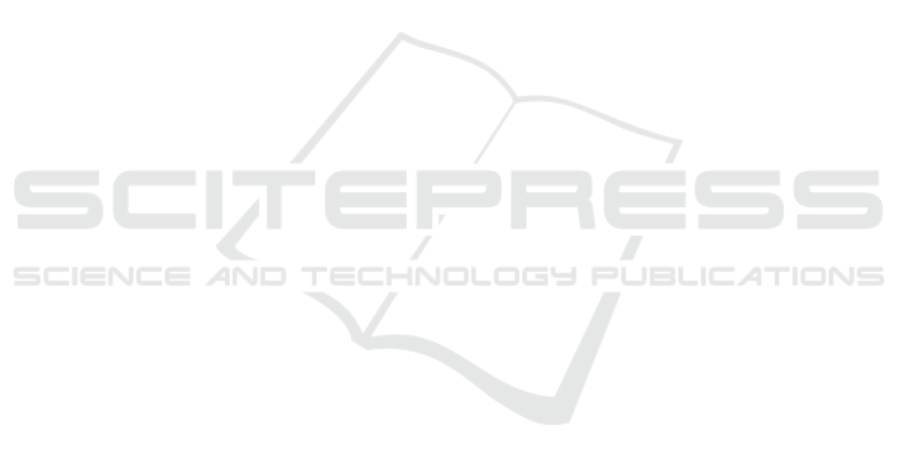
a period of at least 100 ms show a velocity lower than
the specified maximum velocity, this was recognized
by the evaluation program as a fixation. During this
process, all fixation durations as well as the number of
fixations were saved. In addition, the eye-hit positions
at the beginning and end of a fixation were determined
and the theoretical transition distances calculated.
5 RESULTS
The sample consists of N
′
= 121 test subjects, all of
whom are students for a Bachelor’s (89.8%) or Mas-
ter’s degree (10.2%) in a variety of subjects, most
of which (86.4%) related to teaching studies. 49.2%
mentioned at least one of their usually two subjects
being in the STEM realm. About 56% are female and
44% male with a mean age of 22.6 (SD = 2.9). The
sample has low prior knowledge about MR, which
was rated at a mean of 1.60 (SD = 0.98) on a five-
point Likert-scale (1 = very low to 5 = very high).
However, a slightly increased interest in MR with a
mean of 3.64 (SD = 1.07) and increased affinity to
digital technologies with a mean of 3.85 (SD = 1.04),
on the same scale as above, is reported by the test sub-
jects.
Each of the test persons completed three tasks,
both with analog and with virtual dice. Thus, a to-
tal of N = 363 data sets could be examined. In Ta-
ble 1, the results of a Spearman correlation analysis
between eye-tracking parameters and self-rated cog-
nitive load types are shown for the aggregated data
(N = 363). The table contains the correlation co-
efficient r and the p-value for significance (2-sided)
for each of the eye-tracking parameters examined and
columnwise listed for total cognitive load (CL) and its
three subcategories (GCL, ICL, ECL) .
It is noted that the fixation rate (FR) is the only
eye-tracking measure investigated, which correlates
with both the total CL and all three subcategories.
The correlation is low-ranked (0.1 ≤ r < 0.3) posi-
tive, highly significant (p < 0.001, denoted by
∗∗∗
)
in all four cases. The other two measures showing
highly significant correlations with CL, GCL and ICL
are percentage of fixations (PF) and general transition
velocity (GTV), where PF ranks in the moderate cor-
relation range (0.3 ≤ r < 0.5) for GCL and ICL and
the correlation with CL is relatively close (r = 0.292).
Besides FR, ECL only correlates highly significant
with MFD, negatively and low-ranked (0.1 ≤ r < 0.3).
Another very significant (p < 0.01, denoted by
∗∗
)
correlation of ECL is found for GTV, however, posi-
tive and low-ranked. In addition, MFD correlates low
negative, significant (p < 0.05, denoted by
∗
) with
CL, very significant with ICL and highly significant
with ECL. Finally, TR correlates negatively with GCL
and ICL but in the low range.
Since data is aggregated for three taks with dif-
ferent level of difficulty here, a task-wise analysis
of correlations is also conducted. Significant corre-
lations are found much less frequent as for the ag-
gregated data set, as shown in Table 2. Only PF
shows highly significant correlations with CL, GCL
and ICL, which are in the moderate range, but only
for task 1. The other correlations for task 1 and 2 are
merely significant with a low-range correlation coef-
ficient including MFD-ECL, PF-GCL, PF-ICL, FR-
ECL, GTV-CL, GTV-GCL and GTV-ICL. The iso-
lated data of task 3 does, however, not show any cor-
relation with CL or its subtypes.
6 DISCUSSION
The hypothesis that the specific eye-tracking parame-
ters chosen for this work correlate with cognitive load
is supported by the results. The fixation rate (FR)
seems to be particularly promising in terms of signif-
icance, while percentage of fixations (PF) shows high
correlation coefficients. However, to distinguish be-
tween different subcategories of cognitive load (CL),
additional parameters such as mean fixation duration
(MFD) or transition ratio (TR) might be needed.
It is important to note that the identified correla-
tions for extraneous cognitive load (ECL) should be
taken with caution, as the data are heavily concen-
trated at levels 1 to 3 in the underlying data. As this
scale does not even cover half of the possible seven-
level rating scale (see Figure 3), the correlations de-
termined for ECL only apply to low to medium ECL.
Similarly, it should be noted that little data is available
for the high range of CL (levels 8 and 9) and the high
range of germane cognitive load (GCL) and intrinsic
cognitive load (ICL) (level 7), see Figure 3.
In general, the data are not sufficiently distributed
across the entire spectrum of the respective cognitive
load. This could be explained by the fact that except
for the virtual dice and the menu interface, there are
no other virtual objects that would add ECL. Also, the
physical test setup is kept simple and clear (see Fig-
ure 1). It follows that the obtained correlations are not
generally applicable without further ado, and further
research is needed to verify generalizability. Further-
more, analysis of the eye-tracking data showed large
variations in the respective parameters between indi-
viduals, resulting in large standard deviations with re-
spect to the respective CL. Nevertheless, the analysis
software was able to extract eye-tracking parameters
Relationship Between Eye-Tracking Metrics and Cognitive Load in Mixed Reality
903