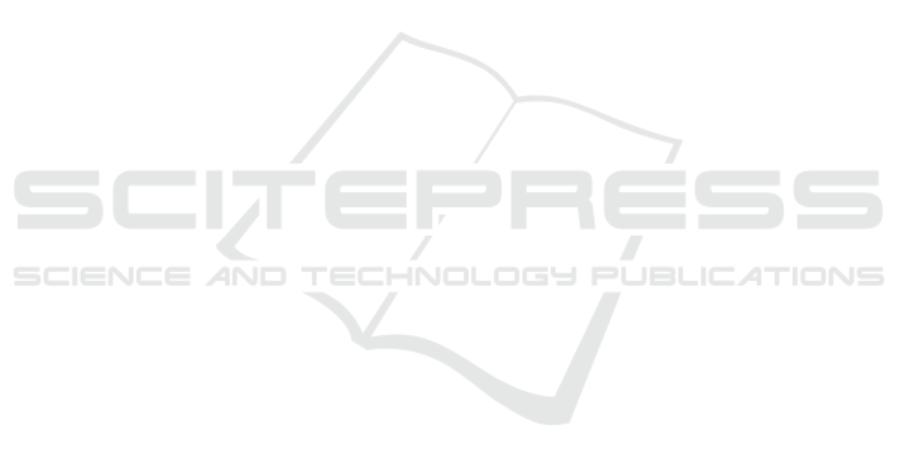
6 FUNDING STATEMENT
This research was fully funded by the Interdisci-
plinary Research Center for Intelligent Secure Sys-
tems (IRC-ISS) through the Deanship of Research,
King Fahd University of Petroleum and Minerals,
Dhahran 31261, Saudi Arabia. The IRC-ISS research
grant #INSS2516 supported the required data and
computing resources for this experiment.
7 DATA AND CODE
AVAILABILITY
Both datasets used in this study are publicly available
on Kaggle, provided in (Xing et al., 2023), (Kanda-
gatla, 2022). The code for this study is available from
the authors upon reasonable request.
REFERENCES
Ageing and (AAH), H. (2008). Who global report on
falls prevention in older age. https://www.who.int/
publications/i/item/9789241563536. Accessed: Mar.
03, 2024.
Alam, E., Sufian, A., Dutta, P., and Leo, M. (2022). Vision-
based human fall detection systems using deep learn-
ing: A review. Computers in biology and medicine,
146:105626.
Alanazi, T. and Muhammad, G. (2022). Human fall detec-
tion using 3d multi-stream convolutional neural net-
works with fusion. Diagnostics, 12(12).
Bo, L. (2023). Human fall detection for smart home car-
ing using yolo networks. International Journal of Ad-
vanced Computer Science and Applications, 14(4).
Chen, Z., Wang, Y., and Yang, W. (2022). Video based fall
detection using human poses. In CCF Conference on
Big Data, pages 283–296. Springer.
Chollet, F. (2017). Xception: Deep learning with depthwise
separable convolutions. In Proceedings of the IEEE
Conference on Computer Vision and Pattern Recogni-
tion (CVPR), pages 1251–1258.
Gomes, M. E. N., Mac
ˆ
edo, D., Zanchettin, C., de Mattos-
Neto, P. S. G., and Oliveira, A. (2022). Multi-human
fall detection and localization in videos. Computer
Vision and Image Understanding, 220.
Gunale, K. G., Mukherji, P., and Motade, S. N. (2023). Con-
volutional neural network-based fall detection for the
elderly person monitoring. Journal of Advances in In-
formation Technology, 14(6):1169–1176.
He, K., Zhang, X., Ren, S., and Sun, J. (2016). Deep resid-
ual learning for image recognition. In Proceedings of
the IEEE Conference on Computer Vision and Pattern
Recognition (CVPR), pages 770–778.
Hoang, V. H., Lee, J. W., Piran, M. J., and Park, C. S.
(2023). Advances in skeleton-based fall detection in
rgb videos: From handcrafted to deep learning ap-
proaches. IEEE Access, 11:92322–92352.
Howard, A. G., Zhu, M., Chen, B., Kalenichenko, D.,
Wang, W., Weyand, T., Andreetto, M., and Adam,
H. (2017). Mobilenets: Efficient convolutional neu-
ral networks for mobile vision applications. arXiv
preprint arXiv:1704.04861.
Huang, G., Liu, Z., Maaten, L. V. D., and Weinberger, K. Q.
(2017). Densely connected convolutional networks.
In Proceedings of the IEEE Conference on Computer
Vision and Pattern Recognition (CVPR), pages 4700–
4708.
Kan, X., Zhu, S., Zhang, Y., and Qian, C. (2023). A
lightweight human fall detection network. Sensors,
23(22).
Kandagatla, U. K. (2022). Fall detection dataset. https:
//www.kaggle.com/datasets/uttejkumarkandagatla/
fall-detection-dataset. Accessed: Dec. 20, 2024.
Lian, J., Yuan, X., Li, M., and Tzeng, N. F. (2021). Fall
detection via inaudible acoustic sensing. Proc ACM
Interact Mob Wearable Ubiquitous Technol, 5(3).
MakeSense.AI (2024). Makesense.ai - free annotation tool
for ai and machine learning. https://www.makesense.
ai/. Accessed: 2024-12-20.
N
´
u
˜
nez-Marcos, A. and Arganda-Carreras, I. (2024).
Transformer-based fall detection in videos. En-
gineering Applications of Artificial Intelligence,
132:107937.
Simonyan, K. and Zisserman, A. (2015). Very deep con-
volutional networks for large-scale image recogni-
tion. International Conference on Learning Represen-
tations (ICLR).
Szegedy, C., Vanhoucke, V., Ioffe, S., Shlens, J., and Wo-
jna, Z. (2016). Rethinking the inception architecture
for computer vision. In Proceedings of the IEEE Con-
ference on Computer Vision and Pattern Recognition
(CVPR), pages 2818–2826.
Xing, Z., Chaturvedi, K., Braytee, A., Li, J., and
Prasad, M. (2023). Fe2i fall detection dataset
(annotated). https://www.kaggle.com/datasets/starxz/
fe2i-fall-detection-datasetannotated. Accessed: Dec.
21, 2024.
Zi, X., Chaturvedi, K., Braytee, A., Li, J., and Prasad, M.
(2023). Detecting human falls in poor lighting: Ob-
ject detection and tracking approach for indoor safety.
Electronics (Switzerland), 12(5).
IS4WB_SC 2025 - Special Session on Innovative Strategies to Enhance Older Adults’ Well-being and Social Connections
420