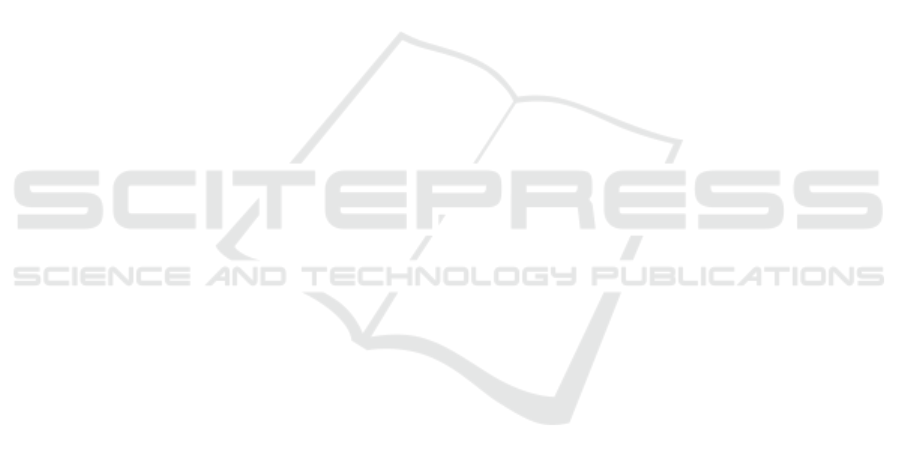
to address unreliable V2V scenarios (Li et al., 2022).
The proposed solution focuses on decentralized data
consistency over long distances, leveraging LoRa’s
capabilities. While effective in scenarios like pla-
tooning, the system faces limitations in transmis-
sion speed and latency, suggesting a need for further
research into advanced communication technologies
like Cellular-V2X (C-V2X).
In a more generic proposal (Vieira et al., 2024a),
that is able to support different consensus protocols,
Vieira et al. introduce the Verifiable Event Exten-
sion (VEE), a lightweight and modular applicational
extension designed specifically for Intelligent Trans-
portation System (ITS) messages. It enhances vehic-
ular networks by seamlessly adding functionality for
data security, consensus, and trading while maintain-
ing compatibility with existing V2X communication
standard protocols. This design ensures that the im-
plementation does not disrupt or require significant
modifications to the current Cooperative ITS (C-ITS)
framework.
VEE is structured into three core modules that
provide specific functionalities. The Ledger Mod-
ule utilizes localchains, a geographically based dis-
tributed ledger technology, to ensure data immutabil-
ity and traceability, making vehicular data tamper-
proof and highly reliable. The Consensus Module
handles consensus processes, providing an agreement
mechanism for various applications. The PBFT algo-
rithm is given as an example, to enable fault-tolerant
consensus among network participants, particularly
for non safety-critical scenarios. Lastly, the Token
Module facilitates cryptocurrency-based transactions,
enabling the secure and efficient exchange of digital
assets or services, such as toll payments or coopera-
tive maneuver rewards, within the vehicular network.
The VEE framework is designed with perfor-
mance efficiency in mind, minimizing overhead on
the communication channel by leveraging existing
ITS messages as a transport medium. This ap-
proach avoids the need for additional message head-
ers, reducing the network load. The feasibility and
lightweight nature of the system have been validated
through HiL setups, demonstrating its capability to
function effectively under real-world vehicular con-
straints. VEE significantly enhances the security and
accountability of cooperative maneuvers by recording
and verifying vehicular event data. It also supports
distributed trading mechanisms, such as instant road
toll payments and reward systems for cooperative ma-
neuvers, providing a robust platform for value-added
vehicular services. The modular design allows for
customization, enabling its deployment in various ve-
hicular scenarios while addressing diverse use cases.
Finally, V2X messages may lack reliability, for
instance due to sensor errors or malicious nodes,
even when essential security mechanisms (e.g., stan-
dard authentication and authorization protocols) are
in place (Vieira et al., 2024b). To address this issue,
the authors propose the inclusion of consensus infor-
mation on top of the standard V2X messages, in or-
der to be validated using the PBFT algorithm. This
way, it is possible to achieve agreement among vehi-
cles, even in the presence of faults or malicious ac-
tors. The methodology was tested using a HiL setup
that simulates real-world conditions, by introducing a
configurable packet loss rate in the communications
between four OBUs running the ETSI ITS-G5 proto-
col stack. The obtained results showed that dedicated
messages were faster for consensus, while extended
messages were better suited to dense traffic scenarios
due to their lower wireless medium impact. Channel
Busy Ratio (CBR) measurements confirmed the feasi-
bility of both methods. The extended V2X messages
approach is suitable for non-safety-critical scenarios
but may require optimization for time-sensitive tasks.
The reviewed works collectively highlight signif-
icant progress in developing consensus mechanisms
tailored for vehicular networks. From enhancing
PBFT with veto-gathering phases and gossip algo-
rithms to exploring alternative approaches such as
Raft and integrating blockchain technologies, these
efforts address critical challenges such as fault toler-
ance, scalability, and reliability under diverse condi-
tions. However, certain gaps remain unaddressed.
Existing methods typically rely on majority-based
consensus, which, while efficient, can fall short
in scenarios that require unanimous agreement for
safety-critical applications. Although the one-vote
veto mechanism addresses this to some extent, its re-
liance on unanimity can introduce additional delays
and inefficiencies, particularly under moderate/high
packet loss conditions or when the network encom-
passes untrusted nodes. Furthermore, alternative al-
gorithms such as 3PC and other BFT protocols have
not been extensively explored in the context of vehic-
ular networks, leaving questions about their compar-
ative performance under varying packet loss rates and
operational constraints.
To address these limitations, this work focuses on
the systematic evaluation and comparison of differ-
ent consensus algorithms, including PBFT, 3PC, and
other BFT protocols, under diverse network condi-
tions and packet loss rates.
Application of Consensus Protocols to Vehicular Communications Scenarios for the Negotiation of Cooperative Traffic Maneuvers
237