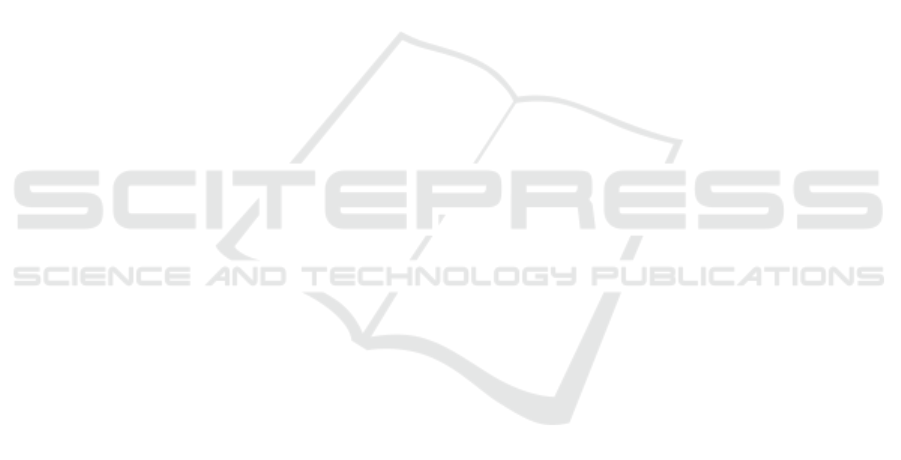
In our modeling, we use notation r to refer to a
resource in the set R of resources to be outsourced,
for which a certain number n ≥ 0 of replicas can exist,
without distinguishing whether reference is made to
the original version of the resource or to one of its
replicas. When explicit reference to a specific version
of a resource (i.e., its original version or one of its
replicas) is needed, we distinguish original resources
from replicas with superscripts: r
0
denotes the
original version of r, while r
i
denotes the i
th
replica
of r. We denote the original version and the set of
replicas existing for resource r with symbol R(r). For
example, with reference to the resources in Figure 3,
consider resource insurance, for which 2 replicas
are needed in addition to the original resource. When
writing insurance, we refer to a generic version
of the resource without explicit consideration of
whether we are referring to the original version or to
one replica. When writing insurance
0
we explicitly
refer to the original version. Also, R(insurance) =
{insurance
0
, insurance
1
, insurance
2
}, which
includes the original version (insurance
0
) and
the first (insurance
1
) and second (insurance
2
)
replica. For readability, we denote with R the
overall set of original resources and their replicas
R =
S
r∈R
R(r). For example, with reference to the
resources in Figure 3, R will include 14 resources:
the 7 original resources, as well as one replica for
each resource but insurance (for which 2 replicas
are defined), and equipment (for which no replica is
defined).
Allocation Modeling. Given a set R of resources and
a set V of nodes, our goal is to determine an alloca-
tion λ : R → V that maps each resource (original or
replica) to a node.
Given a set of nodes, it may be that not every
node is suitable to store every resource. This can be
due to two main factors. The first concerns the fit
between the peculiarities of each node vs. the spe-
cific characteristics or requirements of different re-
sources. For instance, certain resources may require
high-performance nodes with fast processing capabil-
ities, while others might need high-capacity nodes op-
timized for bulk storage. The second factor reflects
the interplay between resource allocations, where the
placement of one resource may influence the suitabil-
ity of a node for storing another resource (e.g., due to
capacity limitations, performance constraints, or op-
erational dependencies).
These two factors motivate two distinct families
of requirements, which may be specified by data own-
ers to regulate allocation. The first family of require-
ments addresses the suitability of individual nodes
for specific resources: the enforcement of such con-
straints determines, for each resource, a set of accept-
able nodes. The second family of requirements ad-
dresses the interplay among allocations: the enforce-
ment of such requirements over acceptable nodes
guarantees that the overall allocation fits the desider-
ata of the owner. We illustrate the first family of re-
quirements along with the notion of acceptable node
in Section 3. We then illustrate the second family of
requirements in Section 4.
3 ACCEPTABLE NODES
The first kind of requirements that should be consid-
ered when computing an allocation of data to stor-
age nodes considers the fit between the characteris-
tics of the different nodes, and those of the resources
to be allocated. This kind of requirements is spec-
ified for each resource r independently, and defines
the node characteristics that make a node suitable or
unsuitable for r. For example, a resource requiring
high reliability might be restricted to nodes with ro-
bust failover mechanisms, while a resource involv-
ing latency-sensitive operations might be allocated to
nodes with low communication delay. By capturing
these requirements, data owners can ensure that their
resources can be allocated only to nodes that meet
their needs and expectations. For clarity, in the re-
mainder of this paper we will denote such require-
ments as resource-level requirements. There are sev-
eral approaches that can be used to specify, and en-
force, this kind of requirements (e.g., (De Capitani
di Vimercati et al., 2021b; De Capitani di Vimer-
cati et al., 2021a)). We aim at remaining general
and do not restrict our approach to operate with any
specific approach. For example, the proposal in (De
Capitani di Vimercati et al., 2021b) provides a lan-
guage for specifying complex resource-level require-
ments, building upon the concept of base require-
ment, denoted c. Given a set {w
1
, . . . , w
n
} of values
in the domain of a node attribute (characteristic) at,
a base requirement on at imposes that at can assume
(at(w
1
, . . . , w
n
)) or cannot assume (¬at(w
1
, . . . , w
n
))
such a set of values. For instance, a base require-
ment of the form prov(provA, provB, provC) states
that, to be acceptable, a node must be managed by
provider provA, provB, or provC. Starting from base
requirements, the specification language in (De Cap-
itani di Vimercati et al., 2021b) permits to express a
variety of complex requirements, summarized in Fig-
ure 4(a). Those complex requirements can model al-
ternatives among base requirements (ANY), sets of
base requirements that must be jointly satisfied (ALL),
conditional requirements (IF-THEN), prohibited char-
Security-Aware Allocation of Replicated Data in Distributed Storage Systems
83