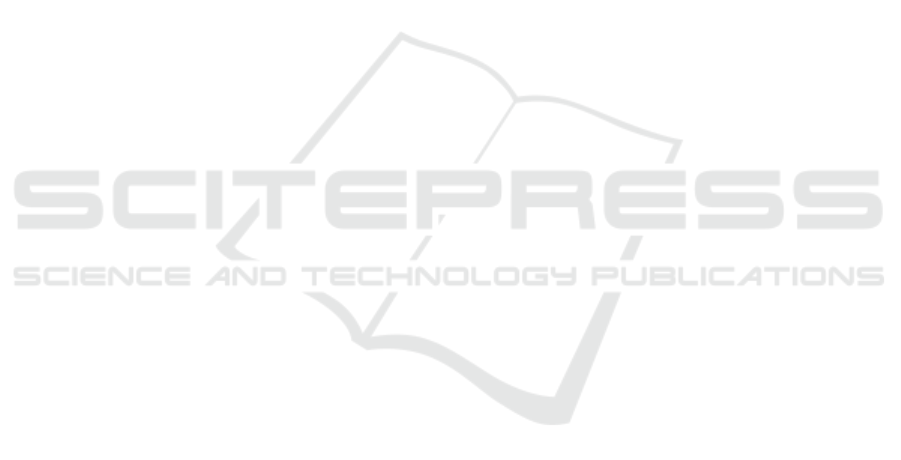
overhead is notable in memory-intensive tasks, its
minimal impact on compute-intensive benchmarks in-
dicates mdx II capability to effectively support diverse
HPC and HPDA applications.
Future efforts will focus on analyzing and opti-
mizing the performance of various large-scale real-
world data science workloads on mdx II, solidifying
the role of mdx II in advancing data science research
and facilitating cross-disciplinary collaborations. An-
other direction is to explore the energy efficiency and
cost-effectiveness of mdx II in comparison to other
academic and public clouds.
ACKNOWLEDGMENTS
This work was partially supported by JST ACT-X
Grant Number JPMJAX24M6, as well as JSPS KAK-
ENHI Grant Numbers JP20K19808 and JP23K16890.
The mdx II system was used to carry out experiments.
REFERENCES
Bugnion, E., Nieh, J., and Tsafrir, D. (2017). Hardware
and Software Support for Virtualization, volume 12.
Morgan & Claypool.
Castro, O., Bruneau, P., Sottet, J. S., and Torregrossa, D.
(2023). Landscape of High-Performance Python to
Develop Data Science and Machine Learning Appli-
cations. ACM Computing Surveys, 56(3).
Date, S., Kido, Y., Katsuura, Y., Teramae, Y., and Kigoshi,
S. (2023). Supercomputer for Quest to Unsolved
Interdisciplinary Datascience (SQUID) and its Five
Challenges. In Sustained Simulation Performance
2021, pages 1–19.
Dreseler, M., Boissier, M., Rabl, T., and Uflacker, M.
(2020). Quantifying TPC-H choke points and their
optimizations. In Proceedings of the VLDB Endow-
ment, volume 13, pages 1206–1220.
Fukazawa, K. and Takahashi, R. (2024). Performance Eval-
uation of the Fourth-Generation Xeon with Different
Memory Characteristics. ACM International Confer-
ence Proceeding Series, (May):55–62.
Gross, J., Ganga, I., and Sridhar, T. (2020). Geneve:
Generic Network Virtualization Encapsulation. Tech-
nical report.
Hancock, D. Y., Fischer, J., Lowe, J. M., Snapp-Childs,
W., Pierce, M., Marru, S., Coulter, J. E., Vaughn, M.,
Beck, B., Merchant, N., Skidmore, E., and Jacobs,
G. (2021). Jetstream2: Accelerating cloud comput-
ing via Jetstream. In Practice and Experience in Ad-
vanced Research Computing, number Ci, pages 1–8,
New York, NY, USA. ACM.
