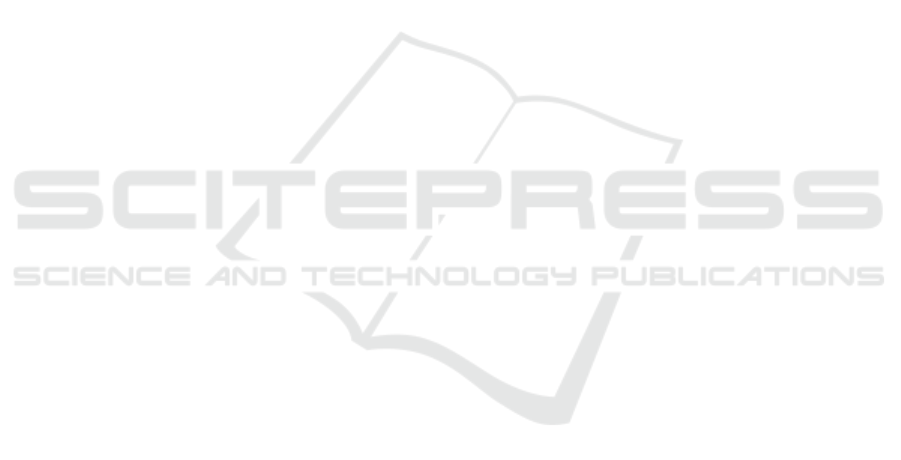
CKAN (2025). Ckan - open source data portal. Accessed:
2025-02-21.
Ferr
´
e-Bigorra, J., Casals, M., and Gangolells, M. (2022).
The adoption of urban digital twins. Cities.
FIWARE Foundation (2024). Fiware: Open source platform
for smart applications. Accessed: 2024-11-27.
for BOLD Cities, C. (2025). Urban digital twins. Accessed:
2025-02-21.
Hardi, R., Nurmandi, A., Purwaningsih, T., and Ab-
dul Manaf, H. B. (2023). Exploration organiza-
tional interoperability in smart governance in indone-
sia and malaysia. In Stephanidis, C., Antona, M.,
Ntoa, S., and Salvendy, G., editors, HCI International
2023 Posters, pages 203–210, Cham. Springer Nature
Switzerland.
Hatzivasilis, G., Askoxylakis, I. G., Alexandris, G., Ani-
cic, D., Br
¨
oring, A., Kulkarni, V., Fysarakis, K.,
and Spanoudakis, G. (2018). The interoperability of
things: Interoperable solutions as an enabler for iot
and web 3.0. 2018 IEEE 23rd International Workshop
on Computer Aided Modeling and Design of Commu-
nication Links and Networks (CAMAD), pages 1–7.
Karabulut, E., Pileggi, S. F., Groth, P., and Degeler, V.
(2024). Ontologies in digital twins: A systematic lit-
erature review. Future Generation Computer Systems,
153:442–456.
Kumar, S., Jeong, S., Ahn, I. Y., and Jarwar, M. A.
(2022). Things data interoperability through annotat-
ing onem2m resources for ngsi-ld entities. In 2022
IEEE International Conferences on Internet of Things
(iThings) and IEEE Green Computing & Communica-
tions (GreenCom) and IEEE Cyber, Physical & Social
Computing (CPSCom) and IEEE Smart Data (Smart-
Data) and IEEE Congress on Cybermatics (Cyber-
matics), pages 119–124.
Lu, Q., Parlikad, A. K., Woodall, P., Ranasinghe, G. D.,
Xie, X., Liang, Z., Konstantinou, E., Heaton, J., and
Schooling, J. (2020). Developing a digital twin at
building and city levels: Case study of west cam-
bridge campus. Journal of Management in Engineer-
ing, 36(3):05020004.
Maxis (2012). Inside the Glassbox (GDC 2012). Accessed:
2025-01-24.
Mihai, S., Yaqoob, M., Hung, D. V., Davis, W., Towakel,
P., Raza, M., Karamanoglu, M., Barn, B., Shetve, D.,
Prasad, R. V., Venkataraman, H., Trestian, R., and
Nguyen, H. X. (2022). Digital twins: A survey on
enabling technologies, challenges, trends and future
prospects. IEEE Communications Surveys & Tutori-
als, 24(4):2255–2291.
Mukherjee, T., Chander, D., Mondal, A., Dasgupta, K.,
Kumar, A., and Venkat, A. (2014). Cityzen: A
cost-effective city management system with incentive-
driven resident engagement. In 2014 IEEE 15th In-
ternational Conference on Mobile Data Management,
volume 1, pages 289–296.
Peldon, D., Banihashemi, S., LeNguyen, K., and Derrible,
S. (2024). Navigating urban complexity: The transfor-
mative role of digital twins in smart city development.
Sustainable Cities and Society, 111:105583.
Pliatsios, A., Kotis, K., and Goumopoulos, C. (2023).
A systematic review on semantic interoperability in
the ioe-enabled smart cities. Internet of Things,
22:100754.
Quek, H. Y., Sielker, F., Kraft, M., Akroyd, J., Bhave, A.,
Richthofen, A. V., Herthogs, P., Yamu, C., Wan, L.,
Nochta, T., Burgess, G., Lim, M. Q., Mosbach, S.,
and Balijepalli, V. S. K. M. (2021). The conundrum in
smart city governance: Interoperability and compati-
bility in an ever-growing digital ecosystem.
Rahman, H. and Hussain, M. I. (2020). A comprehen-
sive survey on semantic interoperability for internet
of things: State-of-the-art and research challenges.
Transactions on Emerging Telecommunications Tech-
nologies, 31(12):e3902.
Shih, C.-S. D., Yang, C.-M., and Cheng, Y.-C. (2015). Data
alignment for multiple temporal data streams without
synchronized clocks on iot fusion gateway. 2015 IEEE
International Conference on Data Science and Data
Intensive Systems, pages 667–674.
Tardieu, H. (2022). Role of gaia-x in the european data
space ecosystem. In Designing Data Spaces.
Thelen, A., Zhang, X., Fink, O., Lu, Y., Ghosh, S., Youn,
B. D., Todd, M. D., Mahadevan, S., Hu, C., and
Hu, Z. (2022). A comprehensive review of digital
twin — part 1: modeling and twinning
enabling technologies. Struct. Multidiscip. Optim.,
65(12).
Veltman, K. H. (2001). Syntactic and semantic interop-
erability: new approaches to knowledge and the se-
mantic web. New Review of Information Networking,
7(1):159–183.
Viola, F., Antoniazzi, F., Aguzzi, C., Kamienski, C., and
Roffia, L. (2019). Mapping the ngsi-ld context model
on top of a sparql event processing architecture: Im-
plementation guidelines. In 2019 24th Conference of
Open Innovations Association (FRUCT), pages 493–
501.
Waddell, P., Garcia-Dorado, I., Maurer, S. M., Boeing, G.,
Gardner, M., Porter, E., and Aliaga, D. G. (2018). Ar-
chitecture for modular microsimulation of real estate
markets and transportation. ArXiv, abs/1807.01148.
SMEN 2025 - Special Session on Smart City and Smart Energy Networks
204