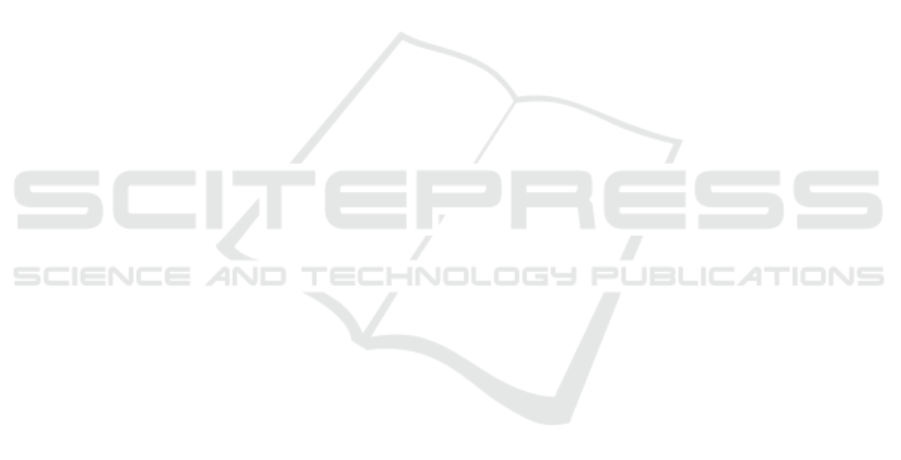
page 13–19, New York, NY, USA. Association for
Computing Machinery.
Atchariyachanvanich, K., Nalintippayawong, S., and Jula-
vanich, T. (2019). Reverse SQL Question Generation
Algorithm in the DBLearn Adaptive E-Learning Sys-
tem. IEEE Access, 7:54993–55004.
Becker, M., Liang, S., and Frank, A. (2021). Reconstruct-
ing Implicit Knowledge with Language Models. In
Workshop on Knowledge Extraction and Integration
for Deep Learning Architectures; Deep Learning In-
side Out.
Cc2020 Task Force (2020). Computing Curricula 2020:
Paradigms for Global Computing Education. ACM,
New York, NY, USA.
Chaudhari, M. S., Hire, M. A., Mandale, M. B., and Vanjari,
M. S. (2021). Structural Query Language Question
Creation by using Inverse Way.
Do, Q. C. D., Agrawal, R., Rao, D., and Gudivada, V. N.
(2014). Automatic Generation of SQL Queries.
Eleftherakis, S., Gkini, O., and Koutrika, G. (2021). Let the
Database Talk Back: Natural Language Explanations
for SQL. In SEA-DataVLDB.
Elmasri, R. and Navathe, S. (2016). Fundamentals of
database systems. Pearson, Hoboken, NJ, seventh edi-
tion edition.
Ericsson, K. A. (2006). The Influence of Experience and
Deliberate Practice on the Development of Superior
Expert Performance. In The Cambridge Handbook of
Expertise and Expert Performance, Cambridge Hand-
books in Psychology, pages 683–704. Cambridge Uni-
versity Press, Cambridge.
Farahnak, F., Rafiee, L., Kosseim, L., and Fevens, T. (2020).
Surface realization using pretrained language mod-
els. In IEEE Working Conference on Mining Software
Repositories.
Flesch, R. F. (1948). A new readability yardstick. Journal
of Applied Psychology, 32(3):221–233.
Gudivada, V. N., Arbabifard, K., and Rao, D. (2017). Auto-
mated Generation of SQL Queries that Feature Speci-
fied SQL Constructs.
Gwet, K. (2012). Handbook of inter-rater reliability: The
definitive guide to measuring the extent of agreement
among raters.
Hauser, F., Stark, T., Mottok, J., Gruber, H., and Reuter,
R. (2020). Deliberate Practice in Programming: How
is it carried out by programmers? In Proceedings of
the 4th European Conference on Software Engineer-
ing Education, ECSEE ’20, pages 42–46, New York,
NY, USA. Association for Computing Machinery.