Li, J., Bobyr, A., Boehm, S., Brantley, W., Brunst, H., Cave-
lan, A., Chandrasekaran, S., Cheng, J., Ciorba, F. M.,
Colgrove, M., Curtis, T., Daley, C., Ferrato, M., De
Souza, M. G., Hagerty, N., Henschel, R., Juckeland,
G., Kelling, J., Li, K., Lieberman, R., McMahon, K.,
Melnichenko, E., Neggaz, M. A., Ono, H., Ponder,
C., Raddatz, D., Schueller, S., Searles, R., Vasilev, F.,
Vergara, V. M., Wang, B., Wesarg, B., Wienke, S., and
Zavala, M. (2022). SPEChpc 2021 Benchmark Suites
for Modern HPC Systems. In ICPE 2022 - Compan-
ion of the 2022 ACM/SPEC International Conference
on Performance Engineering, pages 15–16.
Lockwood, G. K., Tatineni, M., and Wagner, R. (2014). SR-
IOV: Performance Benefits for Virtualized Intercon-
nects. In Proceedings of the 2014 Annual Conference
on Extreme Science and Engineering Discovery Envi-
ronment, pages 1–7, New York, NY, USA. ACM.
Philip Chen, C. L. and Zhang, C. Y. (2014). Data-intensive
applications, challenges, techniques and technolo-
gies: A survey on Big Data. Information Sciences,
275:314–347.
Raschka, S., Patterson, J., and Nolet, C. (2020). Machine
learning in python: Main developments and technol-
ogy trends in data science, machine learning, and arti-
ficial intelligence. Information (Switzerland), 11(4).
Russell, R. (2008). Virtio: Towards a de-facto standard for
virtual I/O devices. Operating Systems Review (ACM),
42(5):95–103.
Stewart, C. A., Fischer, J., Merchant, N., Stanzione, D. C.,
Hancock, D. Y., Cockerill, T., Miller, T., Taylor, J.,
Vaughn, M., Liming, L., Lowe, J. M., and Skidmore,
E. (2016). Jetstream - Performance, early experiences,
and early results. ACM International Conference Pro-
ceeding Series, 17-21-July-2016.
Stewart, C. A., Hancock, D., Stanzioneb, D., Turnerd, G.,
Cockerill, T. M., Merchant, N., Taylor, J., Vaughn, M.,
Foster, I., Skidmore, E., Tuecke, S., and Gaffney, N. I.
(2015). Jetstream: A self-provisioned, scalable sci-
ence and engineering cloud environment. ACM Inter-
national Conference Proceeding Series, 2015-July.
Suzumura, T., Sugiki, A., Takizawa, H., Imakura, A., Naka-
mura, H., Taura, K., Kudoh, T., Hanawa, T., Sekiya,
Y., Kobayashi, H., Kuga, Y., Nakamura, R., Jiang, R.,
Kawase, J., Hanai, M., Miyazaki, H., Ishizaki, T., Shi-
motoku, D., Miyamoto, D., Aida, K., Takefusa, A.,
Kurimoto, T., Sasayama, K., Kitagawa, N., Fujiwara,
I., Tanimura, Y., Aoki, T., Endo, T., Ohshima, S.,
Fukazawa, K., Date, S., and Uchibayashi, T. (2022).
mdx: A Cloud Platform for Supporting Data Science
and Cross-Disciplinary Research Collaborations. In
Proceedings of the 2022 IEEE International Confer-
ence on Dependable, Autonomic and Secure Com-
puting, International Conference on Pervasive Intel-
ligence and Computing, International Conference on
Cloud and Big Data Computing, International Con-
ference on Cy, pages 1–7. IEEE.
Towns, J., Cockerill, T., Dahan, M., Foster, I., Gaither, K.,
Grimshaw, A., Hazlewood, V., Lathrop, S., Lifka, D.,
Peterson, G. D., Roskies, R., Scott, J. R., and Wilkens-
Diehr, N. (2014). XSEDE: Accelerating scientific
discovery. Computing in Science and Engineering,
16(5):62–74.
CLOSER 2025 - 15th International Conference on Cloud Computing and Services Science
100