and Oliva, R. (2022). A separate reality:
An update on place illusion and plausibility in virtual
reality. Frontiers in Virtual Reality, 3.
Slater, M., Usoh, M., and Steed, A. (1994). Depth of pres-
ence in virtual environments. Presence: Teleoperators
& Virtual Environments, 3:130–144.
Stefan, H., Mortimer, M., and Horan, B. (2023). Eval-
uating the effectiveness of virtual reality for safety-
relevant training: a systematic review. Virtual Reality,
27(4):2839 – 2869. Cited by: 1; All Open Access,
Hybrid Gold Open Access.
Teodoro, P. N. B., Cardoso, A., and Ramos, D. C. (2023).
Combinac¸
˜
ao do framework 70-20-10 com kirkpatrick
model para construc¸
˜
ao e avaliac¸
˜
ao de treinamentos
apoiados por realidade virtual. Revista Cient
´
ıfica Uni-
versitas, 10(1):73–87.
Thorvald, P., Fast Berglund,
˚
A., and Romero, D. (2021).
The cognitive operator 4.0. In 18th International Con-
ference on Manufacturing Research, ICMR 2021, in-
corporating the 35th National Conference on Man-
ufacturing Research, 7–10 September 2021, Univer-
sity of Derby, Derby, UK, volume 15, pages 3–8. IOS
Press.
Toppi, J., Borghini, G., Petti, M., He, E. J., De Giusti, V.,
He, B., Astolfi, L., and Babiloni, F. (2016). Investi-
gating cooperative behavior in ecological settings: an
eeg hyperscanning study. PloS one, 11(4):e0154236.
Vidal, J. C., Rabelo, T., and Lama, M. (2015). Semantic de-
scription of the experience api specification. In Pro-
ceedings - IEEE 15th International Conference on Ad-
vanced Learning Technologies: Advanced Technolo-
gies for Supporting Open Access to Formal and Infor-
mal Learning, ICALT 2015, page 268 – 269. Cited by:
10.
Villani, V., Gabbi, M., and Sabattini, L. (2022). Promoting
operator’s wellbeing in industry 5.0: detecting men-
tal and physical fatigue. In 2022 IEEE International
Conference on Systems, Man, and Cybernetics (SMC),
pages 2030–2036.
Viol, R. S., Fernandes, P. C., Lacerda, I. I., Melo, K. G. F.,
Nunes, A. J. D. A., Pimenta, A. S. G. a. M., Carneiro,
C. M., Silva, M. C., and Delabrida, S. (2024). Eduvr:
Towards an evaluation platform for user interactions
in personalized virtual reality learning environments.
In Proceedings of the XXII Brazilian Symposium on
Human Factors in Computing Systems, IHC ’23, New
York, NY, USA. Association for Computing Machin-
ery.
Werbi
´
nska-Wojciechowska, S. and Winiarska, K. (2023).
Maintenance performance in the age of industry 4.0:
A bibliometric performance analysis and a systematic
literature review. Ler os artigos 146, 158, 176, 187,
188, 189, 190.
Yadav, D., Yadav, S., and Veer, K. (2020). A comprehen-
sive assessment of brain computer interfaces: Recent
trends and challenges. Journal of Neuroscience Meth-
ods.
Towards a VR-BCI Based System to Evaluate the Effectiveness of Immersive Technologies in Industry
701