ISO Central Secretary (2016). Information technology
– Database languages – SQL – Part 2: Founda-
tion (SQL/Foundation). Standard ISO/IEC 9075-
2:2016/Cor 2:2022, International Organization for
Standardization.
Iyer, S., Konstas, I., Cheung, A., and Zettlemoyer, L.
(2016). Summarizing Source Code using a Neural At-
tention Model. Proceedings of the 54th Annual Meet-
ing of the Association for Computational Linguistics
(Volume 1: Long Papers).
Kokkalis, A., Vagenas, P., Zervakis, A., Simitsis, A.,
Koutrika, G., and Ioannidis, Y. E. (2012). Logos: a
system for translating queries into narratives. Pro-
ceedings of the 2012 ACM SIGMOD International
Conference on Management of Data.
Kosh, A., Simpson, M. A., Bickel, L., Kellogg, M., and
Sanford-Moore, E. (2018). A Cost-Benefit Analysis
of Automatic Item Generation. Educational Measure-
ment: Issues and Practice, 38.
Koutrika, G., Simitsis, A., and Ioannidis, Y. E. (2010). Ex-
plaining structured queries in natural language. 2010
IEEE 26th International Conference on Data Engi-
neering (ICDE 2010), pages 333–344.
Krippendorff, K. (2004). Reliability in content analy-
sis: Some common misconceptions and recommenda-
tions. Human Communication Research, 30:411–433.
Lewis, M., Liu, Y., Goyal, N., Ghazvininejad, M., Mo-
hamed, A., Levy, O., Stoyanov, V., and Zettlemoyer,
L. (2019). Bart: Denoising sequence-to-sequence pre-
training for natural language generation, translation,
and comprehension.
Li, Y., Radev, D., and Rafiei, D. (2024). Natural Lan-
guage Interfaces to Databases. Synthesis Lectures on
Data Management. Springer International Publishing,
Cham.
Ma, D., Chen, X., Cao, R., Chen, Z., Chen, L., and Yu, K.
(2021). Relation-Aware Graph Transformer for SQL-
to-Text Generation. Applied Sciences.
Mandamadiotis, A., Koutrika, G., and Amer-Yahia, S.
(2024). Guided sql-based data exploration with user
feedback. In 2024 IEEE 40th International Confer-
ence on Data Engineering (ICDE), pages 4884–4896.
Oya, M. (2011). Syntactic dependency distance as sentence
complexity measure. Proceedings of the 16th Inter-
national Conference of Pan-Pacific Association of Ap-
plied Linguistics.
Raffel, C., Shazeer, N., Roberts, A., Lee, K., Narang, S.,
Matena, M., Zhou, Y., Li, W., and Liu, P. J. (2023).
Exploring the limits of transfer learning with a unified
text-to-text transformer.
Raslan, G. The Impact of the Zone of Proximal Develop-
ment Concept (Scaffolding) on the Students Problem
Solving Skills and Learning Outcomes. In BUiD Doc-
toral Research Conference 2023, pages 59–66, Cham.
Springer Nature Switzerland.
Reimers, N. and Gurevych, I. (2019). Sentence-bert: Sen-
tence embeddings using siamese bert-networks.
Stephen Cass (2024). Top Programming Languages 2024.
van der Lee, C., Gatt, A., van Miltenburg, E., and Krah-
mer, E. J. (2021). Human evaluation of automatically
generated text: Current trends and best practice guide-
lines. Comput. Speech Lang., 67:101151.
Westacott, R., Badger, K., Kluth, D., Gurnell, M., Reed, M.
W. R., and Sam, A. H. (2023). Automated Item Gen-
eration: impact of item variants on performance and
standard setting. BMC Medical Education, 23(1):659.
Xu, K., Wu, L., Wang, Z., Yu, M., Chen, L., and Sheinin,
V. (2018). SQL-to-Text Generation with Graph-to-
Sequence Model. In Conference on Empirical Meth-
ods in Natural Language Processing.
AIG 2025 - Special Session on Automatic Item Generation
796