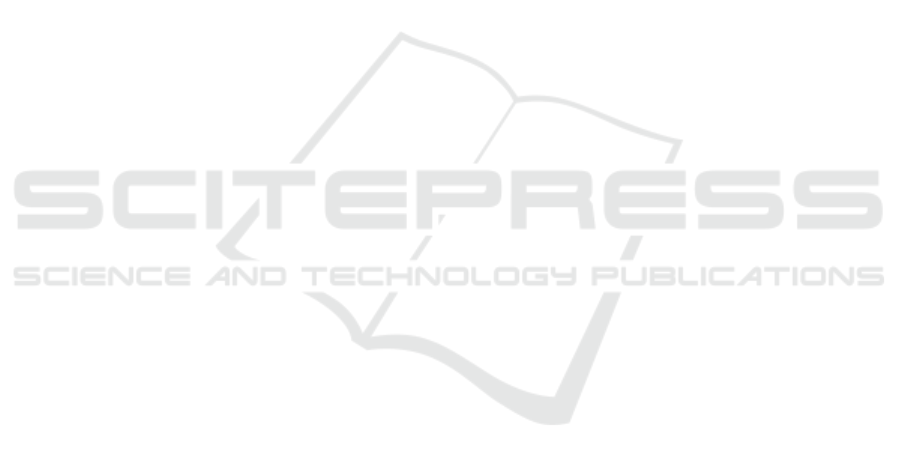
more complex Tasks, INTED2021 Proceedings,
pp. 3235-3241.
Brade, M. (2015). Visualization methods for the inter-
active acquisition and structuring of information
in the context of free-form knowledge model-
ing [Visualisierungsmethoden f
¨
ur das interaktive
Erfassen und Strukturieren von Informationen
im Kontext der Freiform-Wissensmodellierung].
Doctoral Dissertation, TUD Dresden University
of Technology.
Brooke, J. B. (1996). SUS: A ’Quick and Dirty’ Us-
ability Scale.
Christ, P., Laue, R., & Munkelt, T. (2022). ALADIN
– Generator for Tasks and Solution (Hints) in
Computer Science and Related Fields [ALADIN
– Generator f
¨
ur Aufgaben und L
¨
osung(shilf)en
aus der Informatik und angrenzenden Diszi-
plinen].
Christ, P. L., Munkelt, T., & Haake, J. M.
(2024). An Authoring Tool for the Graph-
ical Configuration of Item Generators
[Ein Autorenwerkzeug zur grafischen
Konfiguration von Aufgabengeneratoren].
https://doi.org/10.13140/RG.2.2.33616.11528
Damnik, G., Gierl, M., Proske, A., K
¨
orndle, H., &
Narciss, S. (2018). Automatic Item Generation
as a Means to Increase Interactivity and Adap-
tivity in Digital Learning Resources [Automa-
tische Erzeugung von Aufgaben als Mittel zur
Erh
¨
ohung von Interaktivit
¨
at und Adaptivit
¨
at in
digitalen Lernressourcen]. In E-Learning Sym-
posium 2018 (pp. 5-16). Universit
¨
atsverlag Pots-
dam.
Embretson, S. E., & Yang, X. (2007). Automatic item
generation and cognitive psychology. In C. R.
Rao & S. Sinharay (Eds.), Handbook of statis-
tics: Psychometrics, Volume 26 (pp. 747–768).
Amsterdam, The Netherlands: Elsevier.
Gierl, M. J., Lai, H., & Turner, S. (2012). Using
automatic item generation to create multiple-
choice items for assessments in medical educa-
tion. Medical Education,46, 757–765.
Gierl, M. J., & Haladyna, T. M. (Eds.). (2013). Au-
tomatic item generation: Theory and practice.
Routledge.
Gierl, M. J., & Lai, H. (2013a). Evaluating the qual-
ity of medical multiple-choice items created with
automated processes. Medical education, 47(7),
726-733.
Gierl, M. J., & Lai, H. (2016). Automatic item gener-
ation. In S. Lane, M. R. Raymond, & T.M. Hala-
dyna (Eds.), Handbook of test development (2nd
ed., pp. 410–429). New York, NY: Routledge.
Kosh, A. E., Simpson, M. A., Bickel, L., Kellogg,
M., & Sanford-Moore, E. (2019). A cost–benefit
analysis of automatic item generation. Educa-
tional Measurement: Issues and Practice, 38(1),
48-53.
Krathwohl, D. R. (2002). A Revision Bloom’s Taxon-
omy: An Overview. Theory into Practice, 41(4),
212-218.
Kucharski, S., Damnik, G., Stahr, F., & Braun, I.
(2023). Revision of the AIG Software Toolkit:
A Contribute to More User Friendliness and
Algorithmic Efficiency. In J. Jovanovic, I.-A.
Chounta, J. Uhomoibhi, & B. McLaren: Pro-
ceedings of the 15th International Conference
on Computer Supported Education - Volume 2:
CSEDU. SciTePress, pages 410-417.
Kucharski, S., Stahr, F., Braun, I. & Damnik, G.
(2024). Overcoming Student Passivity with Au-
tomatic Item Generation. In O. Poquet, A.
Ortega-Arranz, O. Viberg, I.-A. Chounta, B.
McLaren and J. Jovanovic: Proceedings of the
16th International Conference on Computer Sup-
ported Education. SciTePress, pages 789 - 798.
Merker, J., Hain, H., Sch
¨
obel, K., & Brassel,
P. (2023). 6. E-Assessment in STEM Fields:
Coding Exercises with Python & Jupyter [E-
Assessment in MINT-F
¨
achern: Coden von
¨
Ubungsaufgaben mit Python & Jupyter]. Dig-
itale Lehre im Rahmen der Grundlagenausbil-
dung in MINT-F
¨
achern an Hochschulen, 96.
Mortimer, T., Stroulia, E., & Yazdchi, M. V. (2012).
IGOR: A Web-Based Automatic Item Genera-
tion Tool. In Automatic Item Generation (pp.
217-230). Routledge.
Proske, A., K
¨
orndle, H. & Narciss, S. (2012). Inter-
active learning tasks. In N. M. Seel (Ed.), Ency-
clopedia of the Sciences of Learning (pp. 1606-
1610). New York: Springer.
Sauro, J. (2011). Measuring usability with the Sys-
tem Usability Scale (SUS). Retrieved from
https://measuringu.com/sus/. Last accessed: Jan-
uary 13, 2025.
Wancham, K., Tangdhanakanond, K., & Kan-
janawasee, S. (2023). Development of the auto-
matic item generation system for the diagnosis
of misconceptions about force and laws of mo-
tion. Eurasia Journal of Mathematics, Science
and Technology Education, 19(6), em2282.
AIG 2025 - Special Session on Automatic Item Generation
776