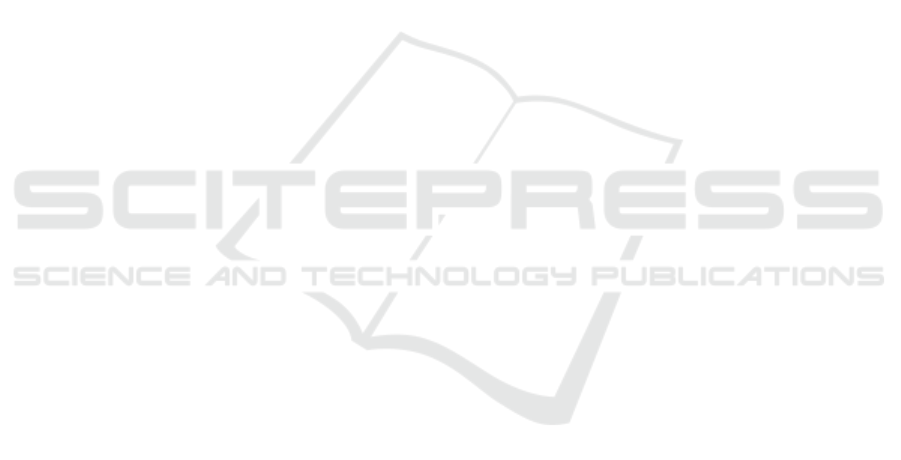
track a student’s progress over a large period of time,
or could be used as a tool for studying the effective-
ness of various practice strategies. The benefits in
transforming our approach from a single practice ses-
sion to a large corpus of practice data could make a
lasting contribution to music pedagogy.
REFERENCES
Coutts, L. (2019). Empowering students to take ownership
of their learning: Lessons from one piano teacher’s
experiences with transformative pedagogy. Interna-
tional Journal of Music Education, 37(3):493–507.
Cuvillier, P. (2016). On temporal coherency of probabilis-
tic modesl for audio-to-score alignment. PhD thesis,
Universit
´
e Pierre-et-Marie-Curie.
Dannenberg, R. and Raphael, C. (2006). Music score align-
ment and computer accompaniment. Commun. ACM,
49:38–43.
Dannenberg, R. B. (1984). An on-line algorithm for real-
time accompaniment. In Proceedings of the 1984 In-
ternational Computer Music Conference, ICMC 1984,
Paris, France, October 19-23, 1984. Michigan Pub-
lishing.
Dannenberg, R. B., Sanchez, M., Joseph, A., Capell, P.,
Joseph, R., and Saul, R. (1990). An expert sys-
tem for teaching piano to novices. In Proceedings of
the 1990 International Computer Music Conference,
ICMC 1990, Glasgow, Scotland, September 10-15,
1990. Michigan Publishing.
Dorfer, M., jr., J. H., Arzt, A., Frostel, H., and Widmer,
G. (2018). Learning audio–sheet music correspon-
dences for cross-modal retrieval and piece identifica-
tion. Transactions of the International Society for Mu-
sic Information Retrieval, 1(1):22.
Fober, D., Letz, S., Orlarey, Y., Askenfelt, A., Falkenberg-
Hansen, K., and Schoonderwaldt, E. (2004). IMUTUS
- an Interactive Music Tuition System. In IRCAM, ed-
itor, Sound and Music Computing Conference, pages
97–103, Paris, France.
Fremery, C., M
¨
uller, M., and Clausen, M. (2010). Handling
repeats and jumps in score performance synchroniza-
tion. In Proc. of the International Conference on Mu-
sic Information Retrieval (ISMIR).
Hogr
¨
afer, M., Heitzler, M., and Schulz, H.-J. (2020). The
state of the art in map-like visualization. In Computer
Graphics Forum, volume 39, pages 647–674. Wiley
Online Library.
Hu, N., Dannenberg, R. B., and Tzanetakis, G. (2003).
Polyphonic audio matching and alignment for music
retrieval. In in Proc. IEEE WASPAA, pages 185–188.
Jiang, Y., Ryan, F., Cartledge, D., and Raphael, C. (2019).
Offline score alignment for realistic music practice. In
Proc. of the Sound Music and Computing Conference
(SMC).
M
¨
uller, M., Kurth, F., and Clausen, M. (2005). Audio
matching via chroma-based statistical features. pages
288–295.
Nakamura, T., Nakamura, E., and Sagayama, S. (2015).
Real-time audio-to-score alignment of music perfor-
mances containing errors and arbitrary repeats and
skips. CoRR, abs/1512.07748.
Pardo, B. and Birmingham, W. P. (2005). Modeling form
for on-line following of musical performances. In
AAAI.
Rabiner, L. R. (1989). A tutorial on hidden Markov models
and selected applications in speech recognition. Pro-
ceedings of the IEEE, 77(2):257–286.
Raphael, C. (2006). Aligning music audio with symbolic
scores using a hybrid graphical model. Machine
Learning, 65:389–409.
Silveira J. M., . G. R. (2016). The effect of audio record-
ing and playback on self-assessment among middle
school instrumental music students. Psychology of
Music, 44(4):880–892.
Vercoe, B. (1984). The synthetic performer in the context
of live performance. In Proceedings of the 1984 In-
ternational Computer Music Conference, ICMC 1984,
Paris, France, October 19-23, 1984. Michigan Pub-
lishing.
Yousician (2022). Yousician. http://yousician.com.
Zhang, Y., Li, Y., Chin, D., and Xia, G. (2019). Adap-
tive multimodal music learning via interactive-haptic
instrument. arXiv preprint arXiv:1906.01197.
Face the Music: Summarizing Unscripted Music Practice from Audio
691